A Dataset Generation Tool for Deep learning-based Motion Planning in Complex Environments
2021 26TH IEEE INTERNATIONAL CONFERENCE ON EMERGING TECHNOLOGIES AND FACTORY AUTOMATION (ETFA)(2021)
摘要
Learning-based approaches for motion planning have gained much attention. These approaches show better performance in terms of computational efficiency as compared to the tradition approaches, such as sampling-based motion planning. One of the key challenges for learning-based approaches is to generate training datasets. This work-in-progress proposes a generalized dataset generation approach for motion planning that allows to generate simulation data for motion planning under geometric, kinodynamic and physics-based constraints. The generated dataset can easily be encoded in any required format for training. This tool will be used at the Institute of Industrial and Control Engineering (IOC-UPC) to test learning-based motion planning strategies for dual arm mobile manipulators under physics-based constraints. As a first step, in this study the developed approach is tested by generating datasets for mobile robots. The generated datasets are used to train motion planning networks, an approach for deep learning-based motion planning.
更多查看译文
关键词
Motion planning, Deep neural networks, dataset generation
AI 理解论文
溯源树
样例
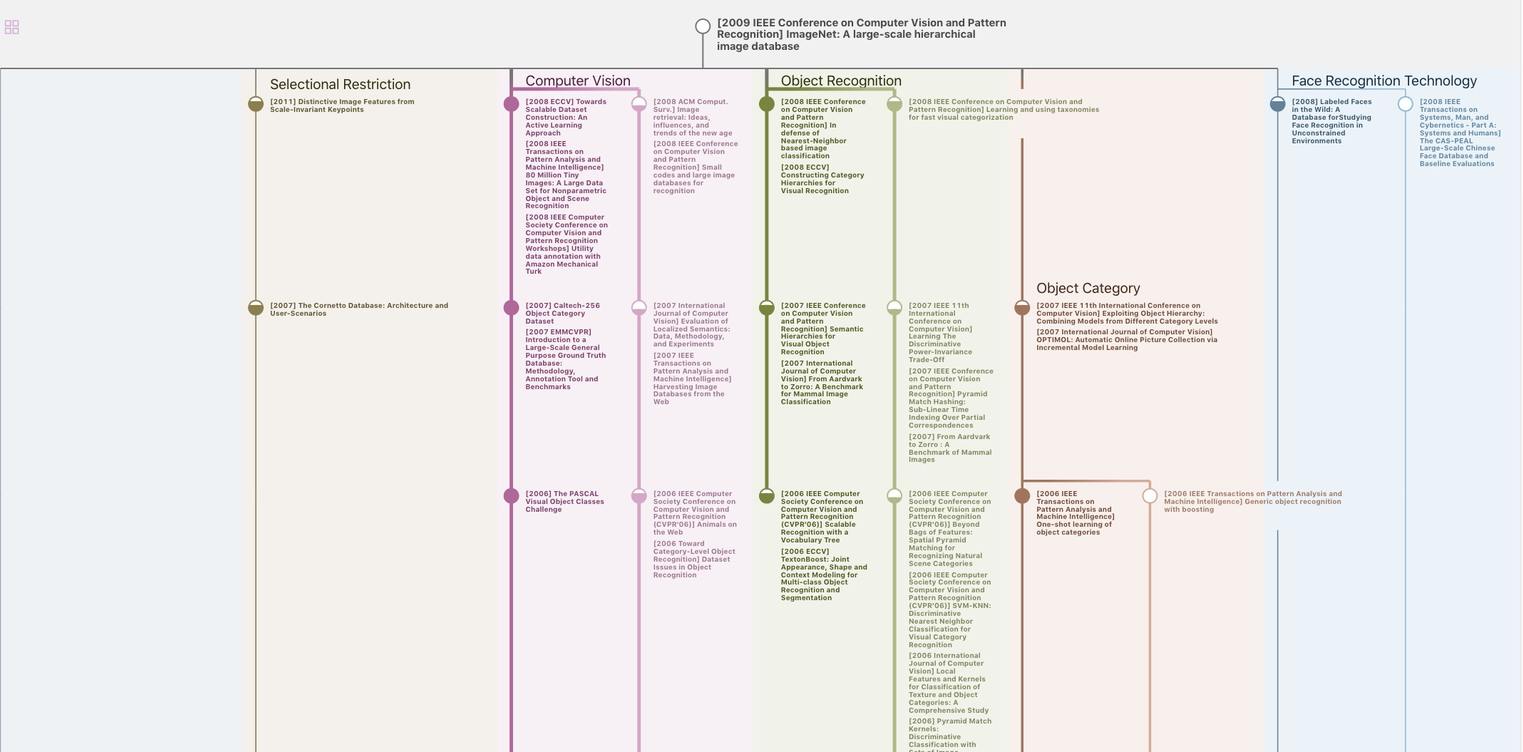
生成溯源树,研究论文发展脉络
Chat Paper
正在生成论文摘要