Implicit vs. Explicit Style Transfer? A Comparison of GAN Architectures for Continuous Path Keyboard Input Modeling
29TH EUROPEAN SIGNAL PROCESSING CONFERENCE (EUSIPCO 2021)(2021)
摘要
The success of continuous path keyboard input as an alternative text input modality requires high-quality training data to inform the underlying recognition model. In [1], we have adopted generative adversarial networks (GANs) to augment the training corpus with synthetic user-realistic paths. GAN-driven synthesis makes it possible to emulate the acquisition of enough paths from enough users to learn a model sufficiently robust across a large population. The present work studies the influence of different GAN architectures on path quality and diversity. Experiments show that explicit content/ style disentanglement resulting from separate style encoding has only a limited impact on end user perception. On the other hand, implicit and explicit style transfer paradigms are complementary in the kind of user-realistic artifacts they generate. Leveraging multiple GAN strategies thus injects more robustness into the model through broader coverage of user idiosyncrasies across a wide lexical range.
更多查看译文
关键词
Continuous path recognition, generative adversarial networks, style transfer, embedded devices
AI 理解论文
溯源树
样例
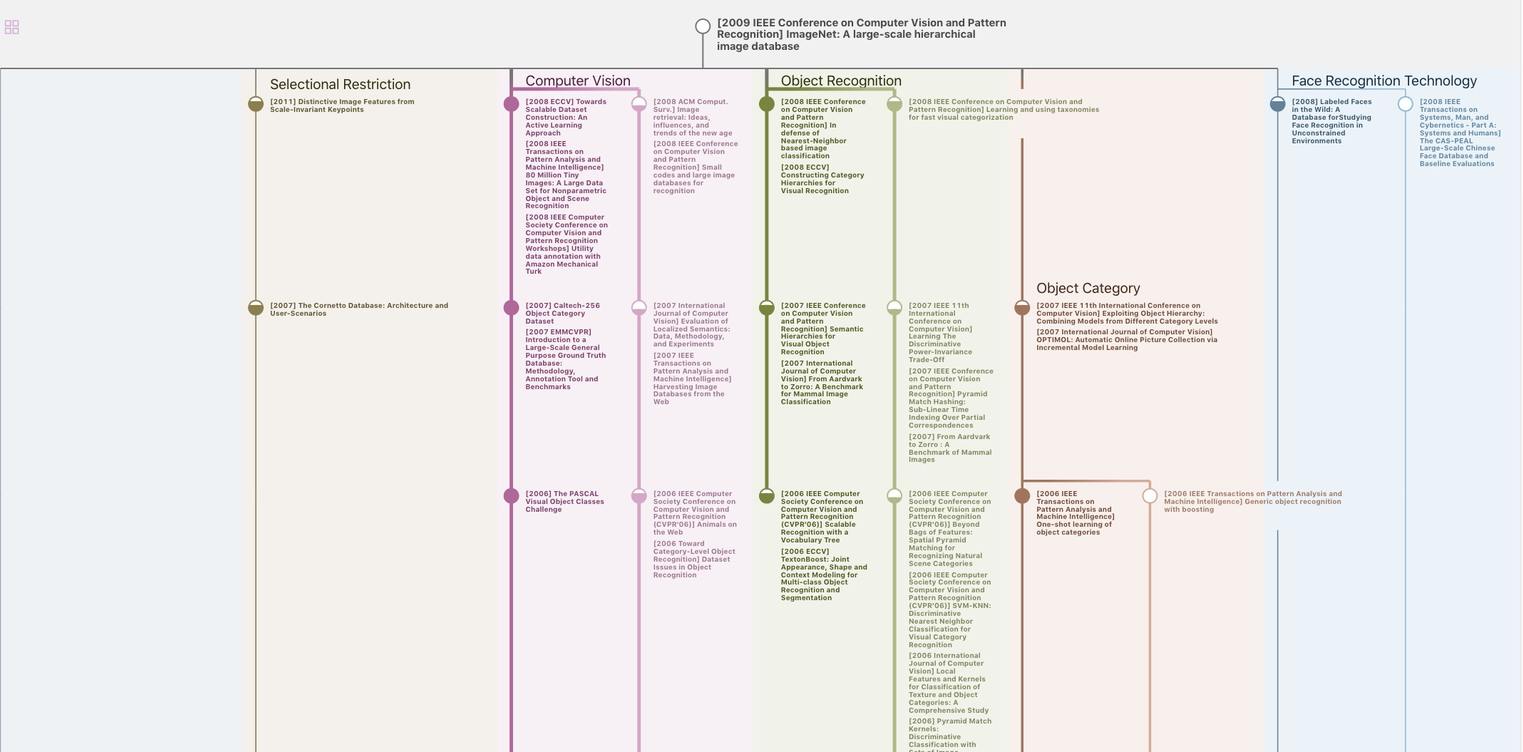
生成溯源树,研究论文发展脉络
Chat Paper
正在生成论文摘要