Enhanced Critical Congenital Cardiac Disease Screening by Combining Interpretable Machine Learning Algorithms
2021 43RD ANNUAL INTERNATIONAL CONFERENCE OF THE IEEE ENGINEERING IN MEDICINE & BIOLOGY SOCIETY (EMBC)(2021)
摘要
Critical Congenital Heart Disease (CCHD) screening that only uses oxygen saturation (SpO2), measured by pulse oximetry, fails to detect an estimated 900 US newborns annually. The addition of other pulse oximetry features such as perfusion index (PIx), heart rate, pulse delay and photoplethysmography characteristics may improve detection of CCHD, especially those with systemic blood flow obstruction such as Coarctation of the Aorta (CoA). To comprehensively study the most relevant features associated with CCHD, we investigated interpretable machine learning (ML) algorithms by using Recursive Feature Elimination (RFE) to identify an optimal subset of features. We then incorporated the trained ML models into the current SpO2-alone screening algorithm. Our proposed enhanced CCHD screening system, which adds the ML model, improved sensitivity by approximately 10 percentage points compared to the current standard SpO2-alone method with minimal to no impact on specificity.
更多查看译文
关键词
interpretable machine learning algorithms,machine learning,screening
AI 理解论文
溯源树
样例
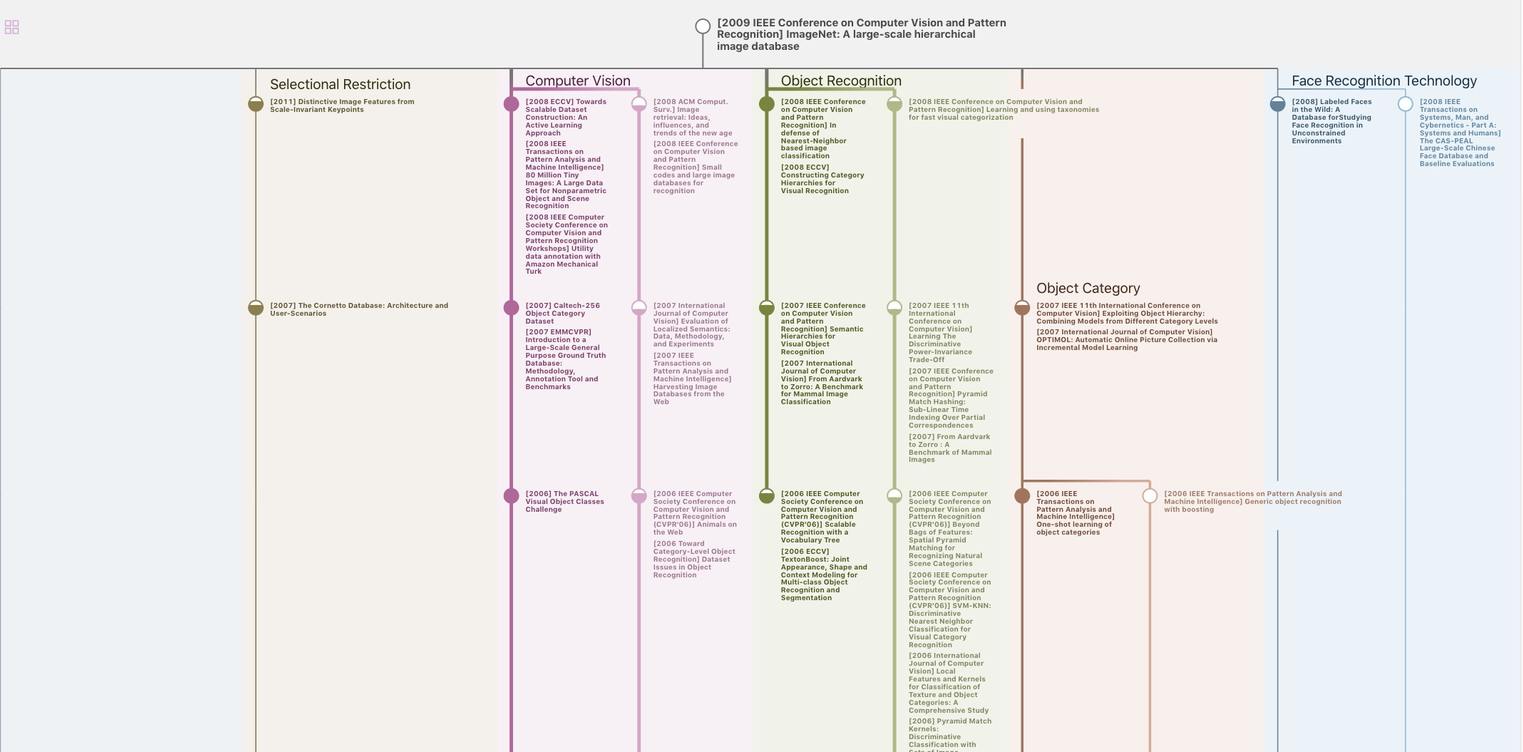
生成溯源树,研究论文发展脉络
Chat Paper
正在生成论文摘要