Multi-resolution 3D-HOG feature learning method for Alzheimer's Disease diagnosis
COMPUTER METHODS AND PROGRAMS IN BIOMEDICINE(2022)
摘要
Background and Objective: Alzheimer's Disease (AD) is a progressive irreversible neurodegeneration disease and thus timely identification is critical to delay its progression. Methods: In this work, we focus on the traditional branch to design discriminative feature extraction and selection strategies to achieve explainable AD identification. Specifically, a spatial pyramid based three-dimensional histogram of oriented gradient (3D-HOG) feature learning method is proposed. Both global and local texture changes are included in spatial pyramid 3D-HOG (SPHOG) features for comprehensive analysis. Then a modified wrapper-based feature selection algorithm is introduced to select the discriminative features for AD identification while reduce feature dimensions. Results: Discriminative SPHOG histograms with various resolutions are selected, which can represent the atrophy characteristics of cerebral cortex with promising performance. As subareas corresponding to selected histograms are consistent with clinical experience, explanatory is emphasized and illustrated with Hippocampus. Conclusion: Experimental results illustrate the effectiveness of the proposed method on feature learning based on samples obtained from common dataset and a clinical dataset. The proposed method will be useful for further medical analysis as its explanatory on other region-of-interests (ROIs) of the brain for early diagnosis of AD. (C) 2021 Elsevier B.V. All rights reserved.
更多查看译文
关键词
Feature learning, Multi-resolution, HOG, Alzheimer's Disease
AI 理解论文
溯源树
样例
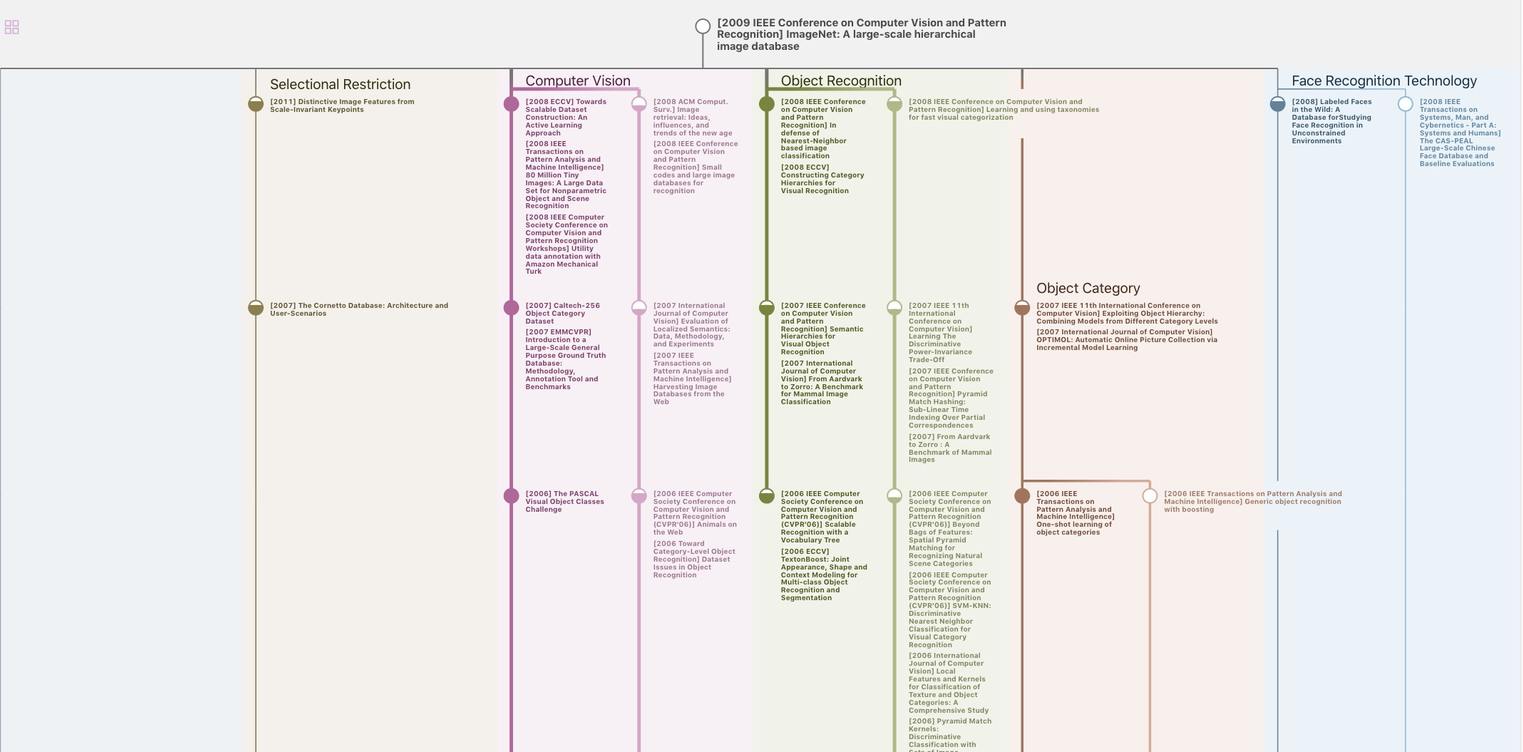
生成溯源树,研究论文发展脉络
Chat Paper
正在生成论文摘要