Depth Uncertainty Networks for Active Learning
arxiv(2021)
摘要
In active learning, the size and complexity of the training dataset changes over time. Simple models that are well specified by the amount of data available at the start of active learning might suffer from bias as more points are actively sampled. Flexible models that might be well suited to the full dataset can suffer from overfitting towards the start of active learning. We tackle this problem using Depth Uncertainty Networks (DUNs), a BNN variant in which the depth of the network, and thus its complexity, is inferred. We find that DUNs outperform other BNN variants on several active learning tasks. Importantly, we show that on the tasks in which DUNs perform best they present notably less overfitting than baselines.
更多查看译文
关键词
active learning,depth,networks,uncertainty
AI 理解论文
溯源树
样例
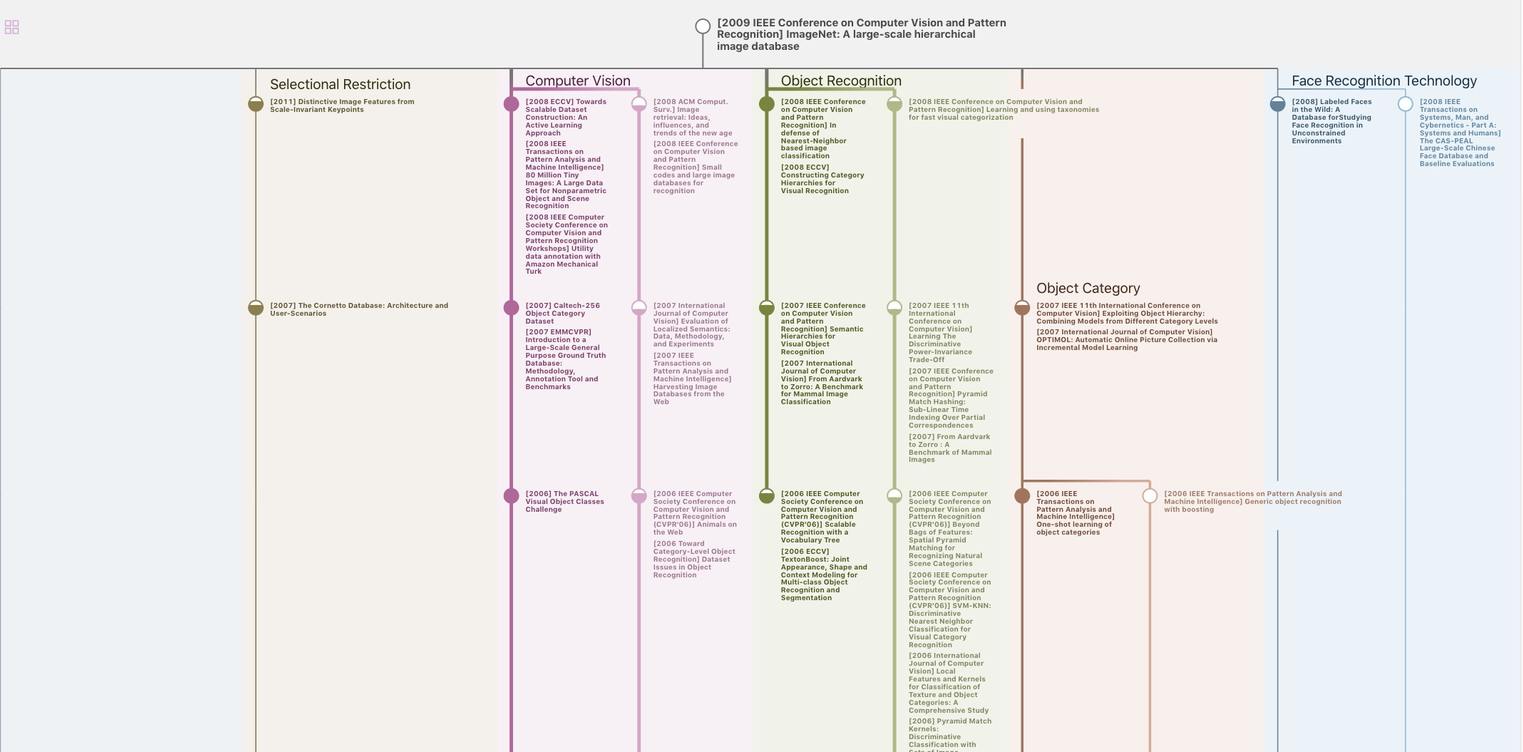
生成溯源树,研究论文发展脉络
Chat Paper
正在生成论文摘要