Adaptive Diffusion Pairwise Fused Lasso LMS Algorithm Over Networks
IEEE transactions on neural networks and learning systems(2023)
摘要
The topic of identification for sparse vector in a distributed way has triggered great interest in the area of adaptive filtering. Grouping components in the sparse vector has been validated to be an efficient way for enhancing identification performance for sparse parameter. The technique of pairwise fused lasso, which can promote similarity between each possible pair of nonnegligible components in the sparse vector, does not require that the nonnegligible components have to be distributed in one or multiple clusters. In other words, the nonnegligible components may be randomly scattered in the unknown sparse vector. In this article, based on the technique of pairwise fused lasso, we propose the novel pairwise fused lasso diffusion least mean-square (PFL-DLMS) algorithm, to identify sparse vector. The objective function we construct consists of three terms, i.e., the mean-square error (MSE) term, the regularizing term promoting the sparsity of all components, and the regularizing term promoting the sparsity of difference between each pair of components in the unknown sparse vector. After investigating mean stability condition of mean-square behavior in theoretical analysis, we propose the strategy of variable regularizing coefficients to overcome the difficulty that the optimal regularizing coefficients are usually unknown. Finally, numerical experiments are conducted to verify the effectiveness of the PFL-DLMS algorithm in identifying and tracking sparse parameter vector.
更多查看译文
关键词
Estimation,Clustering algorithms,Linear programming,Sensors,Mean square error methods,Learning systems,Adaptive systems,Diffusion least mean square (LMS),distributed estimation,mean-square deviation,pairwise fused lasso,sparse parameter
AI 理解论文
溯源树
样例
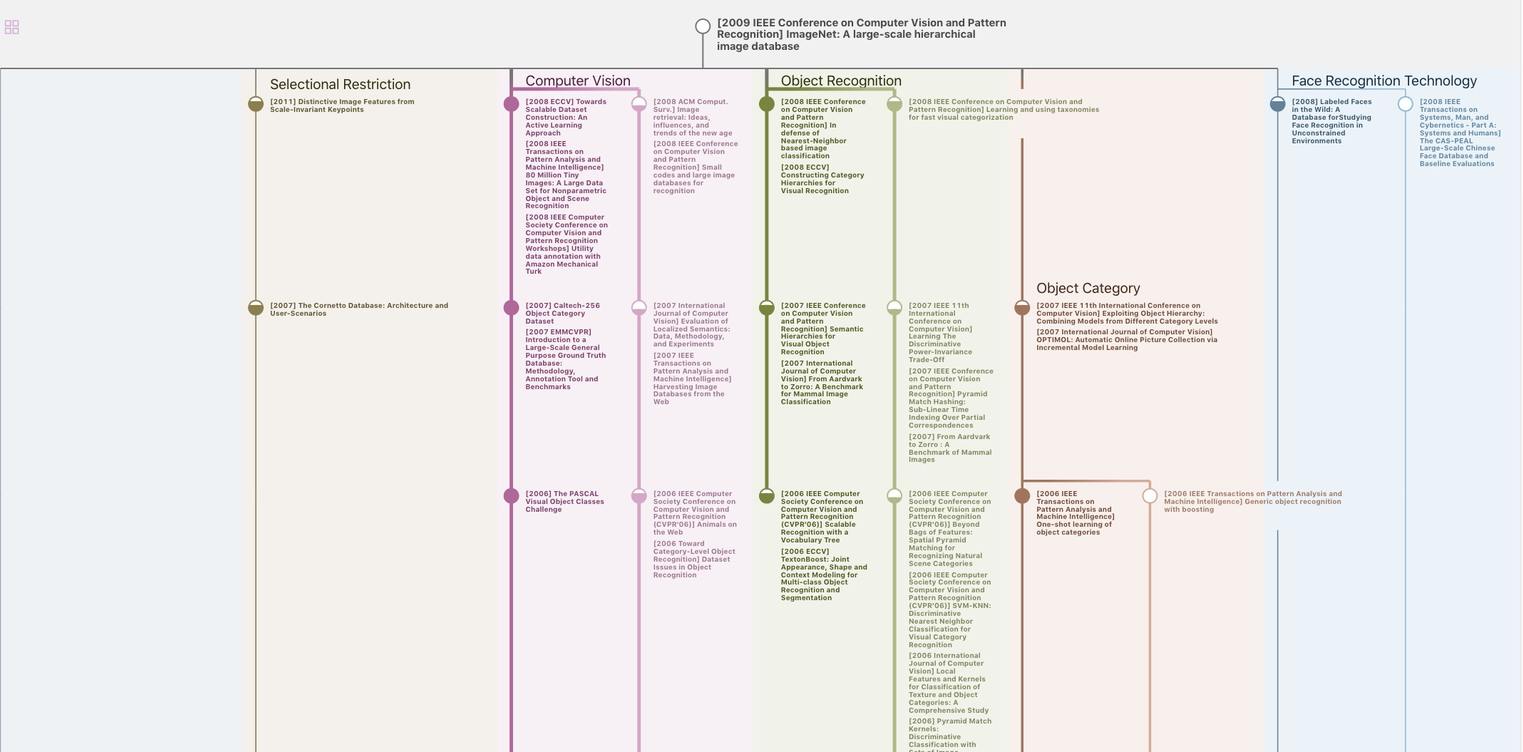
生成溯源树,研究论文发展脉络
Chat Paper
正在生成论文摘要