Gradient Estimation in Model-Based Reinforcement Learning - A Study on Linear Quadratic Environments.
BRACIS(2021)
摘要
Stochastic Value Gradient (SVG) methods underlie many recent achievements of model-based Reinforcement Learning agents in continuous state-action spaces. Despite their practical significance, many algorithm design choices still lack rigorous theoretical or empirical justification. In this work, we analyze one such design choice: the gradient estimator formula. We conduct our analysis on randomized Linear Quadratic Gaussian environments, allowing us to empirically assess gradient estimation quality relative to the actual SVG. Our results justify a widely used gradient estimator by showing it induces a favorable bias-variance tradeoff, which could explain the lower sample complexity of recent SVG methods.
更多查看译文
关键词
Reinforcement learning, Model-based, Machine learning
AI 理解论文
溯源树
样例
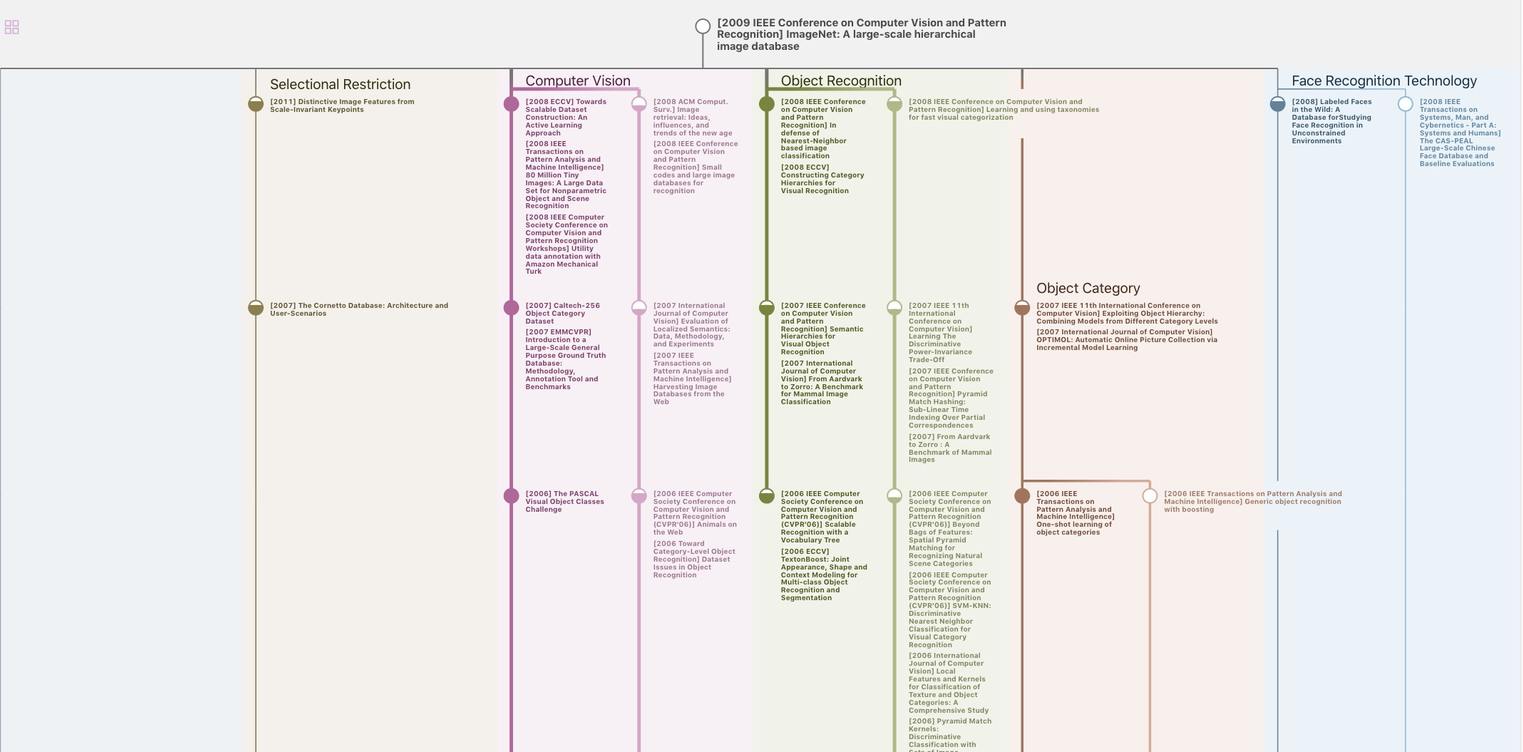
生成溯源树,研究论文发展脉络
Chat Paper
正在生成论文摘要