RegionCLIP: Region-based Language-Image Pretraining
IEEE Conference on Computer Vision and Pattern Recognition(2022)
摘要
Contrastive language-image pretraining (CLIP) using image-text pairs has achieved impressive results on image classification in both zero-shot and transfer learning set-tings. However, we show that directly applying such mod-els to recognize image regions for object detection leads to unsatisfactory performance due to a major domain shift: CLIP was trained to match an image as a whole to a text de-scription, without capturing the fine-grained alignment be-tween image regions and text spans. To mitigate this issue, we propose a new method called RegionCLIP that signifi-cantly extends CLIP to learn region-level visual representations, thus enabling fine-grained alignment between image regions and textual concepts. Our method leverages a CLIP model to match image regions with template captions, and then pretrains our model to align these region-text pairs in the feature space. When transferring our pretrained model to the open-vocabulary object detection task, our method outperforms the state of the art by 3.8 AP50 and 2.2 AP for novel categories on COCO and LVIS datasets, respectively. Further, the learned region representations support zero-shot inference for object detection, showing promising results on both COCO and LVIS datasets. Our code is available at https://github.com/microsoft/RegionCLIP.
更多查看译文
关键词
Representation learning, Recognition: detection,categorization,retrieval, Self-& semi-& meta- Transfer/low-shot/long-tail learning, Vision + language
AI 理解论文
溯源树
样例
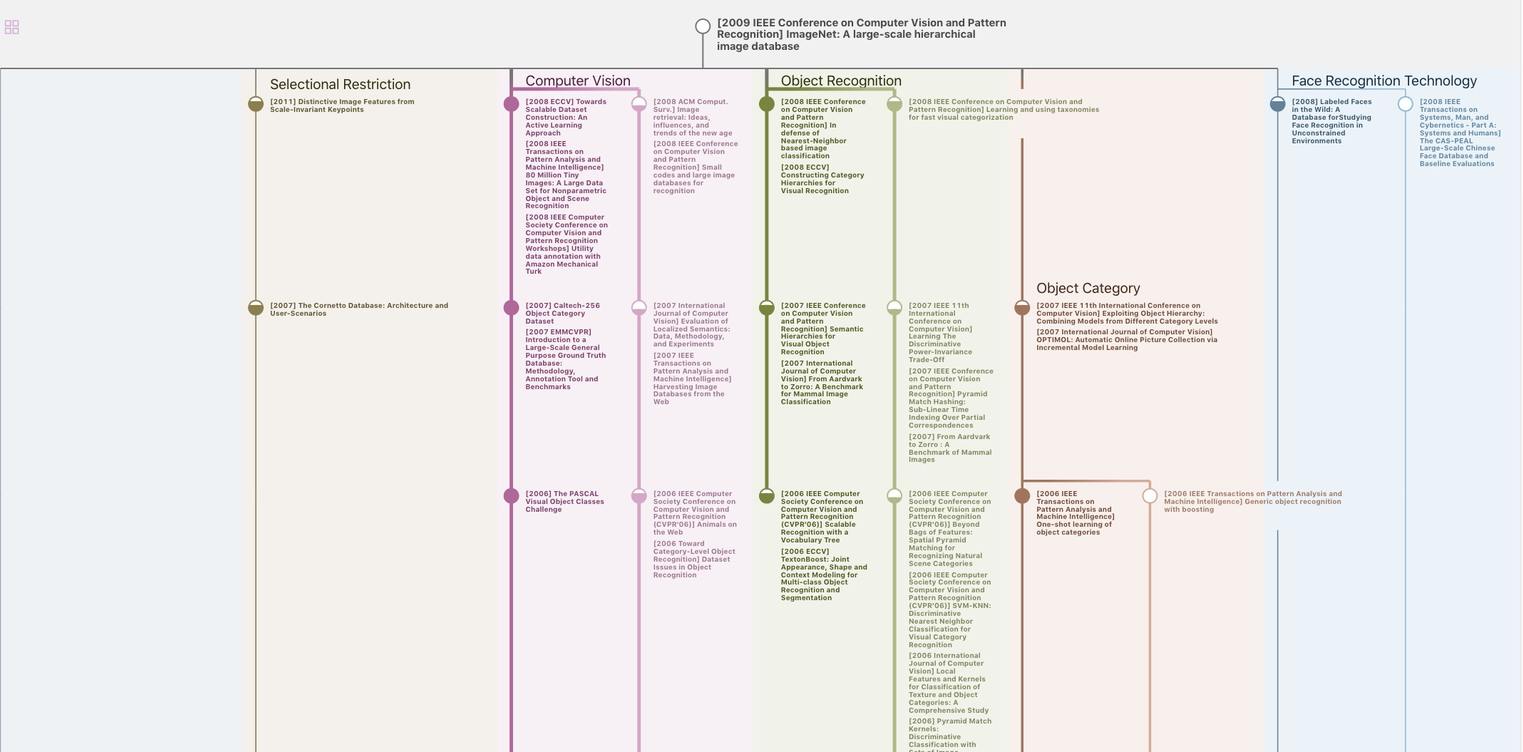
生成溯源树,研究论文发展脉络
Chat Paper
正在生成论文摘要