Two Contrasting Data Annotation Paradigms for Subjective NLP Tasks
North American Chapter of the Association for Computational Linguistics (NAACL)(2022)
摘要
Labelled data is the foundation of most natural language processing tasks. However, labelling data is difficult and there often are diverse valid beliefs about what the correct data labels should be. So far, dataset creators have acknowledged annotator subjectivity, but not actively managed it in the annotation process. This has led to partly-subjective datasets that fail to serve a clear downstream use. To address this issue, we propose two contrasting paradigms for data annotation. The descriptive paradigm encourages annotator subjectivity, whereas the prescriptive paradigm discourages it. Descriptive annotation allows for the surveying and modelling of different beliefs, whereas prescriptive annotation enables the training of models that consistently apply one belief. We discuss benefits and challenges in implementing both paradigms, and argue that dataset creators should explicitly aim for one or the other to facilitate the intended use of their dataset. Lastly, we design an annotation experiment to illustrate the contrast between the two paradigms.
更多查看译文
关键词
subjective nlp tasks,contrasting data annotation paradigms
AI 理解论文
溯源树
样例
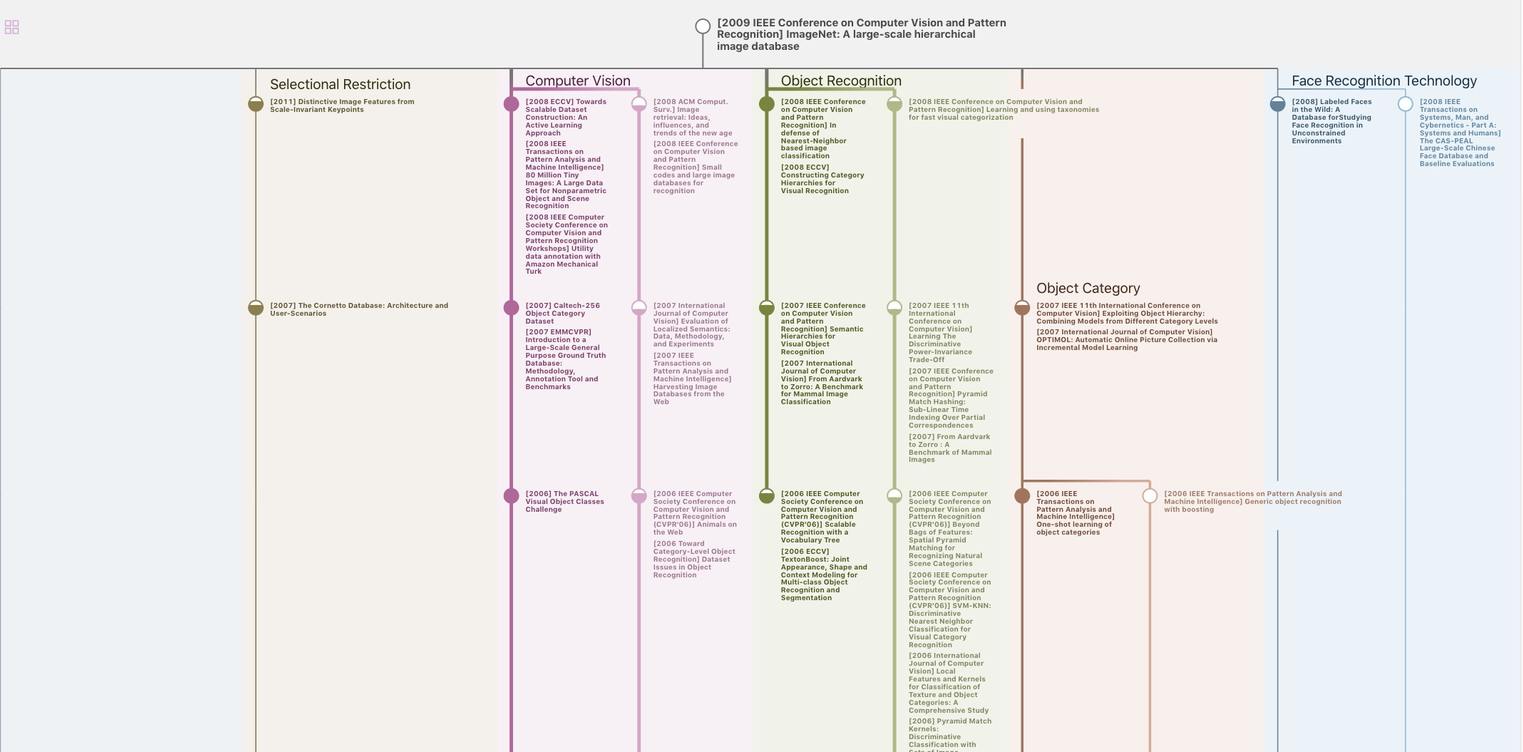
生成溯源树,研究论文发展脉络
Chat Paper
正在生成论文摘要