Robust Inspection of Micro-LED Chip Defects Using Unsupervised Anomaly Detection
12TH INTERNATIONAL CONFERENCE ON ICT CONVERGENCE (ICTC 2021): BEYOND THE PANDEMIC ERA WITH ICT CONVERGENCE INNOVATION(2021)
摘要
Inspection of defects in light emitting diode (LED) chips have been studied to reduce manufacturing cost. Recent studies proposed visual defect inspection methods based on supervised learning of deep neural networks. However, they require datasets with the ground-truth label of each chip, which accompanies prohibitively laborious tasks. In addition, they require class balanced datasets, which is difficult to obtain in an actual industrial process. In order to tackle these limitations, this paper proposes an unsupervised learning based inspection method using anomaly detection that requires no labeled data. On the micro-LED dataset, we demonstrate that our method outperforms previous anomaly detection methods. We achieve 95.82% AUROC result, which is 20.87% higher than convolutional autoencoder and 0.67% higher than Deep SVDD.
更多查看译文
关键词
Deep Learning, Micro-LED, Defect Detection, Anomaly Detection, Visual Inspection
AI 理解论文
溯源树
样例
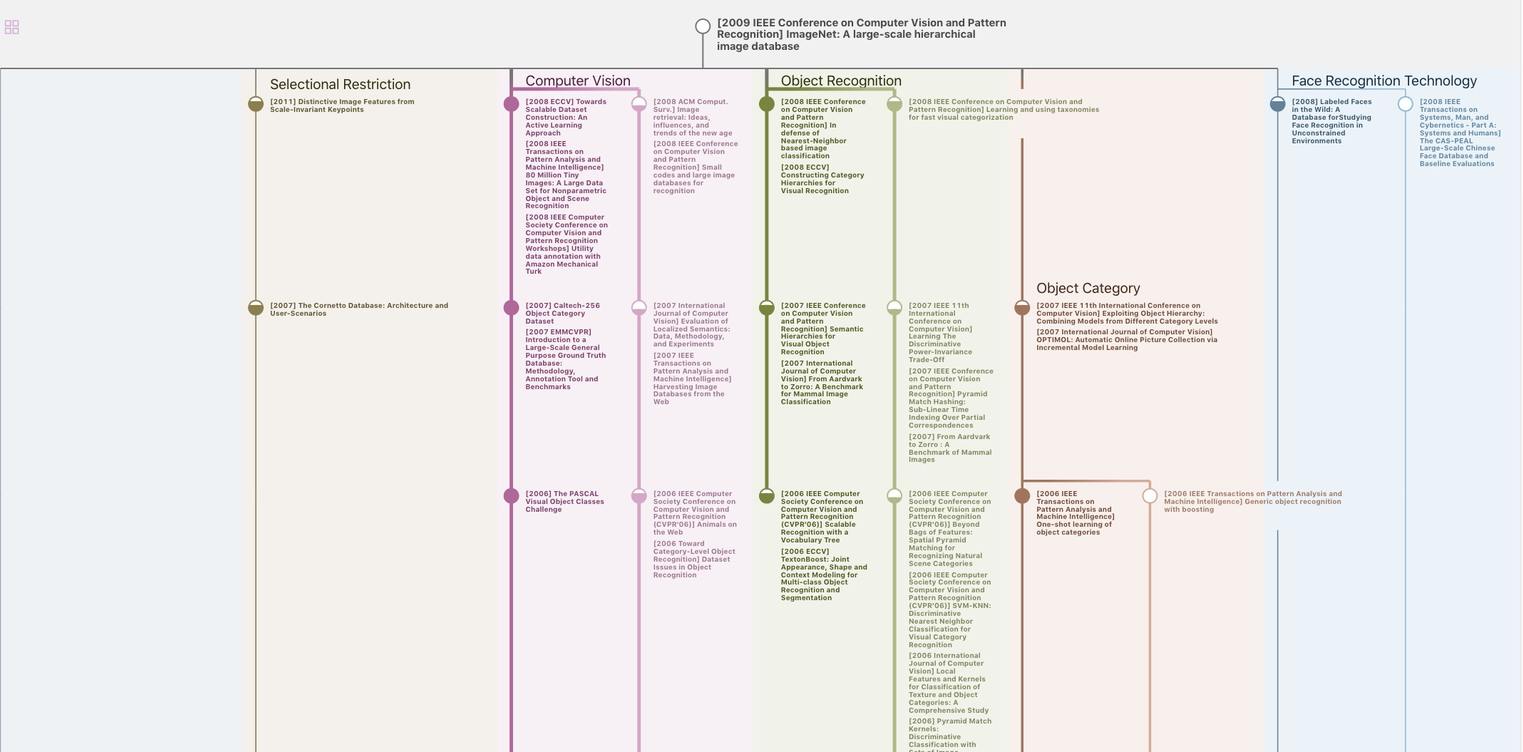
生成溯源树,研究论文发展脉络
Chat Paper
正在生成论文摘要