Non-Asymptotic Analysis of Online Multiplicative Stochastic Gradient Descent
arxiv(2023)
摘要
Past research has indicated that the covariance of the Stochastic Gradient Descent (SGD) error done via minibatching plays a critical role in determining its regularization and escape from low potential points. Motivated by some new research in this area, we prove universality results by showing that noise classes that have the same mean and covariance structure of SGD via minibatching have similar properties. We mainly consider the Multiplicative Stochastic Gradient Descent (M-SGD) algorithm as introduced in previous work, which has a much more general noise class than the SGD algorithm done via minibatching. We establish non asymptotic bounds for the M-SGD algorithm in the Wasserstein distance. We also show that the M-SGD error is approximately a scaled Gaussian distribution with mean $0$ at any fixed point of the M-SGD algorithm.
更多查看译文
关键词
online multiplicative stochastic gradient
AI 理解论文
溯源树
样例
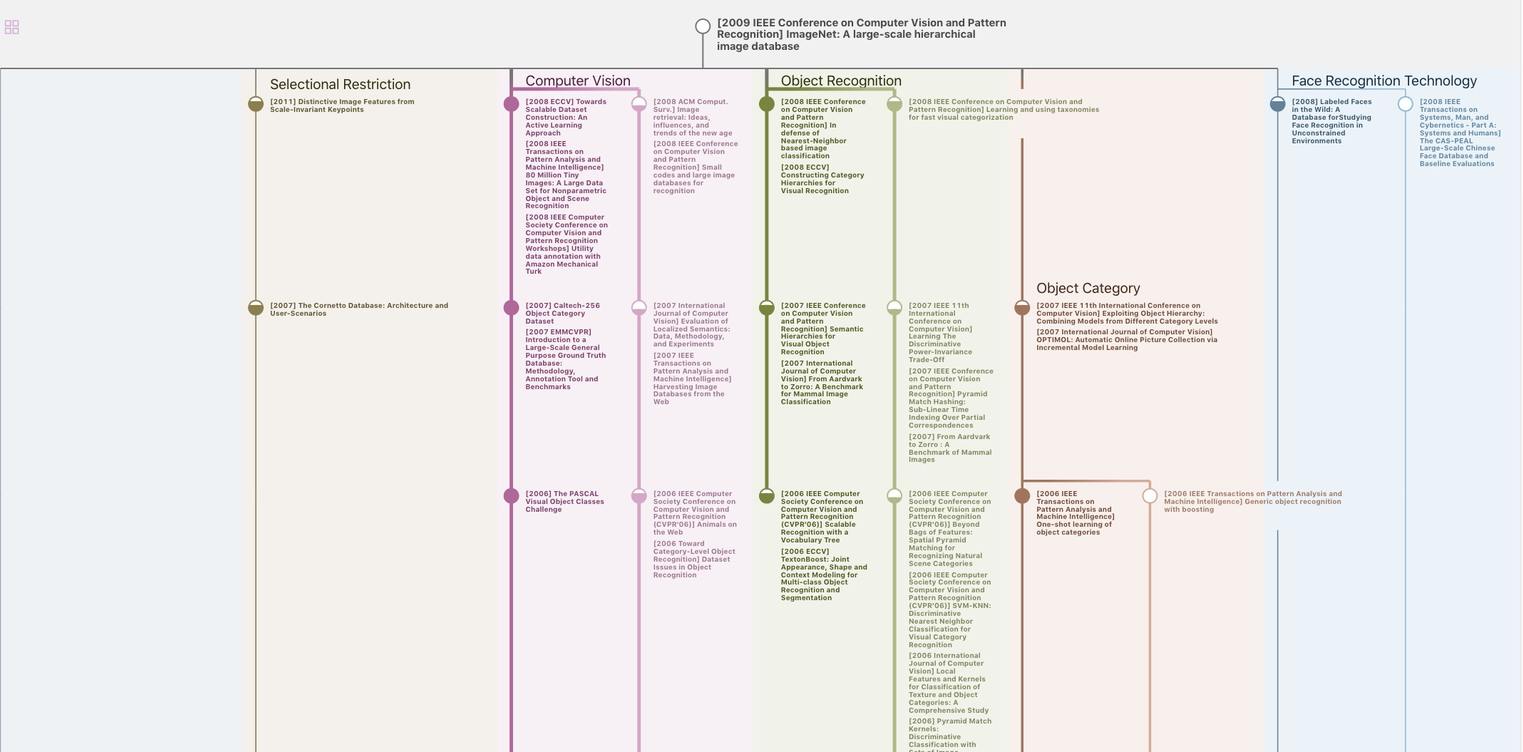
生成溯源树,研究论文发展脉络
Chat Paper
正在生成论文摘要