CPSSDS: Conformal prediction for semi-supervised classification on data streams
Information Sciences(2022)
摘要
In this study, we focus on semi-supervised data stream classification tasks. With the advent of applications that generate vast streams of data, data stream mining algorithms are required to handle the intrinsic issues of learning patterns from this type of non-stationary data. The main challenges are fast arriving, infinite length, limited labeled data, concept drift, and concept evolution. Although many algorithms have been used to overcome these issues, there are still many challenges that need to be addressed and discussed in practical domains. This paper mainly focuses on the limited labeled data and concept drift detection issues. Therefore, a semi-supervised framework called CPSSDS is proposed, which uses an incremental classifier as a base learner and a self-training framework to handle the scarcity of labeled samples. The proposed approach utilizes a form of Conformal Predictors to discover a set of informative unlabeled data instances to add to the original training set in each training procedure which is the main challenge in the standard self-training approach. In terms of this framework, the Kolmogorov-Smirnov test is adopted to detect concept drift by comparing the outputs of conformal prediction for two sequences of data chunks. Finally, experimental results on several synthetic and real datasets demonstrate that the proposed learning framework is comparable to the state-of-the-art semi-supervised algorithms for data streams. The implementation source code of the CPSSDS is available at https://github.com/neginsmd/CPSSDS.git.
更多查看译文
关键词
Data streams,Self-training,Semi-supervised learning,Concept drift,Conformal prediction
AI 理解论文
溯源树
样例
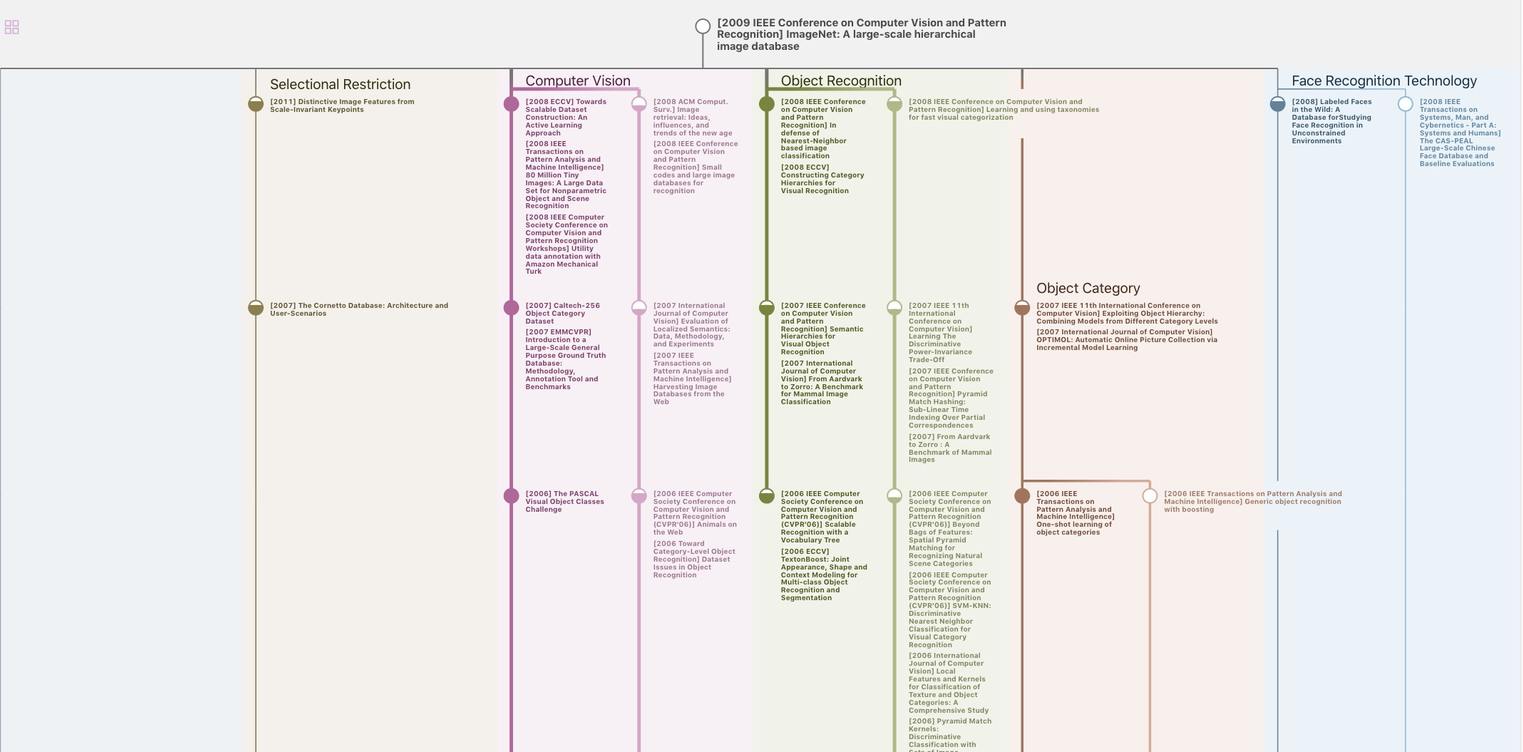
生成溯源树,研究论文发展脉络
Chat Paper
正在生成论文摘要