Graph-based hierarchical record clustering for unsupervised entity resolution
arxiv(2021)
摘要
Here we study the problem of matched record clustering in unsupervised entity resolution. We build upon a state-of-the-art probabilistic framework named the Data Washing Machine (DWM). We introduce a graph-based hierarchical 2-step record clustering method (GDWM) that first identifies large, connected components or, as we call them, soft clusters in the matched record pairs using a graph-based transitive closure algorithm utilized in the DWM. That is followed by breaking down the discovered soft clusters into more precise entity clusters in a hierarchical manner using an adapted graph-based modularity optimization method. Our approach provides several advantages over the original implementation of the DWM, mainly a significant speed-up, increased precision, and overall increased F1 scores. We demonstrate the efficacy of our approach using experiments on multiple synthetic datasets. Our results also provide evidence of the utility of graph theory-based algorithms despite their sparsity in the literature on unsupervised entity resolution.
更多查看译文
关键词
hierarchical record clustering,unsupervised entity
AI 理解论文
溯源树
样例
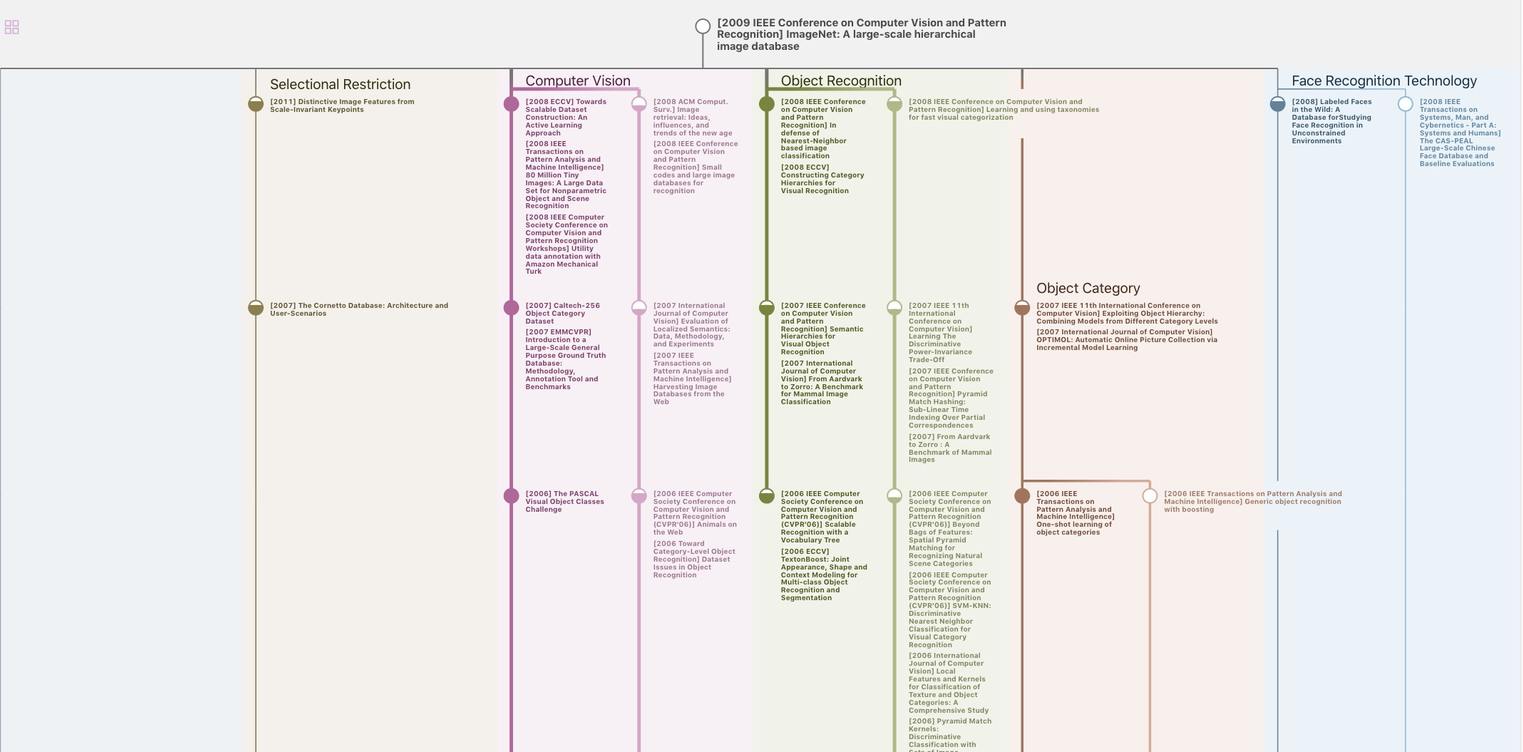
生成溯源树,研究论文发展脉络
Chat Paper
正在生成论文摘要