A fusion method based on class rotations for DNN-DoA Estimation on Spherical Microphone Array
29TH EUROPEAN SIGNAL PROCESSING CONFERENCE (EUSIPCO 2021)(2021)
摘要
Deep Neural Networks (DNN) has been used to estimate the Direction-of-Arrival (DoA) with spherical array under highly reverberant and noisy environments. In this paper, we propose a fusion technique for DNN as a solution to DNN-DOA estimations to obtain a joint decision and outperform the prediction's accuracy of a single network, which may also suggest a data augmentation technique. This proposed fusion consists of averaging the results of multiple networks with their spatially rotated categories to obtain a final approximation. The experiments carried in this work were performed using a 3D Convolutional Recurrent Neural Network (3DCRNN) structure for classification, which classes are determined by the t-design method as a pseudo-uniform spherical sampling. The performed simulations suggests an improvement over a single DNN performance, showing a reduction of the average angular error using 6 Networks can be achieved with 38.96% for highly reverberant and noisy environment.
更多查看译文
关键词
spherical microphone array, direction of arrival, spherical harmonics, convolutional recurrent neural network, late fusion
AI 理解论文
溯源树
样例
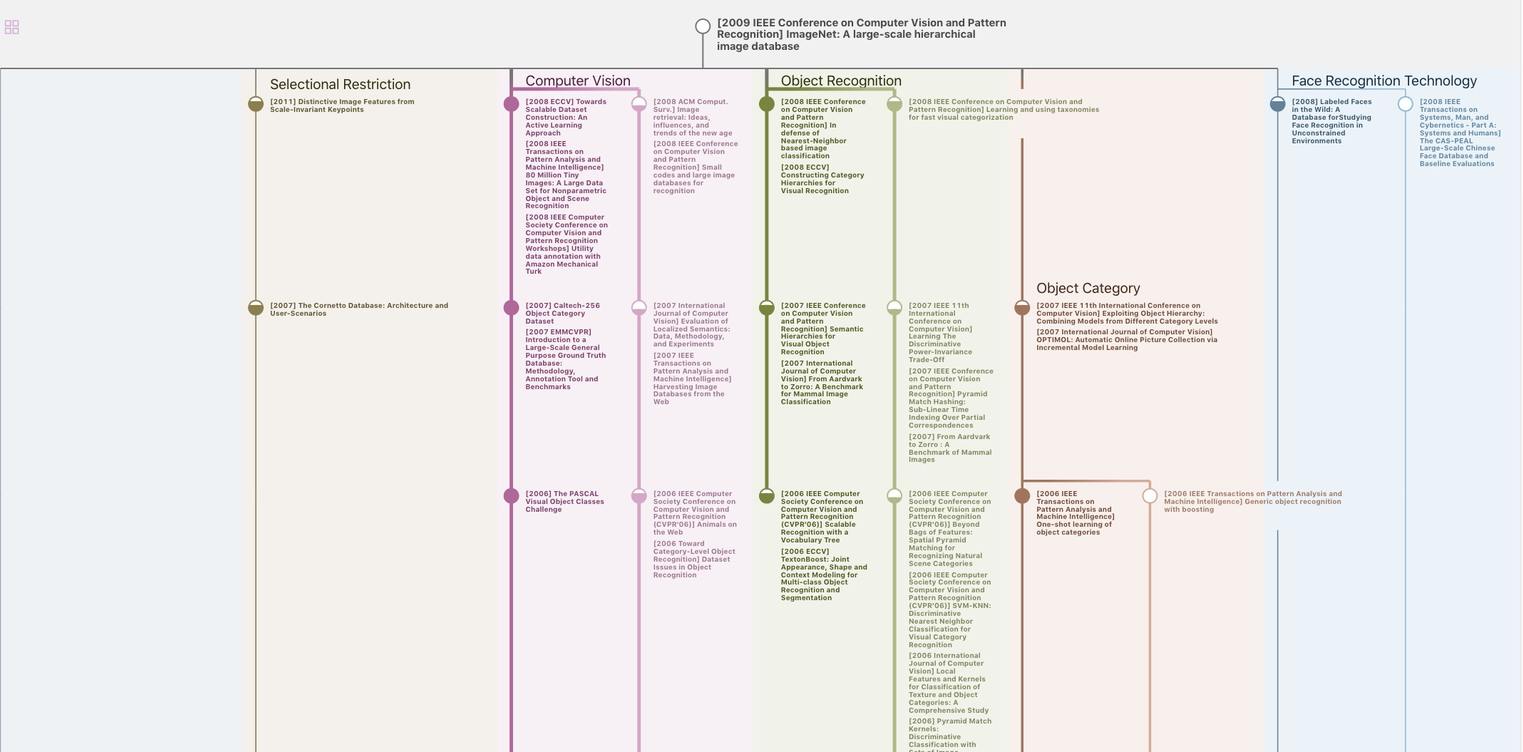
生成溯源树,研究论文发展脉络
Chat Paper
正在生成论文摘要