On efficient transformer-based image pre-training for low-level vision
arxiv(2023)
摘要
Pre-training has marked numerous state of the arts in high-level computer vision, while few attempts have ever been made to investigate how pretraining acts in image processing systems. In this paper, we tailor transformer-based pre-training regimes that boost various low-level tasks. To comprehensively diagnose the influence of pre-training, we design a whole set of principled evaluation tools that uncover its effects on internal representations. The observations demonstrate that pretraining plays strikingly different roles in low-level tasks. For example, pre-training introduces more local information to intermediate layers in super-resolution (SR), yielding significant performance gains, while pre-training hardly affects internal feature representations in denoising, resulting in limited gains. Further, we explore different methods of pre-training, revealing that multi-related-task pretraining is more effective and data-efficient than other alternatives. Finally, we extend our study to varying data scales and model sizes, as well as comparisons between transformers and CNNs. Based on the study, we successfully develop state-of-the-art models for multiple low-level tasks.
更多查看译文
关键词
vision,transformer-based,pre-training,low-level
AI 理解论文
溯源树
样例
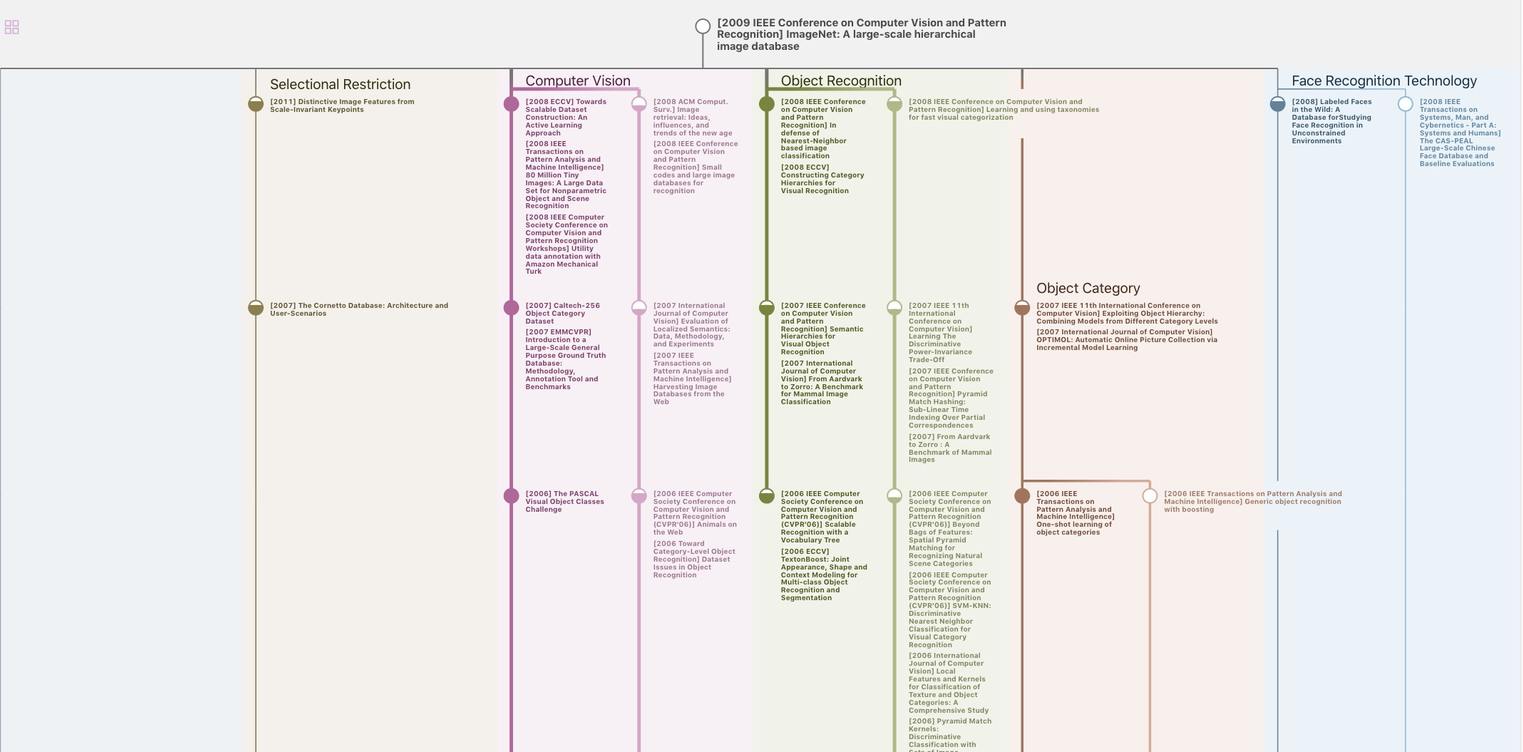
生成溯源树,研究论文发展脉络
Chat Paper
正在生成论文摘要