A comparison among interpretative proposals for Random Forests
Machine Learning with Applications(2021)
摘要
The growing success of Machine Learning (ML) is making significant improvements to predictive models, facilitating their integration in various application fields. Despite its growing success, there are some limitations and disadvantages: the most significant is the lack of interpretability that does not allow users to understand how particular decisions are made. Our study focus on one of the best performing and most used models in the Machine Learning framework, the Random Forest model. It is known as an efficient model of ensemble learning, as it ensures high predictive precision, flexibility, and immediacy; it is recognized as an intuitive and understandable approach to the construction process, but it is also considered a Black Box model due to the large number of deep decision trees produced within it.
更多查看译文
关键词
Random Forest,Model interpretation,Rule extraction,inTrees,NodeHarvest
AI 理解论文
溯源树
样例
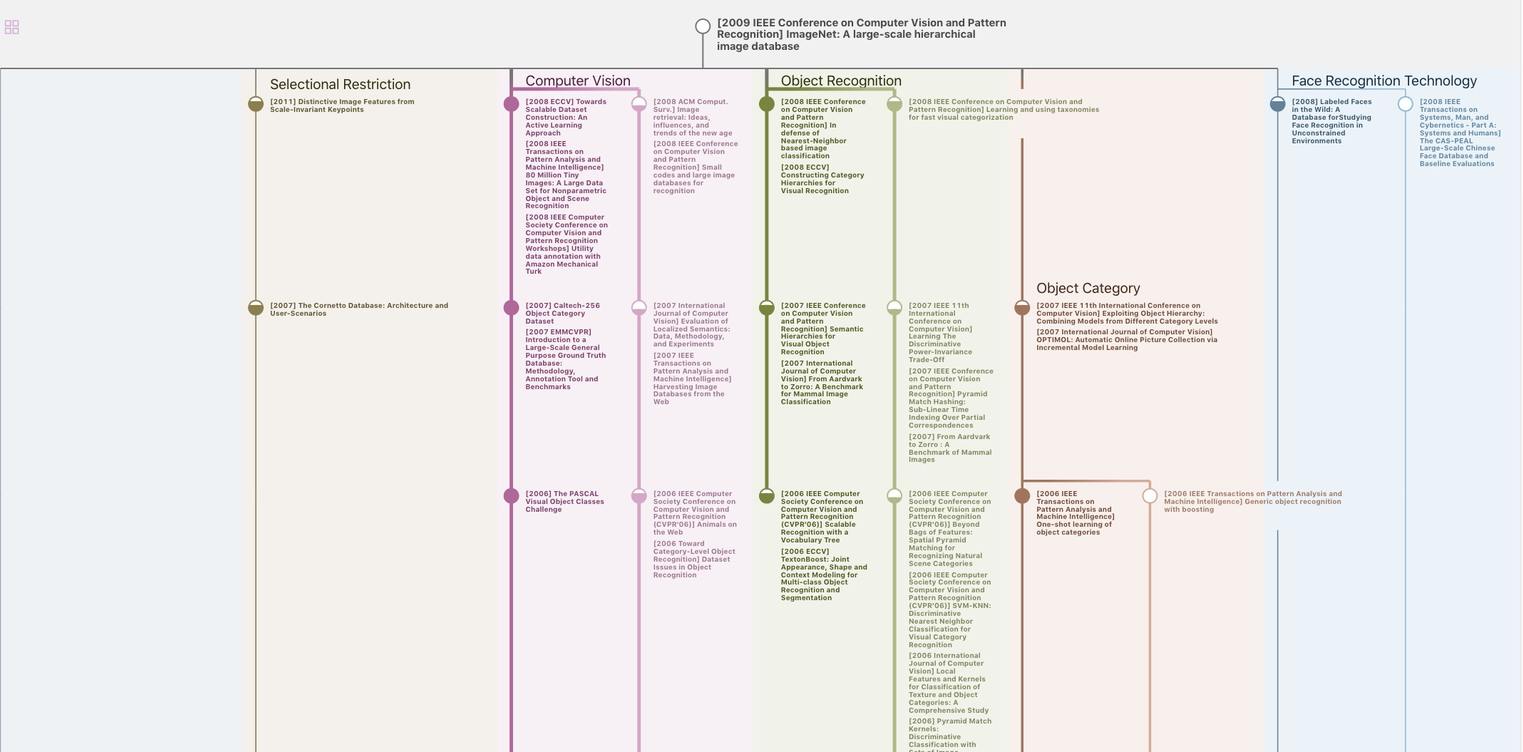
生成溯源树,研究论文发展脉络
Chat Paper
正在生成论文摘要