Design and Validation of a Sensor Fault-Tolerant Module for Real-Time High-Density EMG Pattern Recognition
2021 43RD ANNUAL INTERNATIONAL CONFERENCE OF THE IEEE ENGINEERING IN MEDICINE & BIOLOGY SOCIETY (EMBC)(2021)
摘要
With the advancements in electronics technology, high-density (HD) EMG sensing systems have become available and have been investigated for their feasibility and performance in neural-machine interface (NMI) applications. Comparing to the traditional single channel-based targeted muscle sensing method, HD EMG sensing performs a sampling of the electrical activity over a larger surface area and has the promise of 1) providing richer neural information from one temporal and two spatial dimensions and 2) ease of wear in real life without the need of anatomically targeted electrode placement. To use HD EMG in real-time NMI applications, challenges including high computational burden and unreliability of EMG recordings over time need to be addressed. This paper presented an HD EMG PR based NMI which seamlessly integrates HD EMG PR with a Sensor Fault-Tolerant Module (SFTM) which aimed to provide robust PR in real time. Experimental results showed that the SFTM was able to recover the PR accuracies by 6%-22% from disturbances including contact artifacts and loose contacts. A Python-based implementation of the proposed HD EMG SFTM was developed and was demonstrated to be computationally efficient for real-time performance. These results have demonstrated the feasibility of a robust real-time HD EMG PR-based NMI.
更多查看译文
关键词
Algorithms,Artifacts,Electrodes,Electromyography
AI 理解论文
溯源树
样例
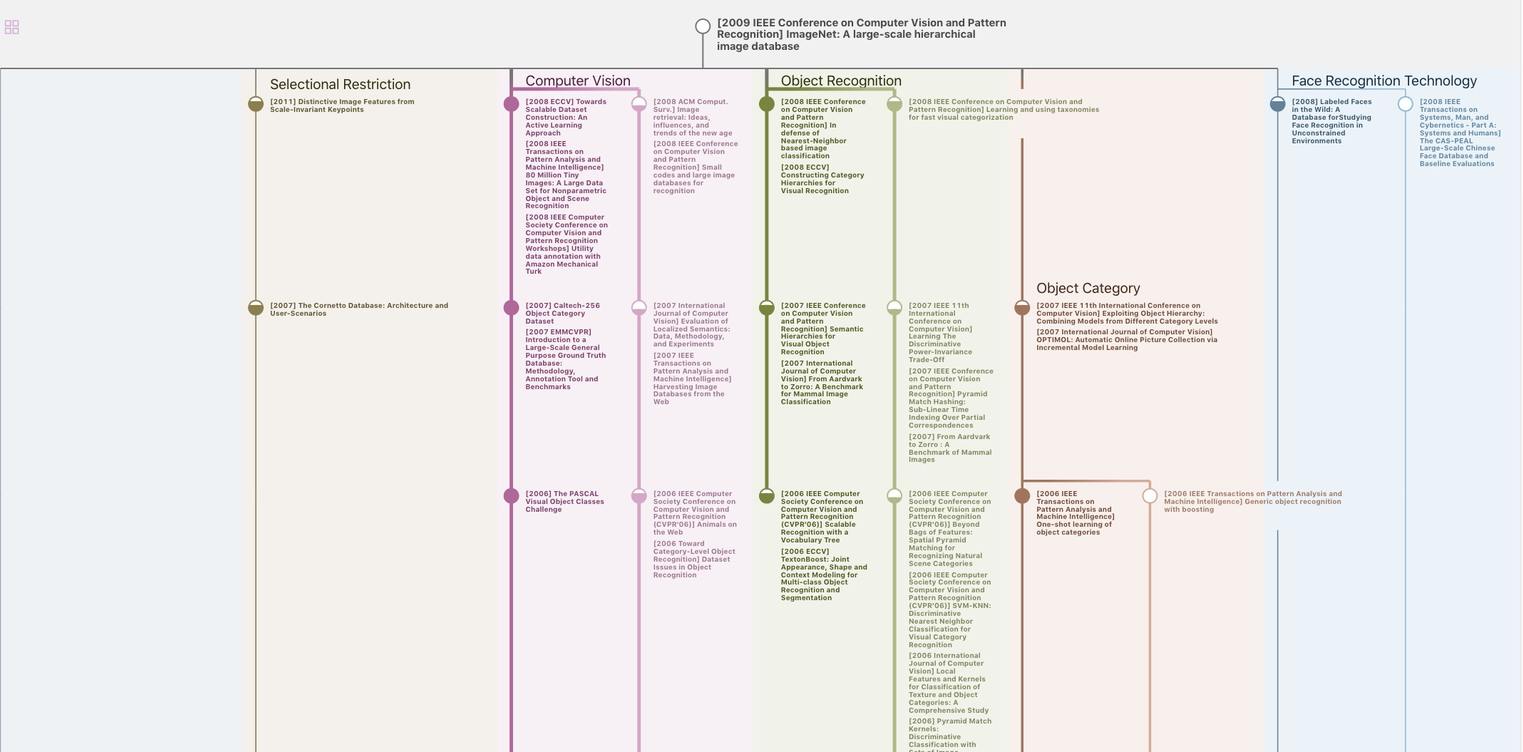
生成溯源树,研究论文发展脉络
Chat Paper
正在生成论文摘要