Surrogate-data-enriched Physics-Aware Neural Networks.
NLDL(2022)
摘要
Neural networks can be used as surrogates for PDE models. They can be made physics-aware by penalizing underlying equations or the conservation of physical properties in the loss function during training. Current approaches allow to additionally respect data from numerical simulations or experiments in the training process. However, this data is frequently expensive to obtain and thus only scarcely available for complex models. In this work, we investigate how physics-aware models can be enriched with computationally cheaper, but inexact, data from other surrogate models like Reduced-Order Models (ROMs). In order to avoid trusting too-low-fidelity surrogate solutions, we develop an approach that is sensitive to the error in inexact data. As a proof of concept, we consider the one-dimensional wave equation and show that the training accuracy is increased by two orders of magnitude when inexact data from ROMs is incorporated.
更多查看译文
关键词
neural networks,surrogate-data-enriched,physics-aware
AI 理解论文
溯源树
样例
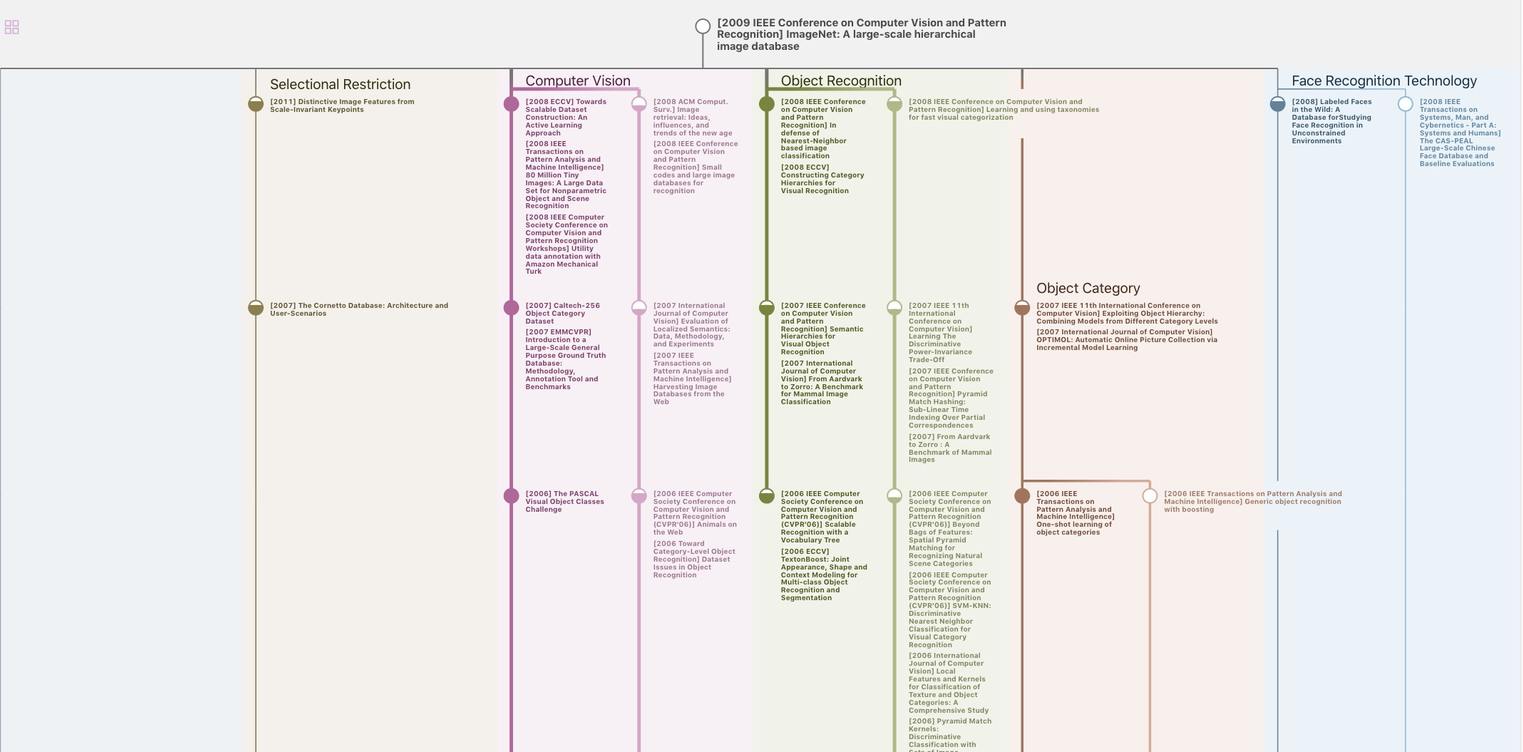
生成溯源树,研究论文发展脉络
Chat Paper
正在生成论文摘要