A comprehensive evaluation of state-of-the-art time-series deep learning models for activity-recognition in post-stroke rehabilitation assessment
2021 43RD ANNUAL INTERNATIONAL CONFERENCE OF THE IEEE ENGINEERING IN MEDICINE & BIOLOGY SOCIETY (EMBC)(2021)
摘要
The recent COVID-19 pandemic has further highlighted the need for improving tele-rehabilitation systems. One of the common methods is to use wearable sensors for monitoring patients and intelligent algorithms for accurate and objective assessments. An important part of this work is to develop an efficient evaluation algorithm that provides a high-precision activity recognition rate. In this paper, we have investigated sixteen state-of-the-art time-series deep learning algorithms with four different architectures: eight convolutional neural networks configurations, six recurrent neural networks, a combination of the two and finally a wavelet-based neural network. Additionally, data from different sensors' combinations and placements as well as different pre-processing algorithms were explored to determine the optimal configuration for achieving the best performance. Our results show that the XceptionTime CNN architecture is the best performing algorithm with normalised data. Moreover, we found out that sensor placement is the most important attribute to improve the accuracy of the system, applying the algorithm on data from sensors placed on the waist achieved a maximum of 42% accuracy while the sensors placed on the hand achieved 84%. Consequently, compared to current results on the same dataset for different classification categories, this approach improved the existing state of the art accuracy from 79% to 84%, and from 80% to 90% respectively.
更多查看译文
关键词
Deep learning, Tele-rehabilitation, Wearable sensors, Time-series, Stroke
AI 理解论文
溯源树
样例
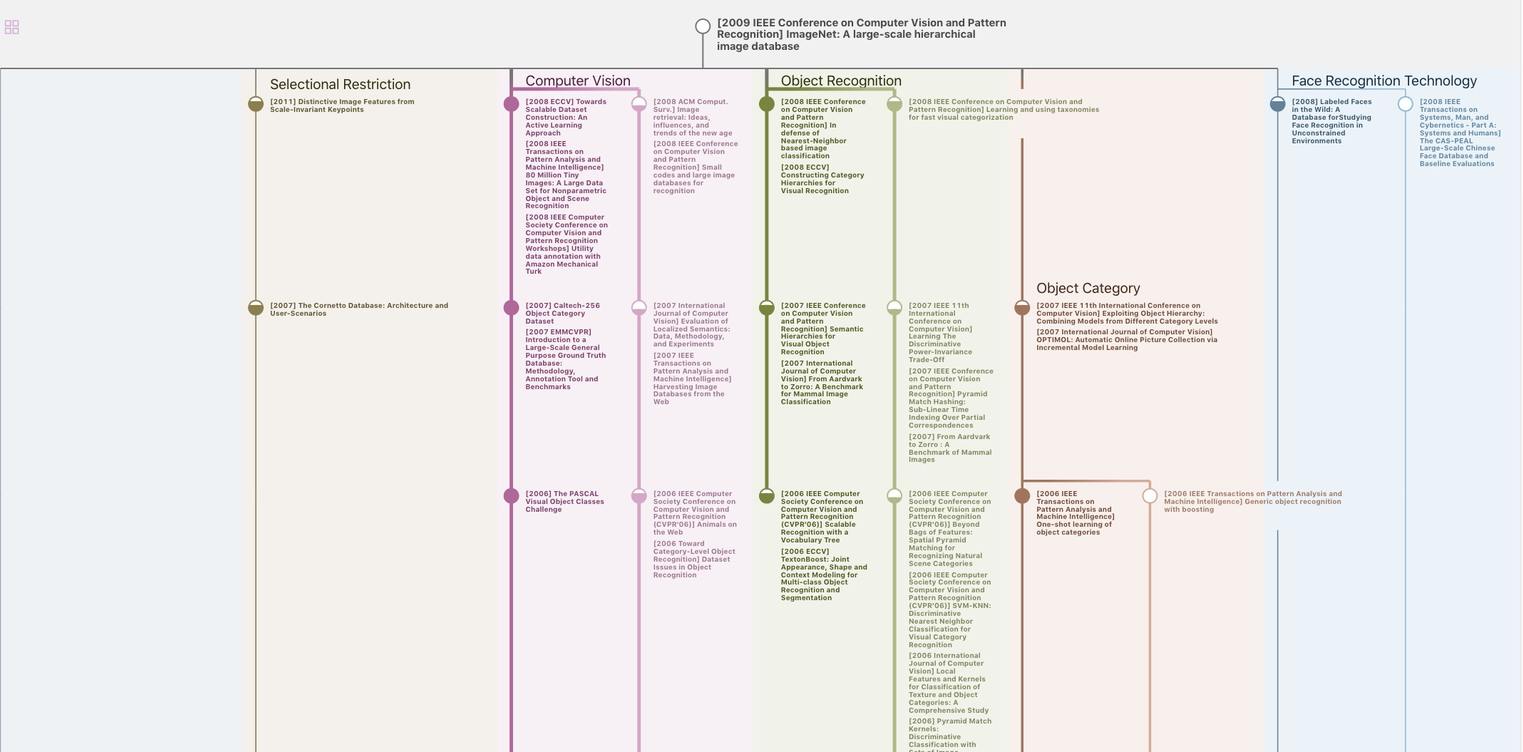
生成溯源树,研究论文发展脉络
Chat Paper
正在生成论文摘要