Self-supervised Projection Denoising for Low-Dose Cone-Beam CT
2021 43RD ANNUAL INTERNATIONAL CONFERENCE OF THE IEEE ENGINEERING IN MEDICINE & BIOLOGY SOCIETY (EMBC)(2021)
摘要
We consider the problem of denoising low-dose x-ray projections for cone-beam CT, where x-ray measurements are typically modeled as signal corrupted by Poisson noise. Since each projection view is a 2D image, we regard the low-dose projection views as examples to train a convolutional neural network. For self-supervised training without ground truth, we partially blind noisy projections and train the denoising model to recover the blind spots of projection views. From the projection views denoised by the learned model, we can reconstruct a high-quality 3D volume with a reconstruction algorithm such as the standard filtered backprojection. Through a series of phantom experiments, our self-supervised denoising approach simultaneously reduces noise level and restores structural information in cone-beam CT images.
更多查看译文
关键词
Algorithms,Cone-Beam Computed Tomography,Image Processing, Computer-Assisted,Phantoms, Imaging,Tomography, X-Ray Computed
AI 理解论文
溯源树
样例
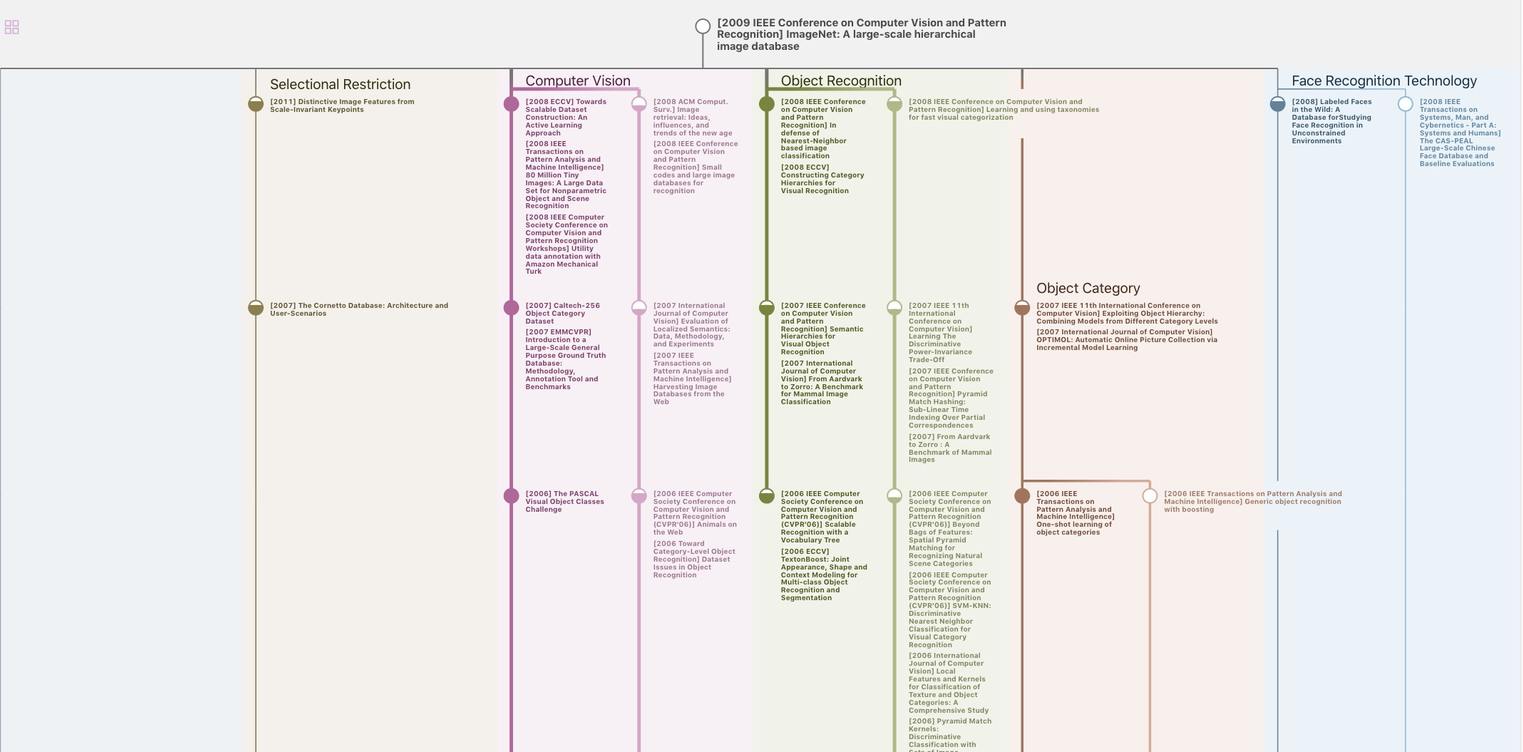
生成溯源树,研究论文发展脉络
Chat Paper
正在生成论文摘要