SGEITL: Scene Graph Enhanced Image-Text Learning for Visual Commonsense Reasoning.
AAAI Conference on Artificial Intelligence(2022)
摘要
Answering complex questions about images is an ambitious goal for machine intelligence, which requires a joint understanding of images, text, and commonsense knowledge, as well as a strong reasoning ability. Recently, multimodal Transformers have made a great progress in the task of Visual Commonsense Reasoning (VCR), by jointly understanding visual objects and text tokens through layers of cross-modality attention. However, these approaches do not utilize the rich structure of the scene and the interactions between objects which are essential in answering complex commonsense questions. We propose aScene Graph Enhanced Image-Text Learning (SGEITL) framework to incorporate visual scene graph in commonsense reasoning. In order to exploit the scene graph structure, at the model structure level, we propose a multihop graph transformer for regularizing attention interaction among hops. As for pre-training, a scene-graph-aware pre-training method is proposed to leverage structure knowledge extracted in visual scene graph. Moreover, we introduce a method to train and generate domain relevant visual scene graph using textual annotations in a weakly-supervised manner. Extensive experiments on VCR and other tasks show significant performance boost compared with the state-of-the-art methods, and prove the efficacy of each proposed component.
更多查看译文
关键词
Knowledge Representation And Reasoning (KRR),Computer Vision (CV),Machine Learning (ML),Cognitive Modeling & Cognitive Systems (CMS)
AI 理解论文
溯源树
样例
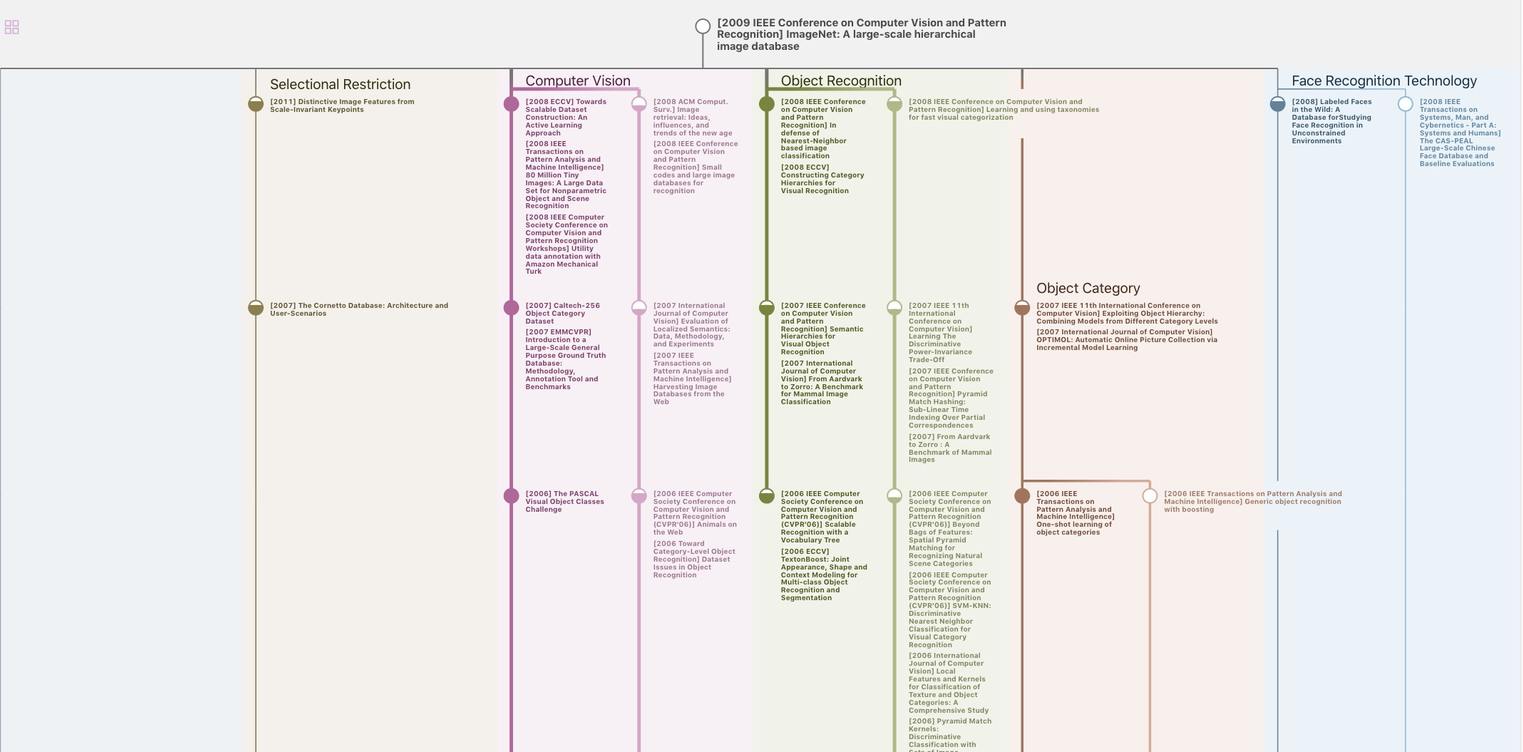
生成溯源树,研究论文发展脉络
Chat Paper
正在生成论文摘要