MVSS-Net: Multi-View Multi-Scale Supervised Networks for Image Manipulation Detection
IEEE Transactions on Pattern Analysis and Machine Intelligence(2023)
摘要
As manipulating images by copy-move, splicing and/or inpainting may lead to misinterpretation of the visual content, detecting these sorts of manipulations is crucial for media forensics. Given the variety of possible attacks on the content, devising a generic method is nontrivial. Current deep learning based methods are promising when training and test data are well aligned, but perform poorly on independent tests. Moreover, due to the absence of authentic test images, their image-level detection specificity is in doubt. The key question is how to design and train a deep neural network capable of learning generalizable features
sensitive
to manipulations in novel data, whilst
specific
to prevent false alarms on the authentic. We propose multi-view feature learning to jointly exploit tampering boundary artifacts and the noise view of the input image. As both clues are meant to be semantic-agnostic, the learned features are thus generalizable. For effectively learning from authentic images, we train with multi-scale (pixel / edge / image) supervision. We term the new network MVSS-Net and its enhanced version MVSS-Net++. Experiments are conducted in both within-dataset and cross-dataset scenarios, showing that MVSS-Net++ performs the best, and exhibits better robustness against JPEG compression, Gaussian blur and screenshot based image re-capturing.
更多查看译文
关键词
Image manipulation detection,multi-view feature learning,multi-scale supervision,model sensitivity and specificity
AI 理解论文
溯源树
样例
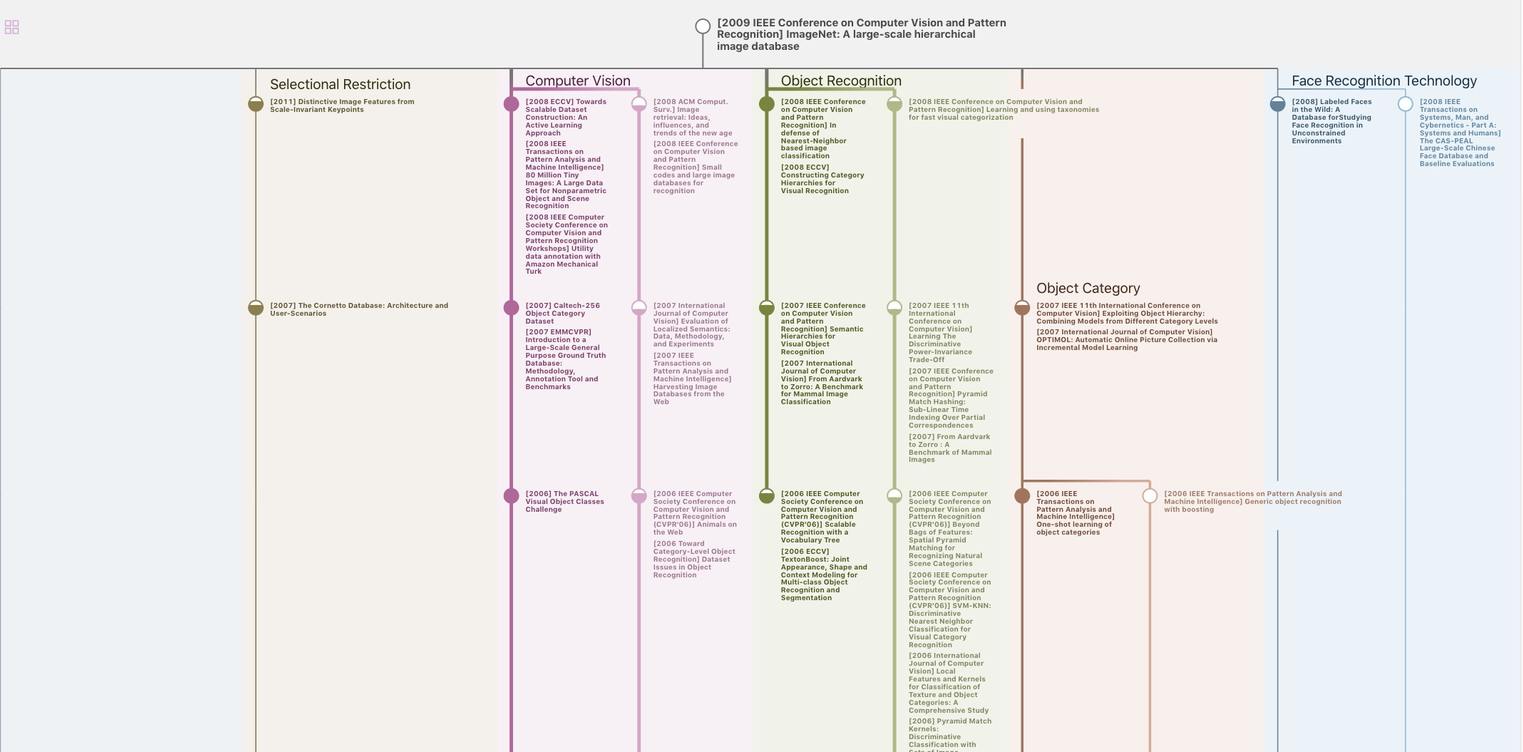
生成溯源树,研究论文发展脉络
Chat Paper
正在生成论文摘要