Deep learning in automated ultrasonic NDE – Developments, axioms and opportunities
NDT & E International(2022)
摘要
The analysis of ultrasonic NDE data has traditionally been addressed by a trained operator manually interpreting data with the support of rudimentary automation tools. Recently, many demonstrations of deep learning (DL) techniques that address individual NDE tasks (data pre-processing, defect detection, defect characterisation, and property measurement) have started to emerge in the research community. These methods have the potential to offer high flexibility, efficiency, and accuracy subject to the availability of sufficient training data. Moreover, they enable the automation of complex processes that span one or more NDE steps (e.g. detection, characterisation, and sizing). There is, however, a lack of consensus on the direction and requirements that these new methods should follow. These elements are critical to help achieve automation of ultrasonic NDE driven by artificial intelligence such that the research community, industry, and regulatory bodies embrace it. This paper reviews the state-of-the-art of autonomous ultrasonic NDE enabled by DL methodologies. The review is organised by the NDE tasks that are addressed by means of DL approaches. Key remaining challenges for each task are noted. Basic axiomatic principles for DL methods in NDE are identified based on the literature review, relevant international regulations, and current industrial needs. By placing DL methods in the context of general NDE automation levels, this paper aims to provide a roadmap for future research and development in the area.
更多查看译文
关键词
Non-destructive evaluation,Deep learning,Ultrasound,Structural integrity,Automation,Axioms
AI 理解论文
溯源树
样例
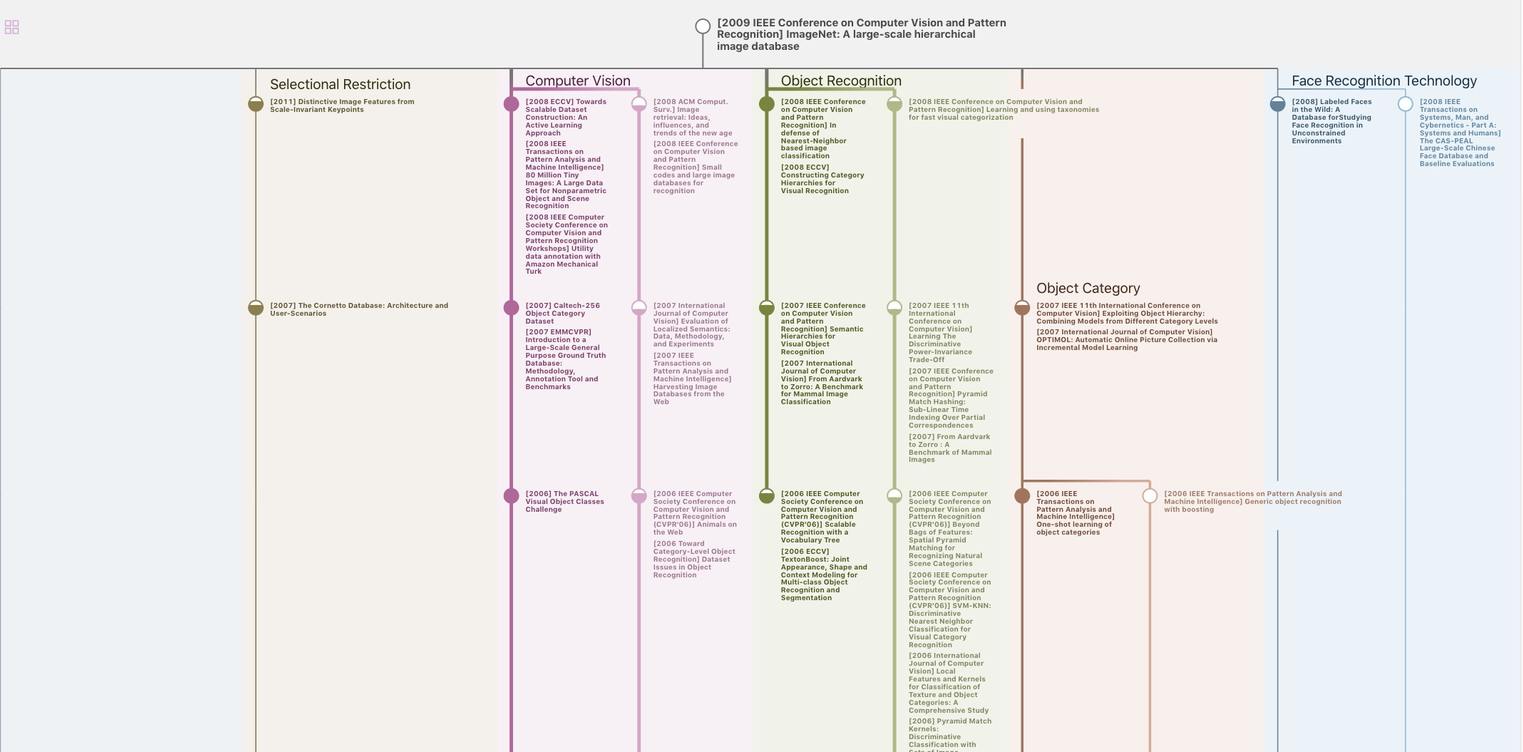
生成溯源树,研究论文发展脉络
Chat Paper
正在生成论文摘要