Injecting Numerical Reasoning Skills into Knowledge Base Question Answering Models
arxiv(2021)
摘要
Embedding-based methods are popular for Knowledge Base Question Answering (KBQA), but few current models have numerical reasoning skills and thus struggle to answer ordinal constrained questions. This paper proposes a new embedding-based KBQA framework which particularly takes numerical reasoning into account. We present NumericalTransformer on top of NSM, a state-of-the-art embedding-based KBQA model, to create NT-NSM. To enable better training, we propose two pre-training tasks with explicit numerical-oriented loss functions on two generated training datasets and a template-based data augmentation method for enriching ordinal constrained QA dataset. Extensive experiments on KBQA benchmarks demonstrate that with the help of our training algorithm, NT-NSM is empowered with numerical reasoning skills and substantially outperforms the baselines in answering ordinal constrained questions.
更多查看译文
关键词
numerical reasoning skills,answering models,knowledge base question
AI 理解论文
溯源树
样例
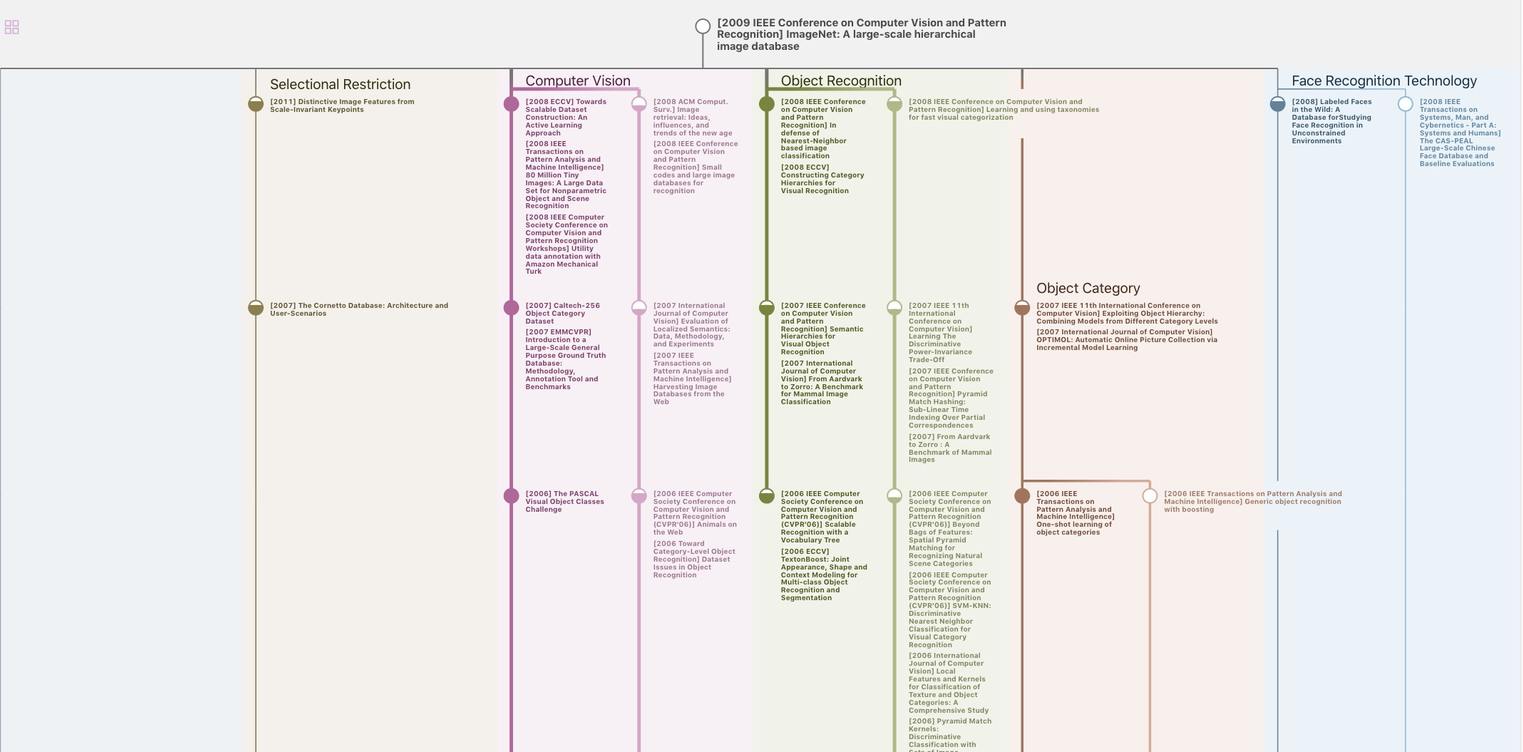
生成溯源树,研究论文发展脉络
Chat Paper
正在生成论文摘要