p Radiomics features based on automatic segmented MRI images: Prognostic biomarkers for triple-negative breast cancer treated with neoadjuvant chemotherapy
EUROPEAN JOURNAL OF RADIOLOGY(2022)
摘要
Purpose: To establish radiomics prediction models based on automatic segmented magnetic resonance imaging (MRI) for predicting the systemic recurrence of triple-negative breast cancer (TNBC) in patients after neoadjuvant chemotherapy (NAC). Materials and methods: A total of 147 patients with TNBC who underwent NAC between January 2009 and December 2018 were enrolled in this study. Clinicopathologic data were collected, and the differences between the recurrent and nonrecurrent patients were analyzed by univariate and multivariate analyses. Patients were randomly divided into training and testing sets. The training set consisted of 104 patients (recurrence: 22, nonrecurrence: 82), and the testing set consisted of 43 patients (recurrence: 9, nonrecurrence: 34). To establish the radiomics prediction model, we used a deep learning segmentation model to automatically segment tumor areas on dynamic contrast-enhanced-MRI images of preand post-NAC magnetic resonance examinations. Radiomics features were then extracted from the tumor areas. Three MRI radiomics models were developed in the training set: a radiomics model based on pre-NAC MRI features (model 1), a radiomics model based on postNAC MRI features (model 2), and a radiomics model based on both preand post-NAC MRI features (model 3). A clinical model for predicting systemic recurrence was built in the training set using independent clinical prediction factors. Receiver operating characteristic curve analysis was used to evaluate the performance of the radiomics and clinical models. Results: The clinical model yielded areas under the curve (AUCs) of 0.747 in the training set and 0.737 in the testing set in terms of predicting systemic recurrence. Models 1, 2, and 3 yielded AUCs of 0.879, 0.91, and 0.963 in the training set and 0.814, 0.802, and 0.933 in the testing set, respectively, in terms of predicting systemic recurrence. All of the radiomics models had achieved higher AUCs than the clinical model in the testing set. DeLong test was used to compare the AUCs between the models and indicated that the predictive performance of model 3 was better than the clinical model, and the difference was statistically significant (p < 0.05). Conclusion: The radiomics models built based on the combination of preand post-NAC MRI features showed good performance in predicting whether patients with TNBC will have systemic recurrence within 3 years postNAC. This can help us non-invasively identify which patients are at high risk of recurrence post-NAC, so that we can strengthen follow-up and treatment of these patients. Then the prognosis of these patients might be improved.
更多查看译文
关键词
Triple-negative breast cancer, Radiomics, Deep learning, DCE-MRI, Recurrence, Neoadjuvant chemotherapy
AI 理解论文
溯源树
样例
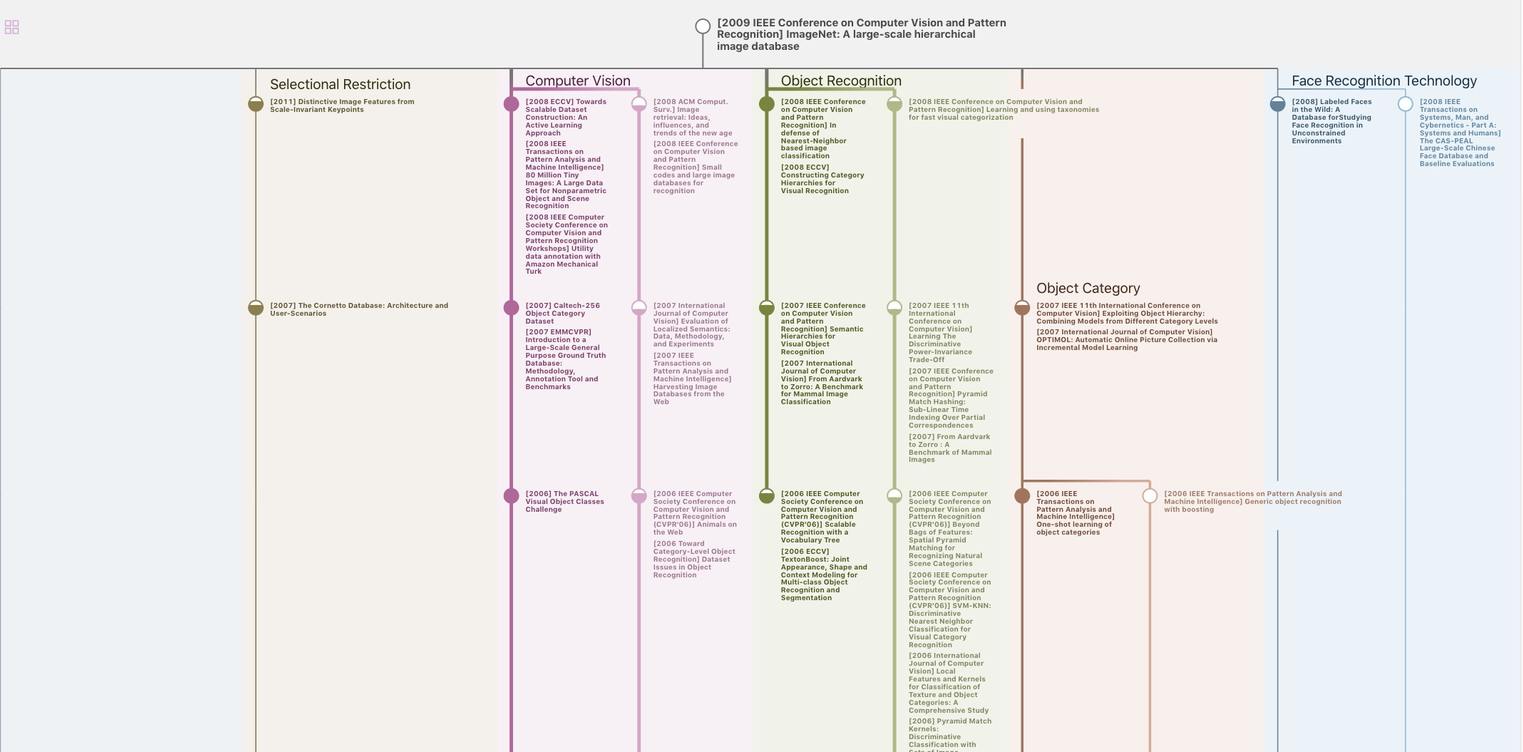
生成溯源树,研究论文发展脉络
Chat Paper
正在生成论文摘要