Exact Biobjective k-Sparse Nonnegative Least Squares
29TH EUROPEAN SIGNAL PROCESSING CONFERENCE (EUSIPCO 2021)(2021)
摘要
The k-sparse nonnegative least squares (NNLS) problem is a variant of the standard least squares problem, where the solution is constrained to be nonnegative and to have at most k nonzero entries. Several methods exist to tackle this NP-hard problem, including fast but approximate heuristics, and exact methods based on brute-force or branch-and-bound algorithms. Although intuitive, the k-sparse constraint is sometimes limited; the parameter k can be hard to tune, especially in the case of NNLS with multiple right-hand sides (MNNLS) where the relevant k could differ between columns. In this work, we propose a novel biobjective formulation of the k-sparse nonnegative least squares problem. We present an extension of Arborescent, a branch-and-bound algorithm for exact k-sparse NNLS, that computes the whole Pareto front (that is, the set of optimal solutions for all values of k) instead of only the k-sparse solution, for virtually the same computing cost. We also present a method for MNNLS that enforces a matrix-wise sparsity constraint, by first computing the Pareto front for each column and then selecting one solution per column to build a globally optimal solution matrix. We show the advantages of the proposed approach for the unmixing of hyperspectral images.
更多查看译文
关键词
sparse approximation, l(0) constraint, biobjective optimization, nonnegative least squares
AI 理解论文
溯源树
样例
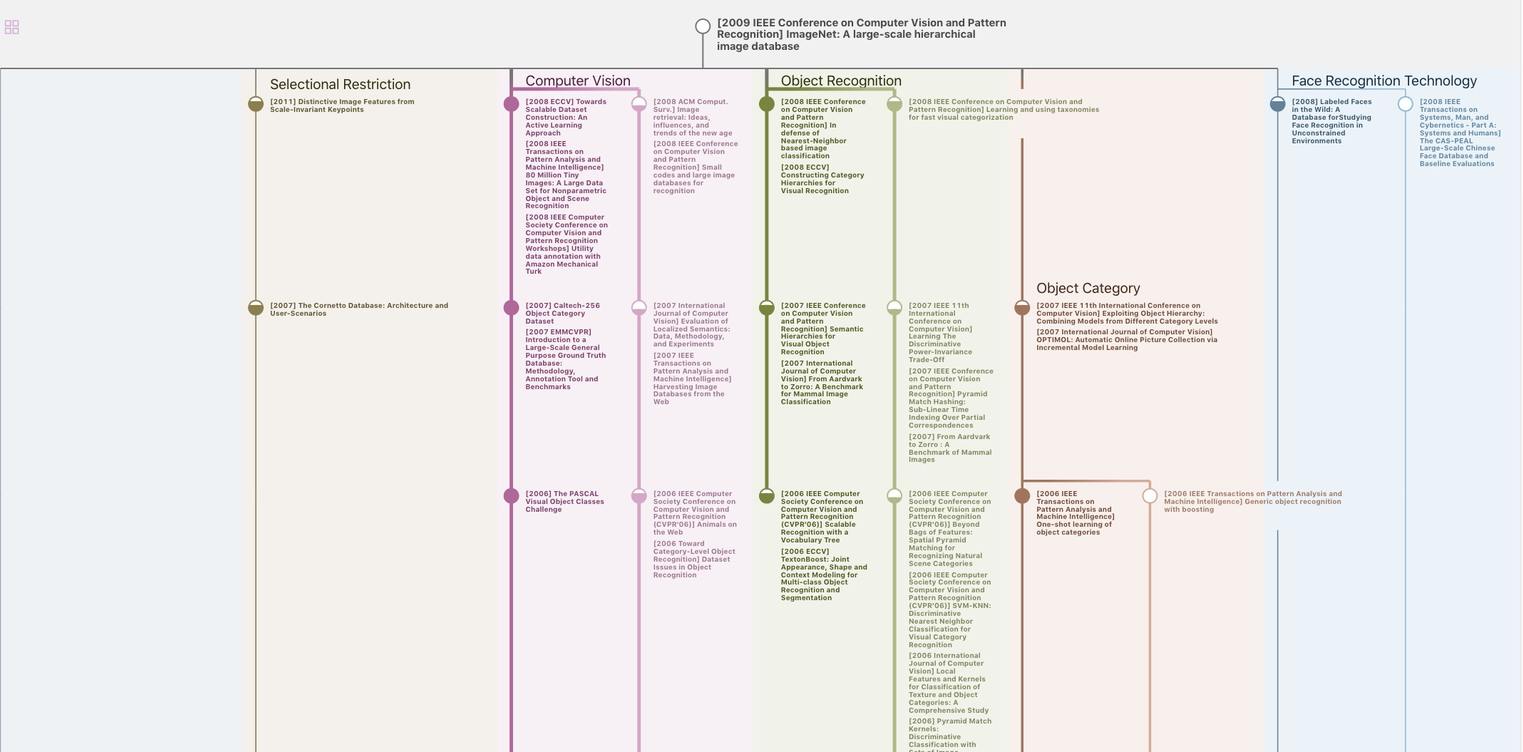
生成溯源树,研究论文发展脉络
Chat Paper
正在生成论文摘要