Machine learning models on chemical inhibitors of mitochondrial electron transport chain
Journal of Hazardous Materials(2022)
摘要
Chemicals can induce adverse effects in humans by inhibiting mitochondrial electron transport chain (ETC) such as disrupting mitochondrial membrane potential, enhancing oxidative stress and causing some diseases. Thus, identifying ETC inhibitors (ETCi) is important to chemical risk assessment and protecting the public health. However, it is not feasible to identify all ETCi with experimental methods. Quantitative structure-activity relationship (QSAR) modeling is a promising method to rapidly and effectively identify ETCi. In this study, QSAR models for predicting ETCi were developed using machine learning methods. A clustering-based under-sampling (CBUS) method was developed to handle the imbalance issue in training sets. Structure-activity landscapes were generated and analyzed for training sets generated by the CBUS method. The consensus QSAR models constructed with CBUS achieved satisfactory performances (balanced accuracy = 0.852) in 100 iterations of five-fold cross validations, indicating the models can effectively classify ETCi. The classification model was further employed to screen chemicals in the Inventory of Existing Chemical Substances of China and 13 chemicals were identified as ETCi. Fifteen structural alerts for ETCi were identified in this study. These results demonstrated that the model and structural alerts are useful to screen ETCi.
更多查看译文
关键词
Mitochondrial electron transport chain,Machine learning,QSAR,Structural alerts
AI 理解论文
溯源树
样例
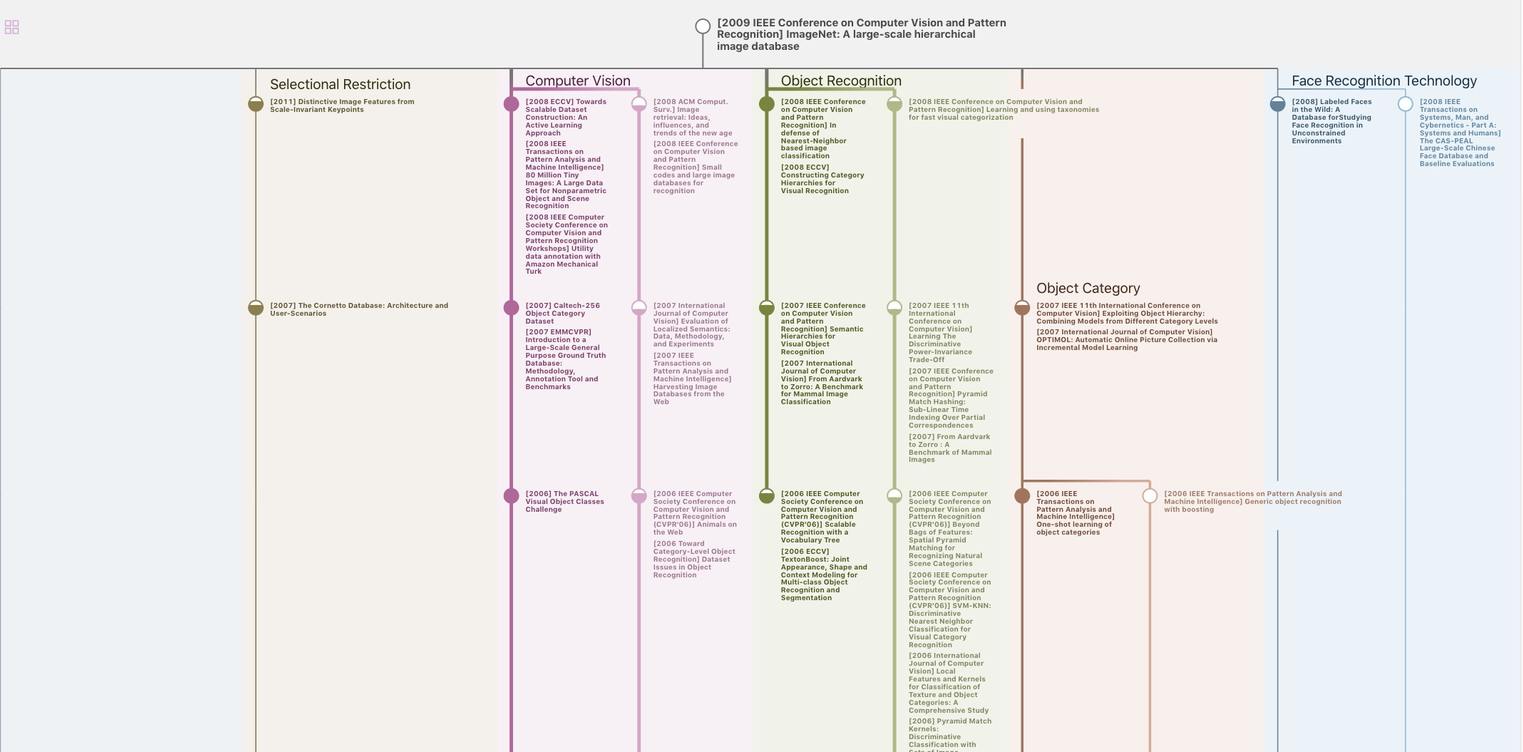
生成溯源树,研究论文发展脉络
Chat Paper
正在生成论文摘要