Pruning Pretrained Encoders with a Multitask Objective
arxiv(2021)
摘要
The sizes of pretrained language models make them challenging and expensive to use when there are multiple desired downstream tasks. In this work, we adopt recent strategies for model pruning during finetuning to explore the question of whether it is possible to prune a single encoder so that it can be used for multiple tasks. We allocate a fixed parameter budget and compare pruning a single model with a multitask objective against the best ensemble of single-task models. We find that under two pruning strategies (element-wise and rank pruning), the approach with the multitask objective outperforms training models separately when averaged across all tasks, and it is competitive on each individual one. Additional analysis finds that using a multitask objective during pruning can also be an effective method for reducing model sizes for low-resource tasks.
更多查看译文
关键词
encoders,multitask objective
AI 理解论文
溯源树
样例
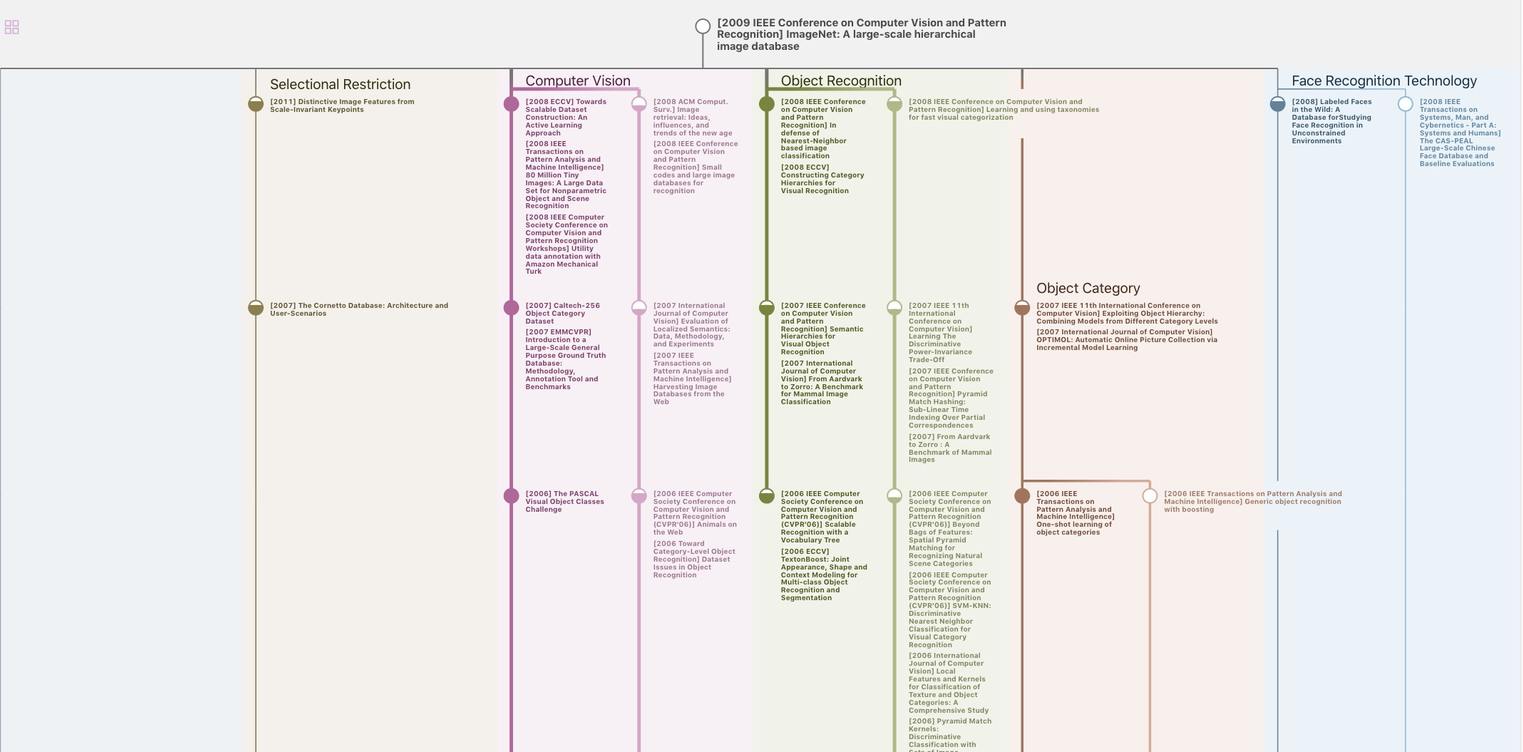
生成溯源树,研究论文发展脉络
Chat Paper
正在生成论文摘要