Multi-Turn RNN-T for Streaming Recognition of Multi-Party Speech.
IEEE International Conference on Acoustics, Speech, and Signal Processing (ICASSP)(2022)
摘要
Automatic speech recognition (ASR) of single channel far-field recordings with an unknown number of speakers is traditionally tackled by cascaded modules. Recent research shows that end-to-end (E2E) multi-speaker ASR models can achieve superior recognition accuracy compared to modular systems. However, these models do not ensure real-time applicability due to their dependency on full audio context. This work takes real-time applicability as the first priority in model design and addresses a few challenges in previous work on multi-speaker recurrent neural network transducer (MS-RNN-T). First, we introduce on-the-fly overlapping speech simulation during training, yielding 14% relative word error rate (WER) improvement on LibriSpeechMix test set. Second, we propose a novel multi-turn RNN-T (MT-RNN-T) model with an overlap-based target arrangement strategy that generalizes to an arbitrary number of speakers without changes in the model architecture. We investigate the impact of the maximum number of speakers seen during training on MT-RNN-T performance on LibriCSS test set, and report 28% relative WER improvement over the two-speaker MS-RNN-T. Third, we experiment with a rich transcription strategy for joint recognition and segmentation of multi-party speech. Through an in-depth analysis, we discuss potential pitfalls of the proposed system as well as promising future research directions.
更多查看译文
关键词
streaming multi-speaker speech recognition,overlapped speech,recurrent neural network transducer,multi-turn
AI 理解论文
溯源树
样例
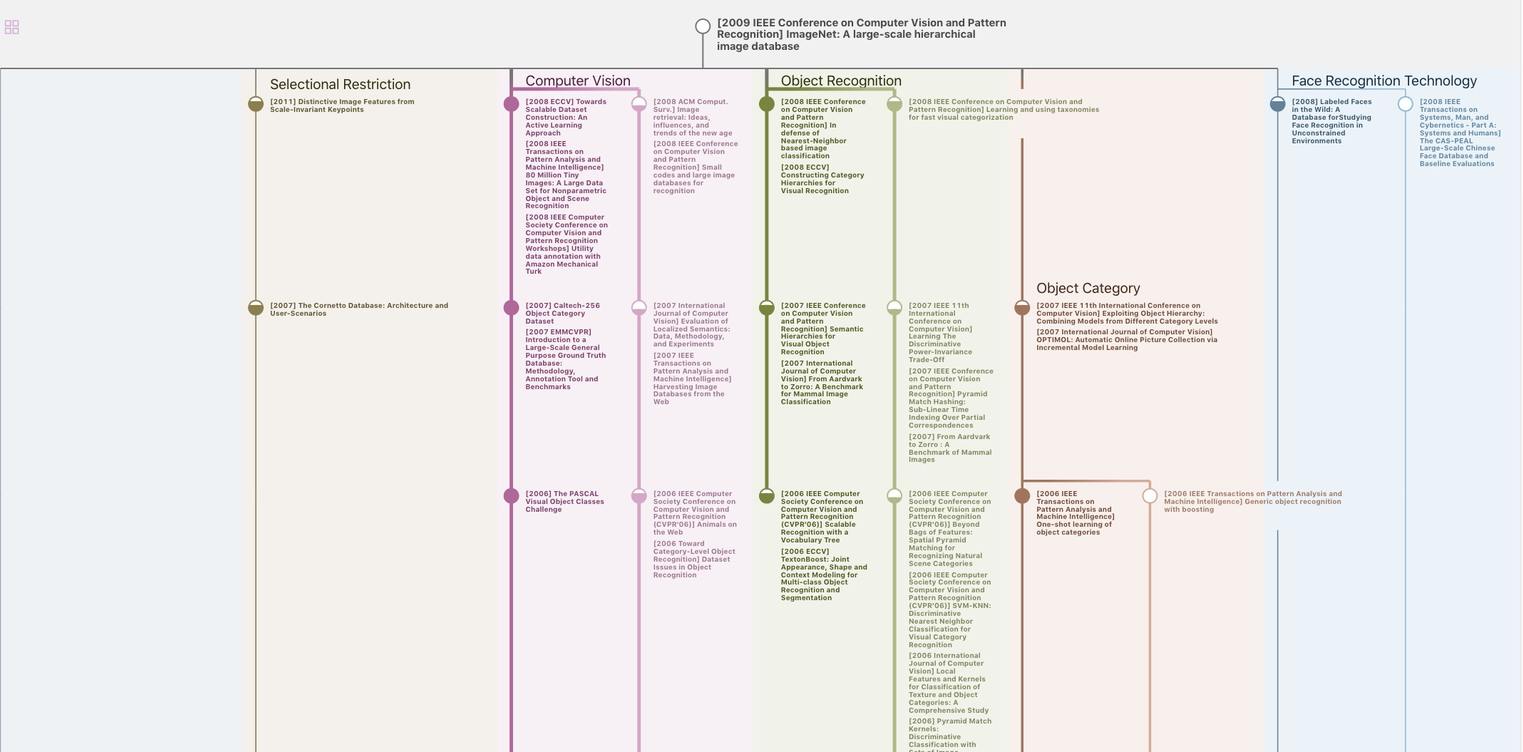
生成溯源树,研究论文发展脉络
Chat Paper
正在生成论文摘要