Fast Computation of Generalized Eigenvectors for Manifold Graph Embedding
2022 IEEE Data Science and Learning Workshop (DSLW)(2022)
摘要
Our goal is to efficiently compute low-dimensional latent coordinates for nodes in an input graph—known as graph embedding—for subsequent data processing such as clustering. Focusing on finite graphs that are interpreted as uniform samples on continuous manifolds (called manifold graphs), we leverage existing fast extreme eigenvector computation algorithms for speedy execution. We first pose a generalized eigenvalue problem for sparse matrix pair (A, B), where A = L – μQ + ∊I is a sum of graph Laplacian L and disconnected two-hop difference matrix Q. Eigenvector v minimizing Rayleigh quotient $\tfrac{\mathbf{v}^ \top \mathbf{Av}}{\mathbf{v}^ \top \mathbf{v}}$ thus minimizes 1-hop neighbor distances while maximizing distances between disconnected 2-hop neighbors, preserving graph structure. Matrix B = diag({b
i
}) that defines eigenvector orthogonality is then chosen so that boundary/interior nodes in the sampling domain have the same generalized degrees. K-dimensional latent vectors for the N graph nodes are the first K generalized eigenvectors for (A, B), computed in $\mathcal{O}(N)$ using LOBPCG, where K ≪ N. Experiments show that our embedding is among the fastest in the literature, while producing the best clustering performance for manifold graphs.
更多查看译文
关键词
Graph embedding,graph signal processing,fast eigenvector computation
AI 理解论文
溯源树
样例
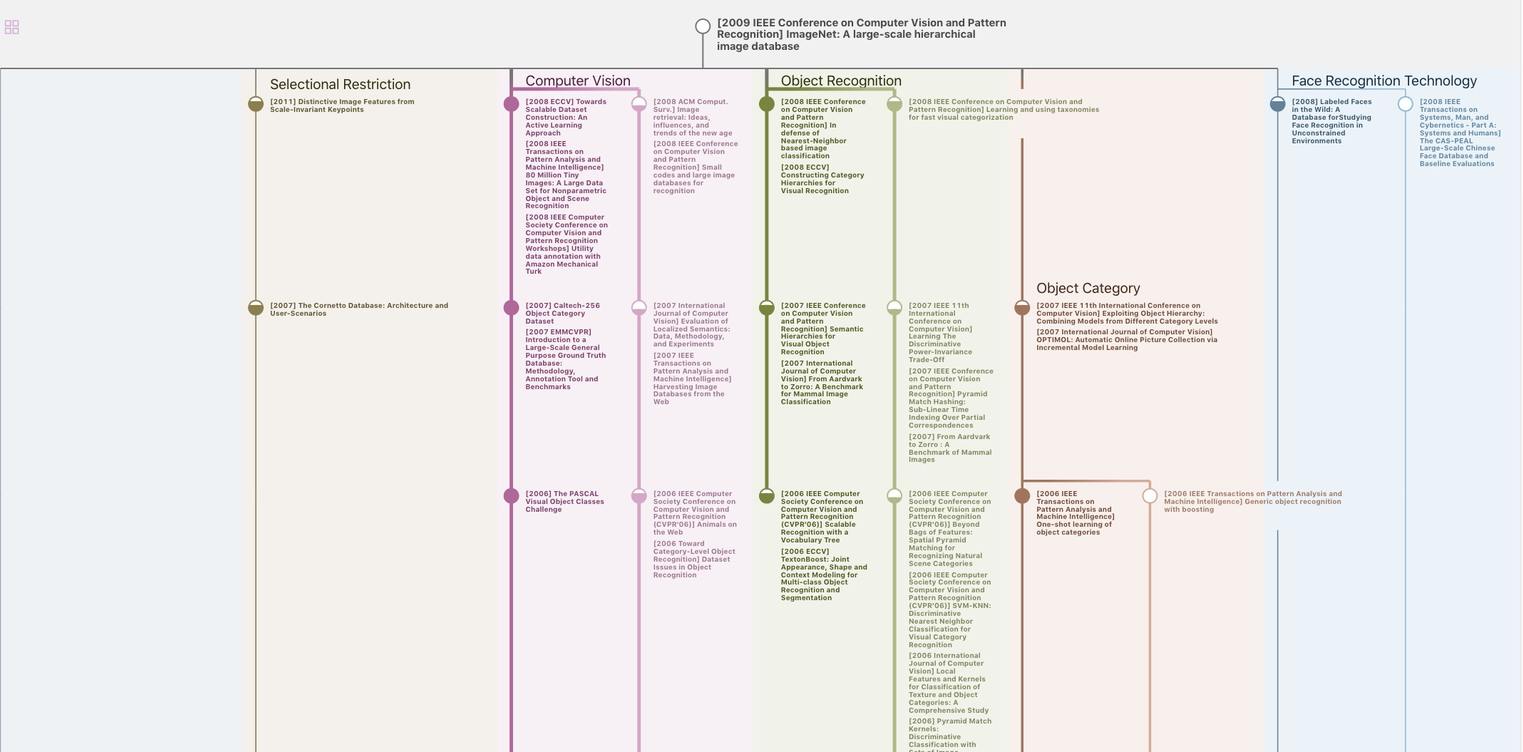
生成溯源树,研究论文发展脉络
Chat Paper
正在生成论文摘要