Improved L1-Tucker via L1-Fitting
29TH EUROPEAN SIGNAL PROCESSING CONFERENCE (EUSIPCO 2021)(2021)
摘要
Tucker decomposition is the generalization of Principal Component Analysis to high-order tensors. The L2-norm-based formulation of standard Tucker suffers from severe sensitivity to outliers. L1-norm-based Tucker (L1-Tucker) has been proposed as an outlier-resistant alternative with documented success in an array of applications. L1-HOSVD is a straightforward solver for L1-Tucker that computes each basis by conducting L1-PCA on the matrix that is derived by flattening the data tensor across the corresponding mode. In this paper, L1-HOSVD is further enhanced via an additional L1-fitting step. The proposed method combines the outlier-resistance of L1-HOSVD with that of L1-fitting and returns jointly-computed bases of enhanced robustness, as corroborated by our numerical studies on synthetic and real-world datasets.
更多查看译文
关键词
Tensors, Tucker, L1-norm, outliers, robustness
AI 理解论文
溯源树
样例
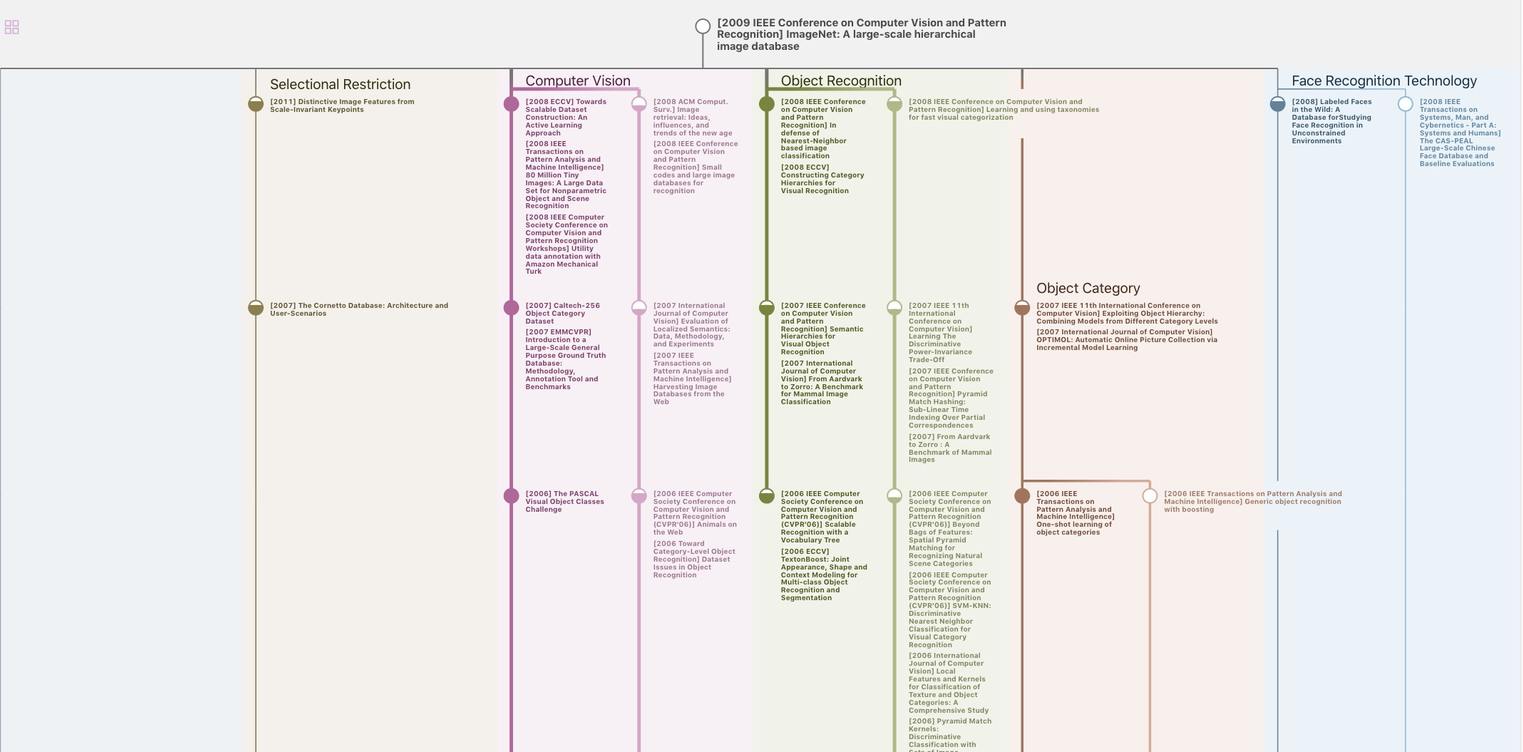
生成溯源树,研究论文发展脉络
Chat Paper
正在生成论文摘要