Adaptive regularization for nonconvex optimization using inexact function values and randomly perturbed derivatives
Journal of Complexity(2022)
摘要
A regularization algorithm allowing random noise in derivatives and inexact function values is proposed for computing approximate local critical points of any order for smooth unconstrained optimization problems. For an objective function with Lipschitz continuous p-th derivative and given an arbitrary optimality order q≤p, an upper bound on the number of function and derivatives evaluations is established for this algorithm. This bound is in expectation, and in terms of a power of the required tolerances, this power depending on whether q≤2 or q>2. Moreover these bounds are sharp in the order of the accuracy tolerances. An extension to convexly constrained problems is also outlined.
更多查看译文
关键词
Evaluation complexity,Regularization methods,Inexact functions and derivatives,Stochastic analysis
AI 理解论文
溯源树
样例
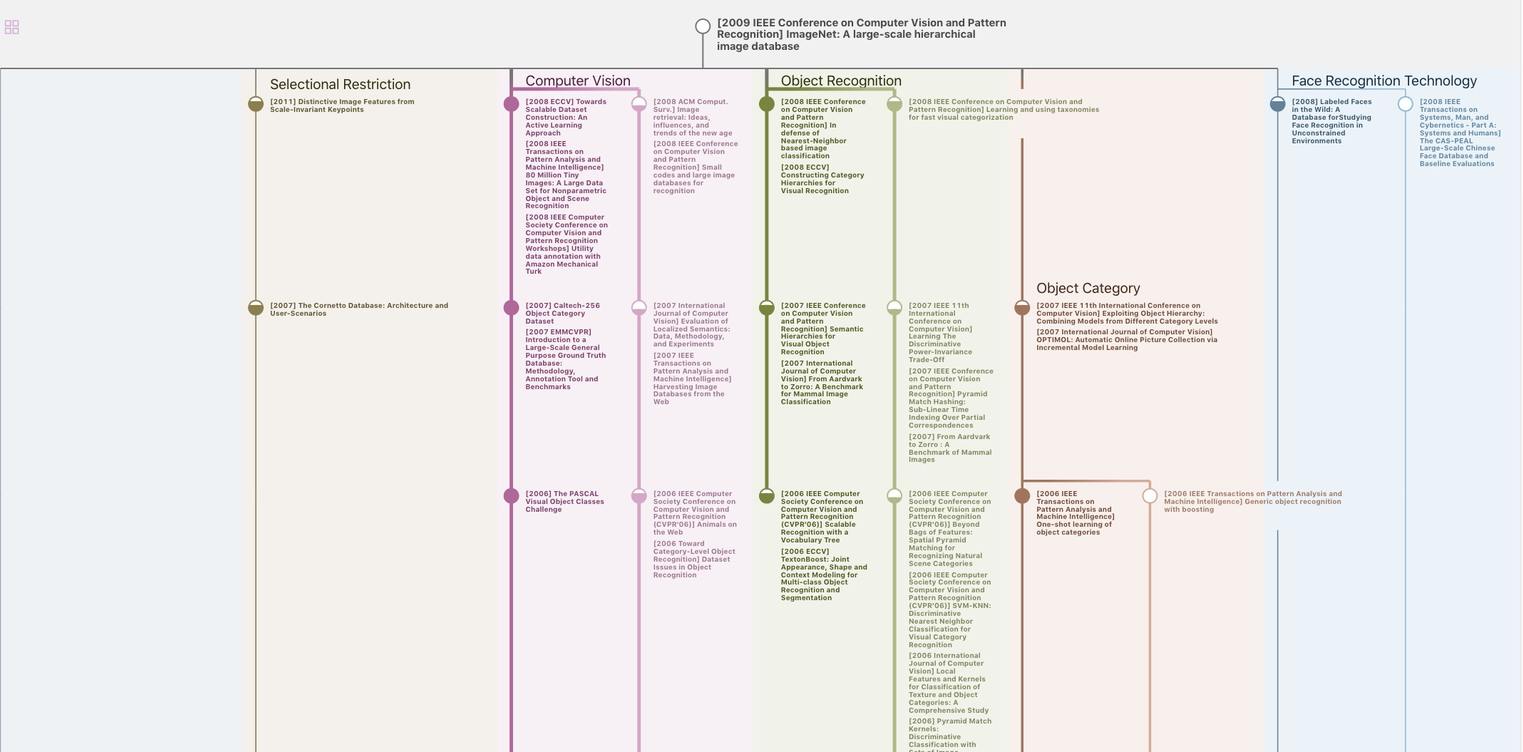
生成溯源树,研究论文发展脉络
Chat Paper
正在生成论文摘要