A Method To Map EEG Signals To Spoken Speech Using Gaussian Process Modeling
29TH EUROPEAN SIGNAL PROCESSING CONFERENCE (EUSIPCO 2021)(2021)
摘要
This work aimed to map electroencephalography (EEG) signals recorded during speech production to an intelligible speech. Experiments were designed to record EEG and spoken speech signals from normal participants. EEG features were processed with a Gaussian process regression method, and used to estimate multiple temporal amplitude envelopes of a spoken speech signal. The estimated envelopes were further applied to synthesize an intelligible speech signal by using a temporal envelope-based vocoder model. The performance of reconstructing the spoken speech signal was evaluated by the short-term objective intelligibility (STOI) index and the root mean square error (RMSE) between the reconstructed vocoded speech and the original spoken speech. Results showed a small RMSE between two sets of mel-frequency cepstral coefficients, and a STOI measurement up to 0.71. Both measures outperformed results from existing studies with similar tasks, indicating the potential in synthesizing an intelligible spoken speech with EEG signals in brain-computer interface based speech communication.
更多查看译文
关键词
electroencephalography, speech synthesis, brain computer interface, stochastic analysis
AI 理解论文
溯源树
样例
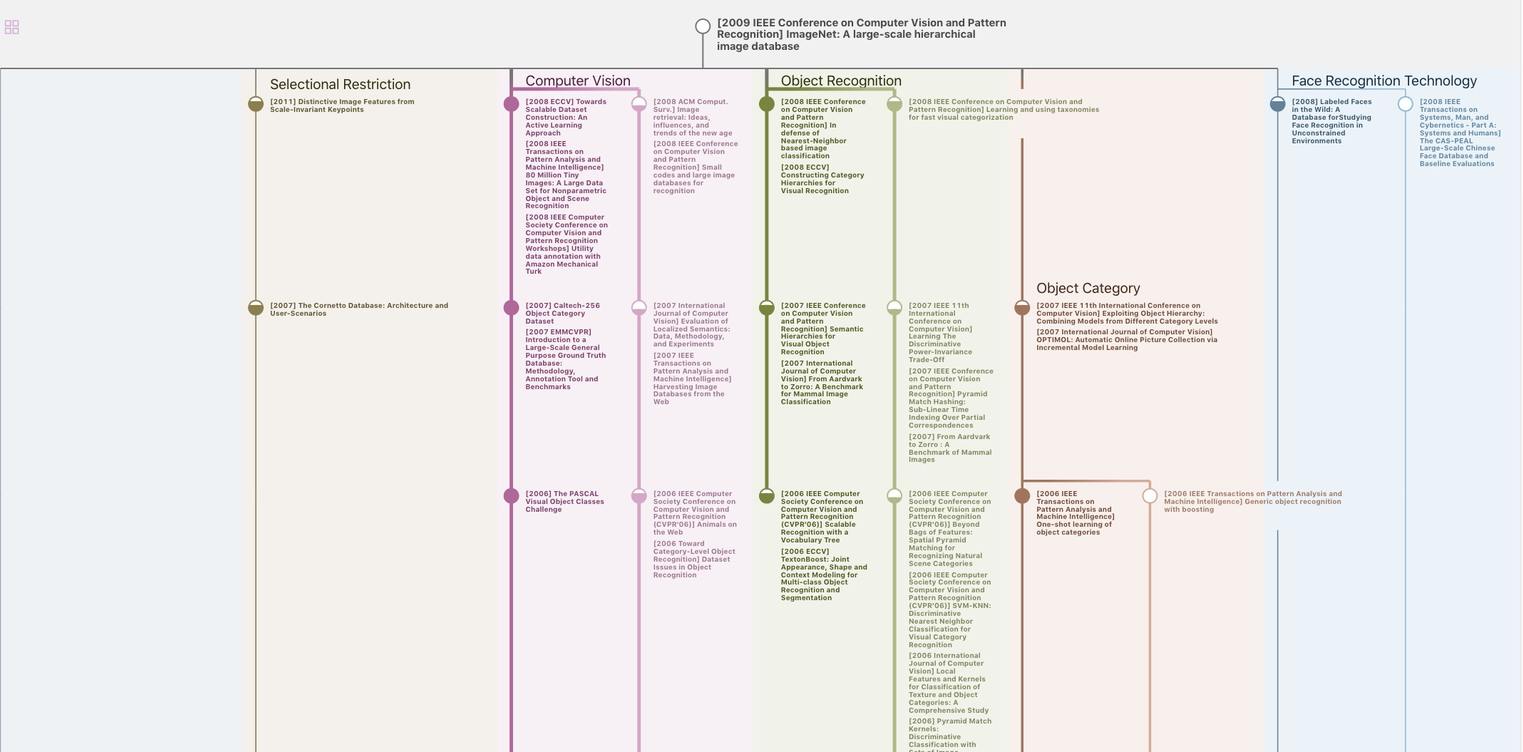
生成溯源树,研究论文发展脉络
Chat Paper
正在生成论文摘要