Multivariate Dynamic Modeling for Bayesian Forecasting of Business Revenue
arxiv(2021)
摘要
Forecasting enterprise-wide revenue is critical to many companies and presents several challenges and opportunities for significant business impact. This case study is based on model developments to address these challenges for forecasting in a large-scale retail company. Focused on multivariate revenue forecasting across collections of supermarkets and product Categories, hierarchical dynamic models are natural: these are able to couple revenue streams in an integrated forecasting model, while allowing conditional decoupling to enable relevant and sensitive analysis together with scalable computation. Structured models exploit multi-scale modeling to cascade information on price and promotion activities as predictors relevant across Categories and groups of stores. With a context-relevant focus on forecasting revenue 12 weeks ahead, the study highlights product Categories that benefit from multi-scale information, defines insights into when, how and why multivariate models improve forecast accuracy, and shows how cross-Category dependencies can relate to promotion decisions in one Category impacting others. Bayesian modeling developments underlying the case study are accessible in custom code for interested readers.
更多查看译文
关键词
Bayesian state space models,commercial forecasting systems,decouple,recouple,forecast assessment,multi-scale hierarchical models,revenue forecasting,supermarket sales forecasting
AI 理解论文
溯源树
样例
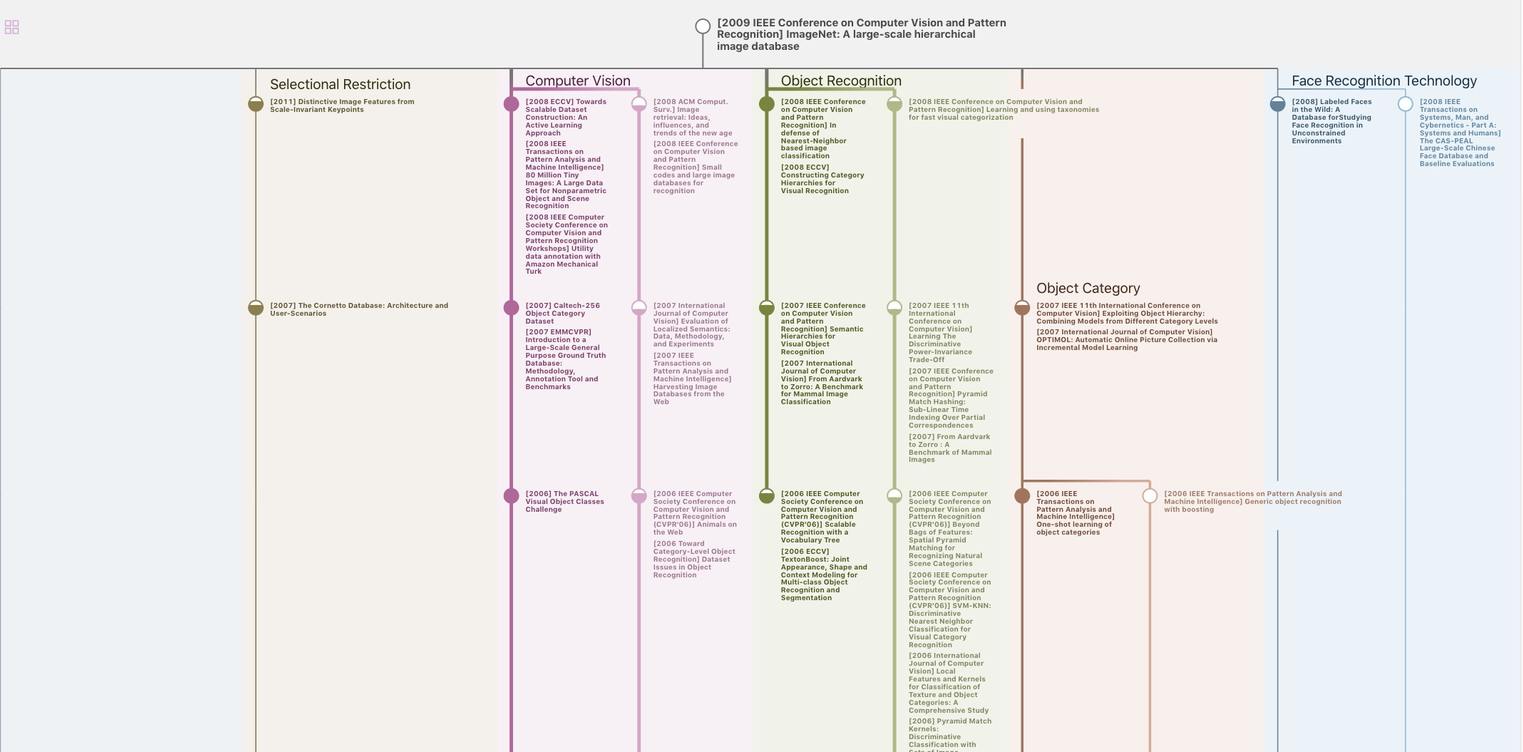
生成溯源树,研究论文发展脉络
Chat Paper
正在生成论文摘要