Estimation of Joint Angle From sEMG and Inertial Measurements Based on Deep Learning Approach
2021 43RD ANNUAL INTERNATIONAL CONFERENCE OF THE IEEE ENGINEERING IN MEDICINE & BIOLOGY SOCIETY (EMBC)(2021)
摘要
Continuous kinematics estimation from surface electromyography (sEMG) allows more natural and intuitive human-machine collaboration. Recent research has suggested the use of multimodal inputs (sEMG signals and inertial measurements) to improve estimation performance. This work focused on assessing the use of angular velocity in combination with myoelectric signals to simultaneously and continuously predict 12 joint angles in the hand. Estimation performance was evaluated for five functional and grasping movements in 20 subjects. The proposed method is based on convolutional and recurrent neural networks using transfer learning (TL). A novel aspect was the use of a pretrained deep network model from basic joint hand movements to learn new patterns present in functional motions. A comparison was carried out with the traditional method based solely on sEMG. Although the performance of the algorithm slightly improved with the use of the multimodal combination, both strategies had similar behavior. The results indicated a significant improvement for a single task: opening a bottle with a tripod grasp.
更多查看译文
关键词
Angle estimation, angular velocity, deep learning, inertial measurements, recurrent and convolutional neural networks (RCNN), surface electromyography (sEMG), transfer learning (TL)
AI 理解论文
溯源树
样例
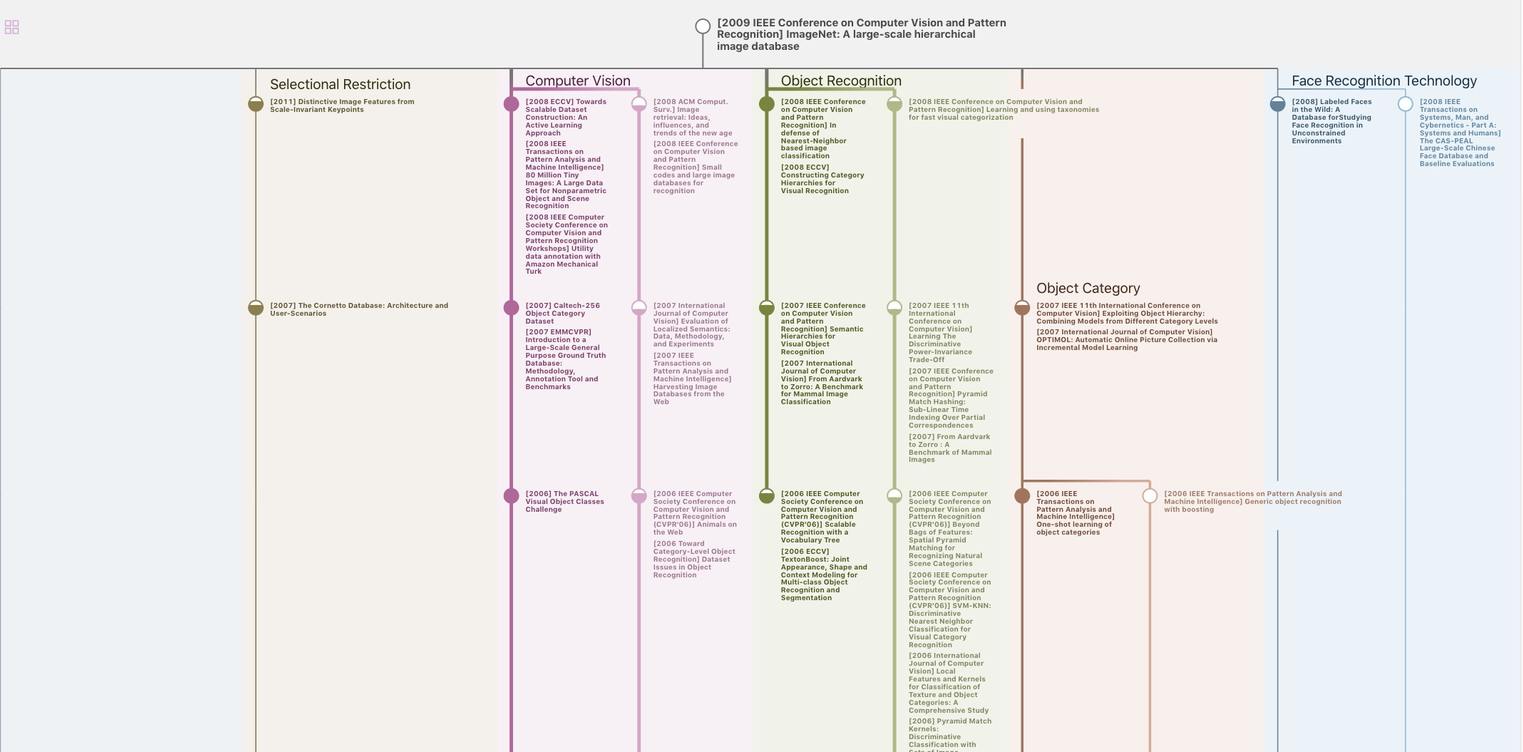
生成溯源树,研究论文发展脉络
Chat Paper
正在生成论文摘要