Bi-exponential modelling of $$W^{^{\prime}}$$ W ′ reconstitution kinetics in trained cyclists
EUROPEAN JOURNAL OF APPLIED PHYSIOLOGY(2021)
摘要
The aim of this study was to investigate the individual
$$W^{^{\prime}}$$
reconstitution kinetics of trained cyclists following repeated bouts of incremental ramp exercise, and to determine an optimal mathematical model to describe
$$W^{^{\prime}}$$
reconstitution. Ten trained cyclists (age 41 ± 10 years; mass 73.4 ± 9.9 kg;
$$\dot{V}{\text{O}}_{2\max }$$
58.6 ± 7.1 mL kg min−1) completed three incremental ramps (20 W min−1) to the limit of tolerance with varying recovery durations (15–360 s) on 5–9 occasions.
$$W^{^{\prime}}$$
reconstitution was measured following the first and second recovery periods against which mono-exponential and bi-exponential models were compared with adjusted R2 and bias-corrected Akaike information criterion (AICc). A bi-exponential model outperformed the mono-exponential model of
$$W^{^{\prime}}$$
reconstitution (AICc 30.2 versus 72.2), fitting group mean data well (adjR2 = 0.999) for the first recovery when optimised with parameters of fast component (FC) amplitude = 50.67%; slow component (SC) amplitude = 49.33%; time constant (τ)FC = 21.5 s; τSC = 388 s. Following the second recovery, W′ reconstitution reduced by 9.1 ± 7.3%, at 180 s and 8.2 ± 9.8% at 240 s resulting in an increase in the modelled τSC to 716 s with τFC unchanged. Individual bi-exponential models also fit well (adjR2 = 0.978 ± 0.017) with large individual parameter variations (FC amplitude 47.7 ± 17.8%; first recovery: (τ)FC = 22.0 ± 11.8 s; (τ)SC = 377 ± 100 s; second recovery: (τ)FC = 16.3.0 ± 6.6 s; (τ)SC = 549 ± 226 s). W′ reconstitution kinetics were best described by a bi-exponential model consisting of distinct fast and slow phases. The amplitudes of the FC and SC remained unchanged with repeated bouts, with a slowing of W′ reconstitution confined to an increase in the time constant of the slow component.
更多查看译文
关键词
Critical power,Recovery,Modelling,Fatigue
AI 理解论文
溯源树
样例
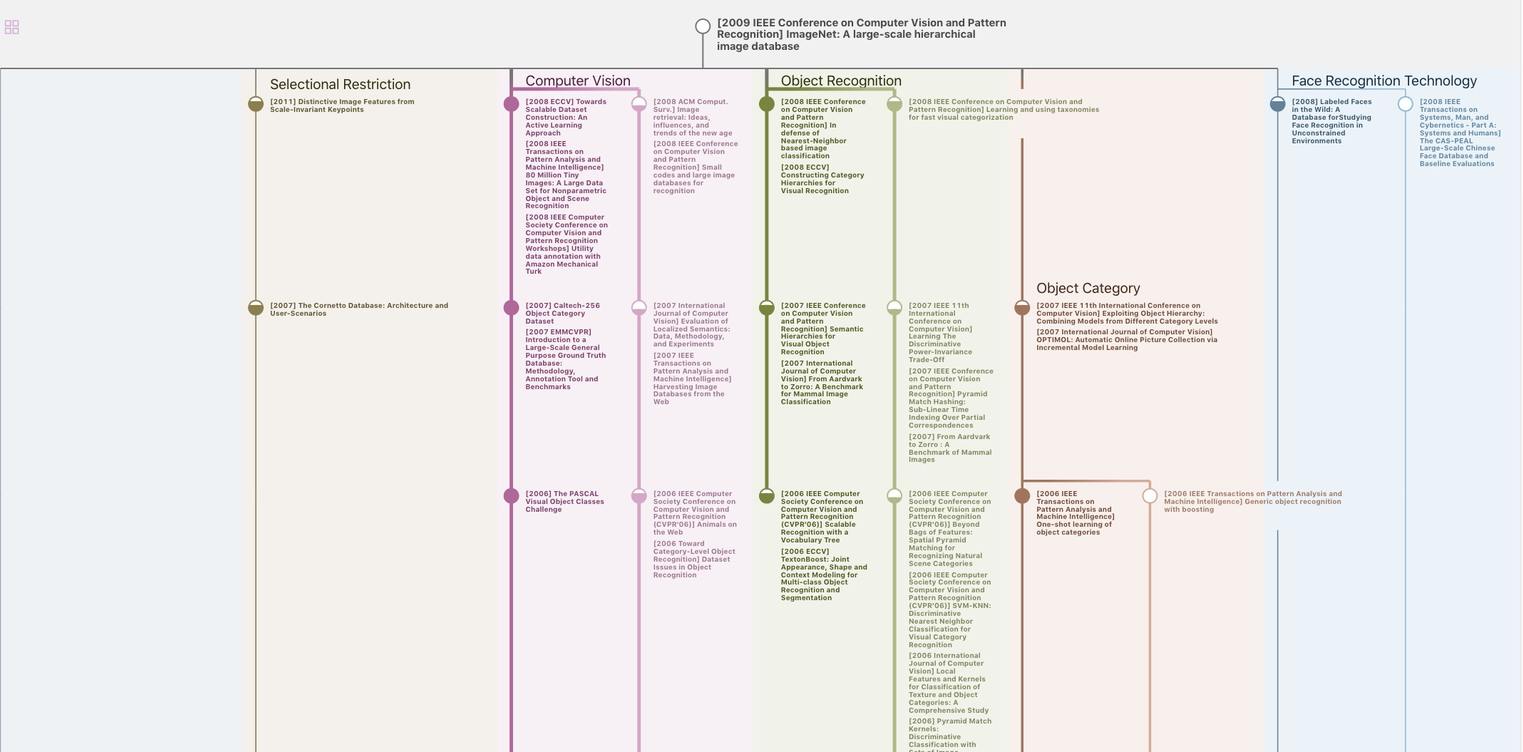
生成溯源树,研究论文发展脉络
Chat Paper
正在生成论文摘要