A Partial Label-Based Machine Learning Approach For Cervical Whole-Slide Image Classification: The Winning TissueNet Solution
2021 43RD ANNUAL INTERNATIONAL CONFERENCE OF THE IEEE ENGINEERING IN MEDICINE & BIOLOGY SOCIETY (EMBC)(2021)
摘要
Cervical cancer is the fourth most common cancer in women worldwide. To determine early treatment for patients, it is critical to accurately classify the cervical intraepithelial lesion status based on a microscopic biopsy. Lesion classification is a 4-class problem, with biopsies being designated as benign or increasingly malignant as class 1-3, with 3 being invasive cancer. Unfortunately, traditional biopsy analysis by a pathologist is time-consuming and subject to intra- and inter-observer variability. For this reason, it is of interest to develop automatic analysis pipelines to classify lesion status directly from a digitalized whole slide image (WSI). The recent TissueNet Challenge was organized to find the best automatic detection pipeline for this task, using a dataset of 1015 annotated WSI slides. In this work, we present our winning end-to-end solution for cervical slide classification composed of a two-step classification model: First, we classify individual slide patches using an ensemble CNN, followed by an SVM-based slide classification using statistical features of the aggregated patch-level predictions. Importantly, we present the key innovation of our approach, which is a novel partial label-based loss function that allows us to supplement the supervised WSI patch annotations with weakly supervised patches based on the WSI class. This led to us not requiring additional expert tissue annotation, while still reaching the winning score of 94.7%. Our approach is a step towards the clinical inclusion of automatic pipelines for cervical cancer treatment planning.Clinical relevance— The explanation of the winning Tis-sueNet AI algorithm for automated cervical cancer classification, which may provide insights for the next generation of computer assisted tools in digital pathology.
更多查看译文
关键词
classification,machine learning,label-based,whole-slide
AI 理解论文
溯源树
样例
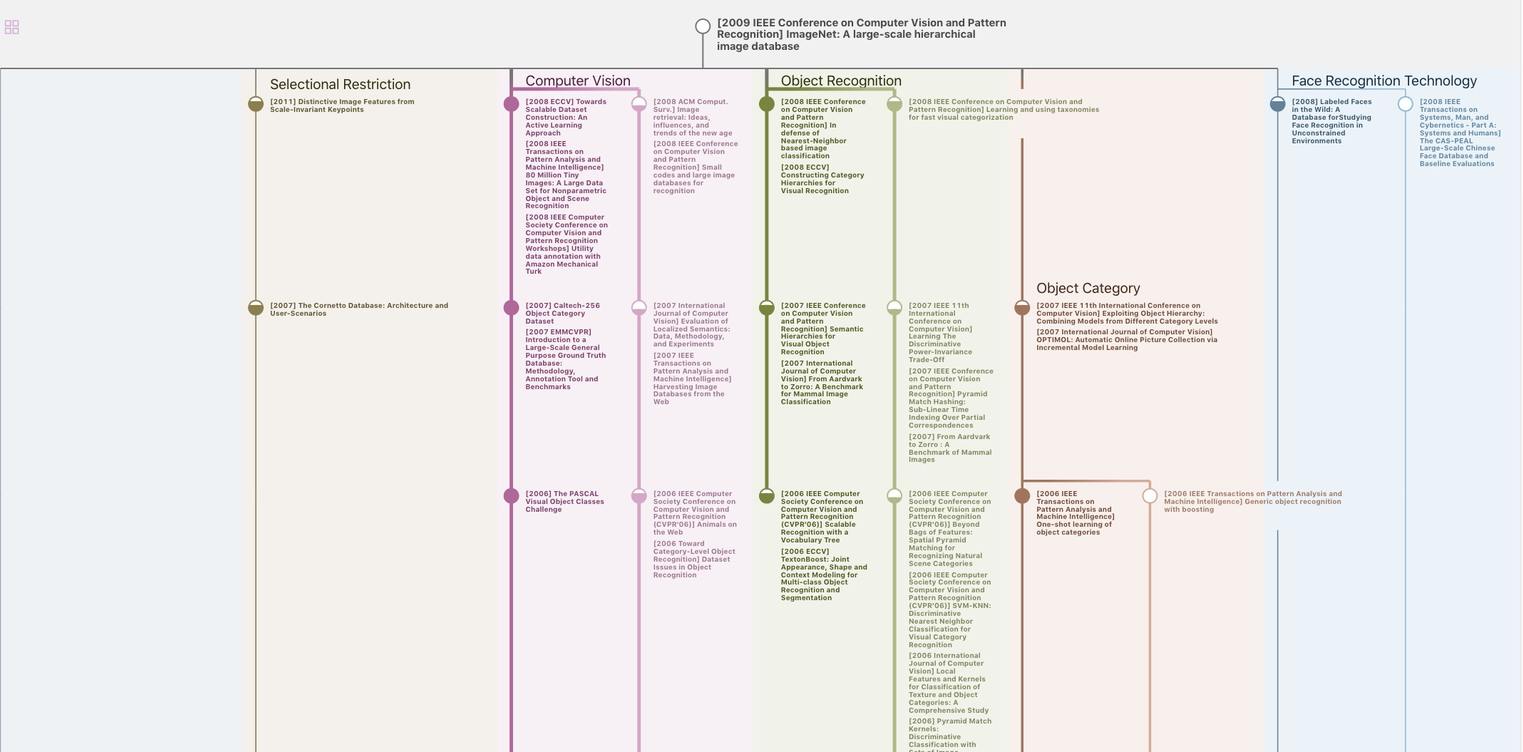
生成溯源树,研究论文发展脉络
Chat Paper
正在生成论文摘要