Influence of Study Composition on the Efficacy of Sleep Detection Using Actigraphy
2021 43RD ANNUAL INTERNATIONAL CONFERENCE OF THE IEEE ENGINEERING IN MEDICINE & BIOLOGY SOCIETY (EMBC)(2021)
摘要
Wearable actigraphy sensors have been useful tools for unobtrusive monitoring of sleep. The influence of the composition and characteristics of study groups such as normal sleep versus sleep disorders affecting the efficacy of sleep assessment using actigraphy has not been fully examined. In this study, we present multi-variate sleep models using actigraphy features obtained from wrist-worn sensors and evaluate the efficacy of sleep detection compared to the overnight polysomnography from two unique datasets: overnight actigraphy recordings in a control population of young healthy individuals (n=31) and 24-hour actigraphy recordings in a more heterogeneous population (n=27) comprised of normal and abnormal sleepers. We evaluate the performance of actigraphy derived logistic regression (LR) and random forest (RF) sleep models for both intra-dataset and inter-dataset training and cross-validation. Both the LR and RF sleep models for the healthy sleep dataset show an area under the receiver operating characteristic (AUROC) of 0.85 +/- 0.02 in the control sleep dataset among 50 random splits of training and testing evaluations. We find the AUROC performance from the heterogeneous sleep dataset involving sleep disorders to be relatively lower as 0.74 +/- 0.05 and 0.80 +/- 0.03 for LR and RF sleep models, respectively. Optimal sleep models trained using heterogeneous datasets perform very well when tested with the normal sleep dataset producing accuracy of similar to 92%. Our study supports that using a more diverse training set benefits the sleep classifier model to be more generalizable for both healthy and abnormal sleepers.
更多查看译文
关键词
Modeling and analysis, Physiological monitoring - Modeling and analysis, Health monitoring applications
AI 理解论文
溯源树
样例
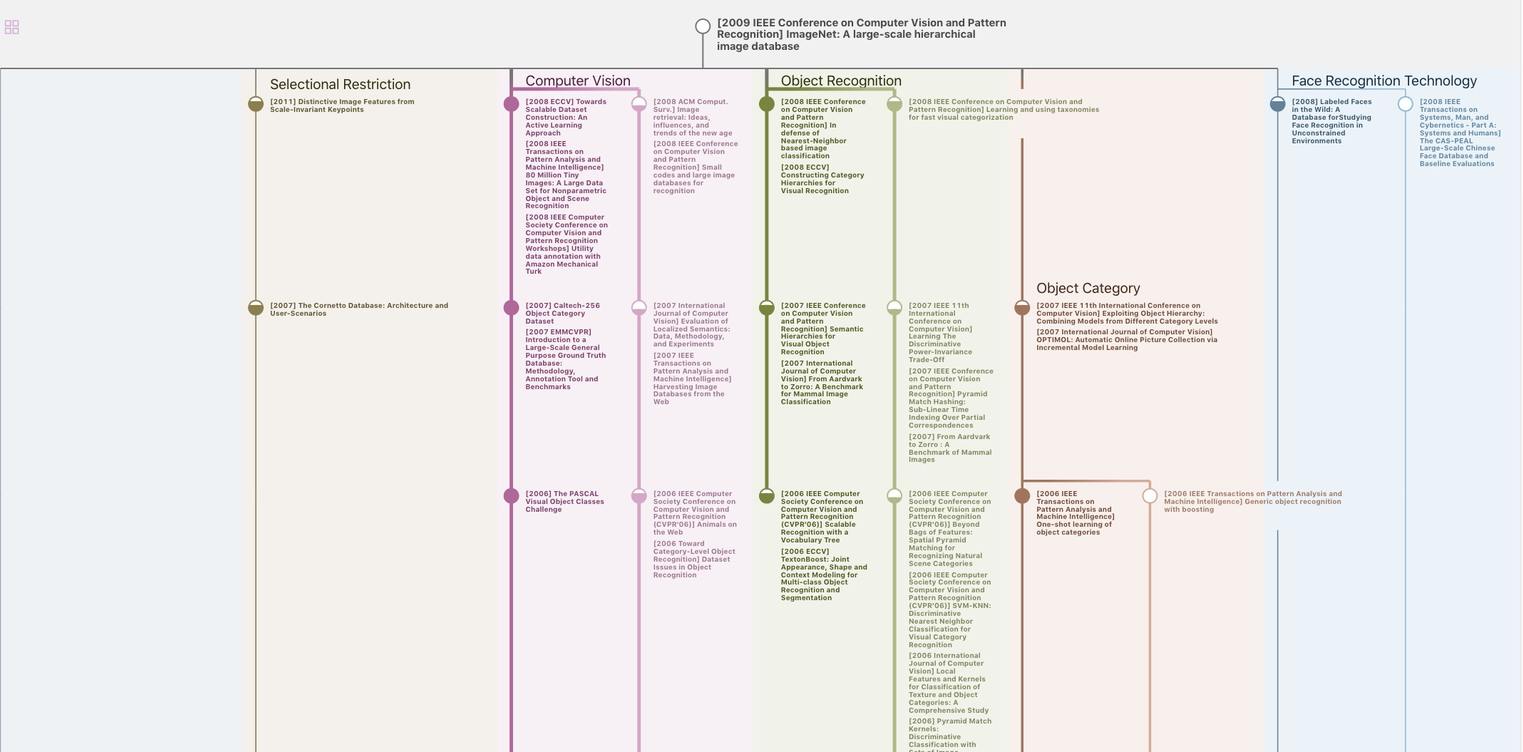
生成溯源树,研究论文发展脉络
Chat Paper
正在生成论文摘要