Building a great multi-lingual teacher with sparsely-gated mixture of experts for speech recognition
arxiv(2021)
摘要
The sparsely-gated Mixture of Experts (MoE) can magnify a network capacity with a little computational complexity. In this work, we investigate how multi-lingual Automatic Speech Recognition (ASR) networks can be scaled up with a simple routing algorithm in order to achieve better accuracy. More specifically, we apply the sparsely-gated MoE technique to two types of networks: Sequence-to-Sequence Transformer (S2S-T) and Transformer Transducer (T-T). We demonstrate through a set of ASR experiments on multiple language data that the MoE networks can reduce the relative word error rates by 16.5\% and 4.7\% with the S2S-T and T-T, respectively. Moreover, we thoroughly investigate the effect of the MoE on the T-T architecture in various conditions: streaming mode, non-streaming mode, the use of language ID and the label decoder with the MoE.
更多查看译文
关键词
teacher,speech,experts,multi-lingual,sparsely-gated
AI 理解论文
溯源树
样例
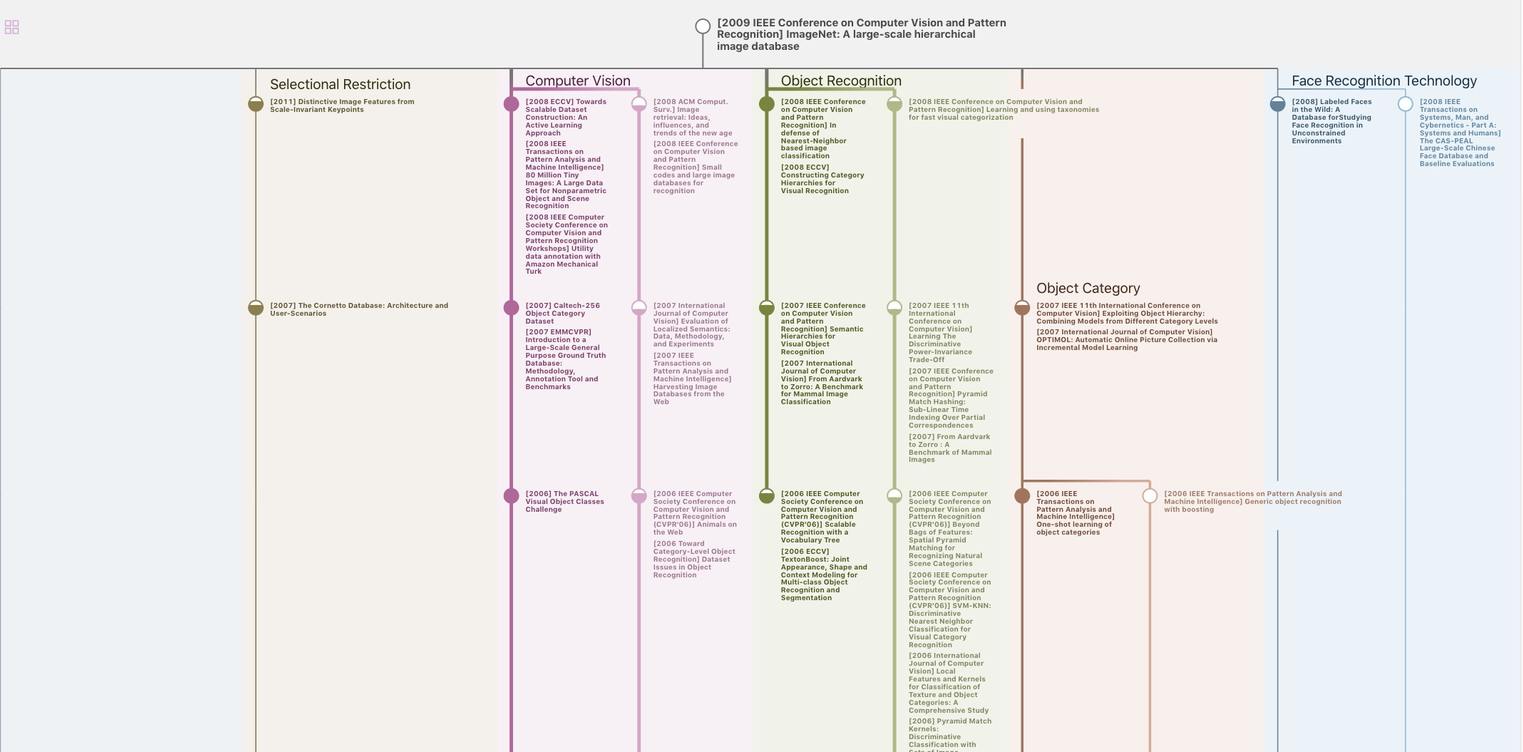
生成溯源树,研究论文发展脉络
Chat Paper
正在生成论文摘要