Accurate Computational Prediction of Core-Electron Binding Energies in Carbon-Based Materials: A Machine-Learning Model Combining Density-Functional Theory and GW
CHEMISTRY OF MATERIALS(2022)
摘要
We present a quantitatively accurate machine-learning (ML) model for the computational prediction of core-electron binding energies, from which X-ray photoelectron spectroscopy (XPS) spectra can be readily obtained. Our model combines density functional theory (DFT) with GW and uses kernel ridge regression for the ML predictions. We apply the new approach to disordered materials and small molecules containing carbon, hydrogen, and oxygen and obtain qualitative and quantitative agreement with experiment, resolving spectral features within 0.1 eV of reference experimental spectra. The method only requires the user to provide a structural model for the material under study to obtain an XPS prediction within seconds. Our new tool is freely available online through the XPS Prediction Server.
更多查看译文
关键词
energies,core-electron,carbon-based,machine-learning,density-functional
AI 理解论文
溯源树
样例
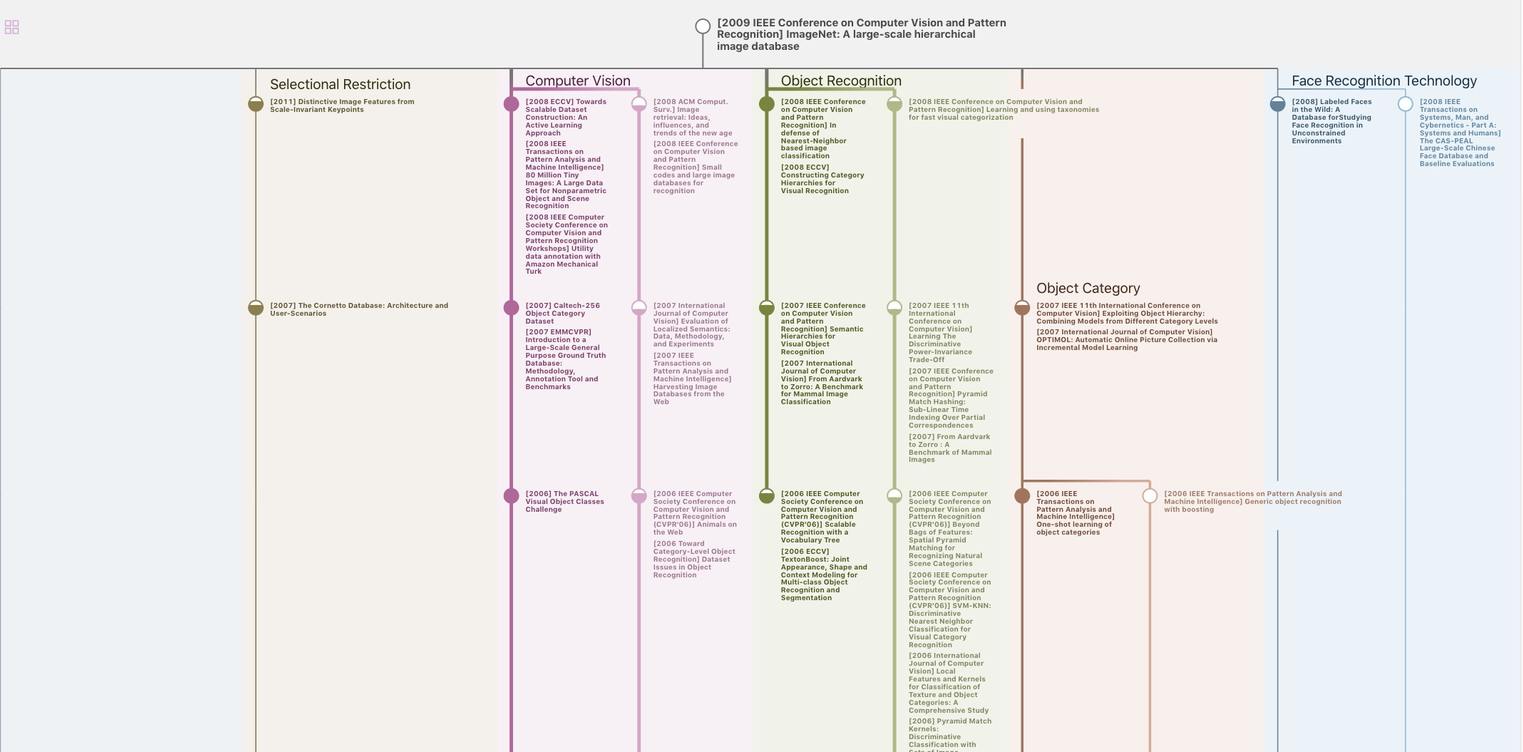
生成溯源树,研究论文发展脉络
Chat Paper
正在生成论文摘要