Classification of Epileptic Seizure From EEG Signal Based on Hilbert Vibration Decomposition and Deep Learning
2021 43RD ANNUAL INTERNATIONAL CONFERENCE OF THE IEEE ENGINEERING IN MEDICINE & BIOLOGY SOCIETY (EMBC)(2021)
摘要
a convolution neural network (CNN) architecture has been designed to classify epileptic seizures based on two-dimensional (2D) images constructed from decomposed mono-components of electroencephalogram (EEG) signals. For the decomposition of EEG, Hilbert vibration decomposition (HVD) has been employed. In this work, four brain rhythms - delta, theta, alpha, and beta have been utilized to obtain the monocomponents. Certainly, the data-driven CNN model is most efficient for 2D image processing and recognition. Therefore, 2D images have been generated from one-dimensional (1D) decomposed mono-components by employing continuous wavelet transform (CWT). Next, simultaneous multiple input images in parallel have been directly fed into the CNN pipeline for feature extraction and classification. For evaluation, the EEG dataset provided by the Bonn University has been taken into consideration. Further, a 5-fold cross-validation technique has been applied to obtain generalized and robust classification performance. The average classification accuracy, sensitivity, and specificity reached up to 98.6%, 97.2%, and 100% respectively. The results show that the proposed idea is very much efficient in seizure classification. The proposed idea resourcefully combines the advantages of HVD and CNN to classify epileptic seizures from EEG signal.
更多查看译文
关键词
hilbert vibration decomposition,epileptic seizure,eeg signal,classification,deep learning
AI 理解论文
溯源树
样例
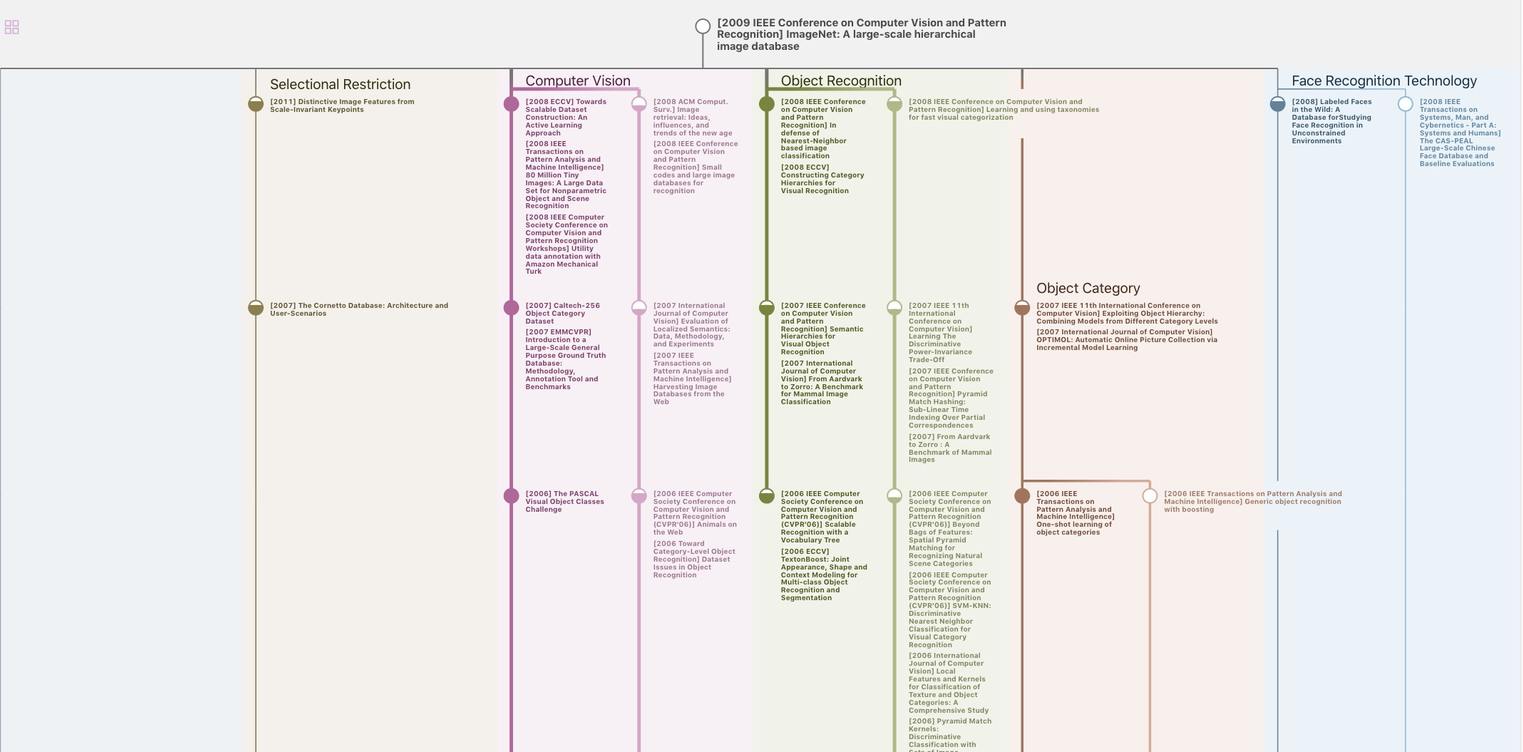
生成溯源树,研究论文发展脉络
Chat Paper
正在生成论文摘要