DeepEdge: A New QoE-Based Resource Allocation Framework Using Deep Reinforcement Learning for Future Heterogeneous Edge-IoT Applications
IEEE Transactions on Network and Service Management(2021)
摘要
Edge computing is emerging to empower the future of Internet of Things (IoT) applications. However, due to heterogeneity of applications, it is a significant challenge for the edge cloud to effectively allocate multidimensional limited resources (CPU, memory, storage, bandwidth, etc.) with constraints of applications’ Quality of Service (QoS) requirements. In this paper, we address the resource allocation problem in Edge-IoT systems through developing a novel framework named
DeepEdge
that allocates resources to the heterogeneous IoT applications with the goal of maximizing users’ Quality of Experience (QoE). To achieve this goal, we develop a novel QoE model that considers aligning the heterogeneous requirements of IoT applications to the available edge resources. The alignment is achieved through selection of QoS requirement range that can be satisfied by the available resources. In addition, we propose a novel two-stage deep reinforcement learning (DRL) scheme that effectively allocates edge resources to serve the IoT applications and maximize the users’ QoE. Unlike the typical DRL, our scheme exploits deep neural networks (DNN) to improve actions’ exploration by using DNN to map the Edge-IoT state to joint resource allocation action that consists of resource allocation and QoS class. The joint action not only maximize users’ QoE and satisfies heterogeneous applications’ requirements but also align the QoS requirements to the available resources. In addition, we develop a Q-value approximation approach to tackle the large space problem of Edge-IoT. Further evaluation shows that
DeepEdge
brings considerable improvements in terms of QoE, latency and application tasks’ success ratio in comparison to the existing resource allocation schemes.
更多查看译文
关键词
Resource allocation,deepEdge,edge-IoT,deep reinforcement learning (DRL),quality of experience (QoE)
AI 理解论文
溯源树
样例
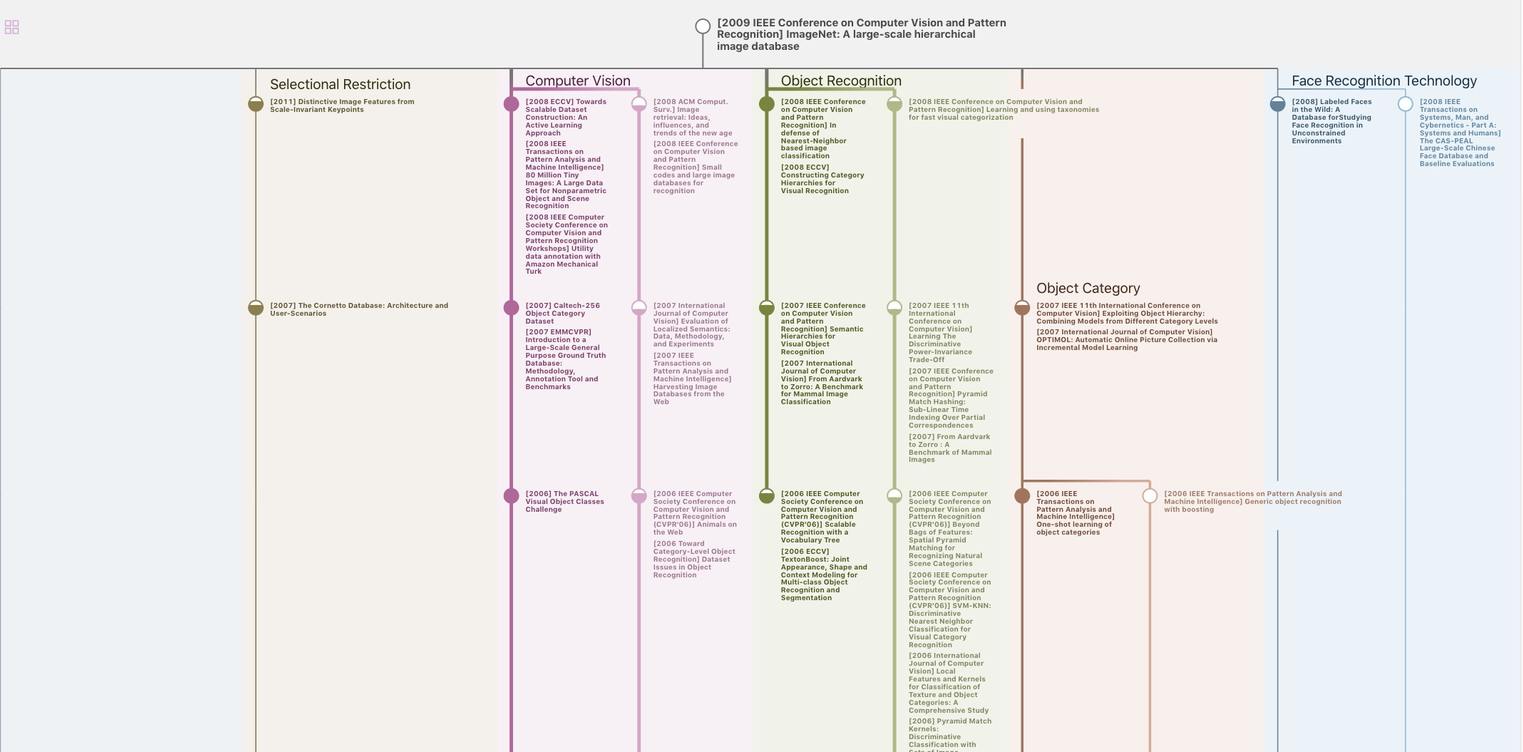
生成溯源树,研究论文发展脉络
Chat Paper
正在生成论文摘要