Error Diagnosis of Deep Monocular Depth Estimation Models
2021 IEEE/RSJ INTERNATIONAL CONFERENCE ON INTELLIGENT ROBOTS AND SYSTEMS (IROS)(2021)
摘要
Estimating depth from a monocular image is an ill-posed problem: when the camera projects a 3D scene onto a 2D plane, depth information is inherently and permanently lost. Nevertheless, recent work has shown impressive results in estimating 3D structure from 2D images using deep learning. In this paper, we put on an introspective hat and analyze state-of-the-art monocular depth estimation models in indoor scenes to understand these models’ limitations and error patterns. To address errors in depth estimation, we introduce a novel Depth Error Detection Network (DEDN) that spatially identifies erroneous depth predictions in the monocular depth estimation models. By experimenting with multiple state-of-the-art monocular indoor depth estimation models on multiple datasets, we show that our proposed depth error detection network can identify a significant number of errors in the predicted depth maps. Our module is flexible and can be readily plugged into any monocular depth prediction network to help diagnose its results. Additionally, we propose a simple yet effective Depth Error Correction Network (DECN) that iteratively corrects errors based on our initial error diagnosis.
更多查看译文
关键词
depth,deep,models,estimation
AI 理解论文
溯源树
样例
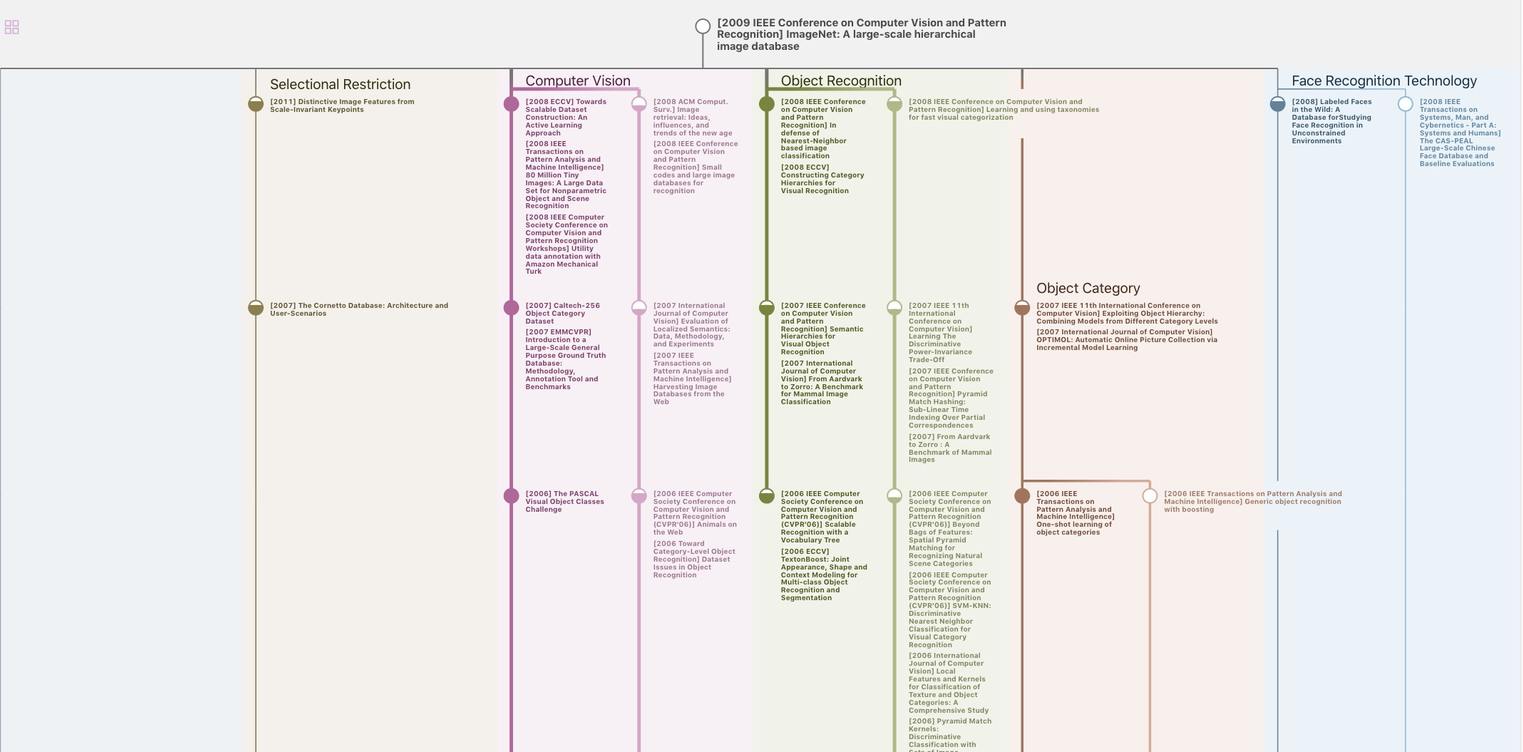
生成溯源树,研究论文发展脉络
Chat Paper
正在生成论文摘要