A Reconfigurable Accelerator Design for Quantized Depthwise Separable Convolutions.
ISOCC(2021)
摘要
Convolutional Neural Networks (CNNs) are widely applied in various computer version applications such as object recognition and image classification. With large amount of Multiply Accumulate Operations (MACs) in CNN computations, it is a trend to process the data locally at edge devices to avoid significant data movement. MobileNets were proposed as a light-weight neural network for mobile and embedded devices by using depthwise separable convolutions. Moreover, to further reduce commutation efforts, quantization can be appropriately applied to MobileNets without significant accuracy loss. To provide an efficient computation platform for quantized MobileNets, in this paper we propose a novel performance-aware reconfigurable accelerator design which prefect fit the depthwise separable convolutions. Experimental results show that we can achieve 16% area reduction and 1.07x speedup compared to previous MobileNets accelerator design.
更多查看译文
关键词
AI accelerator,Reconfigurable Structure,Mobilenets
AI 理解论文
溯源树
样例
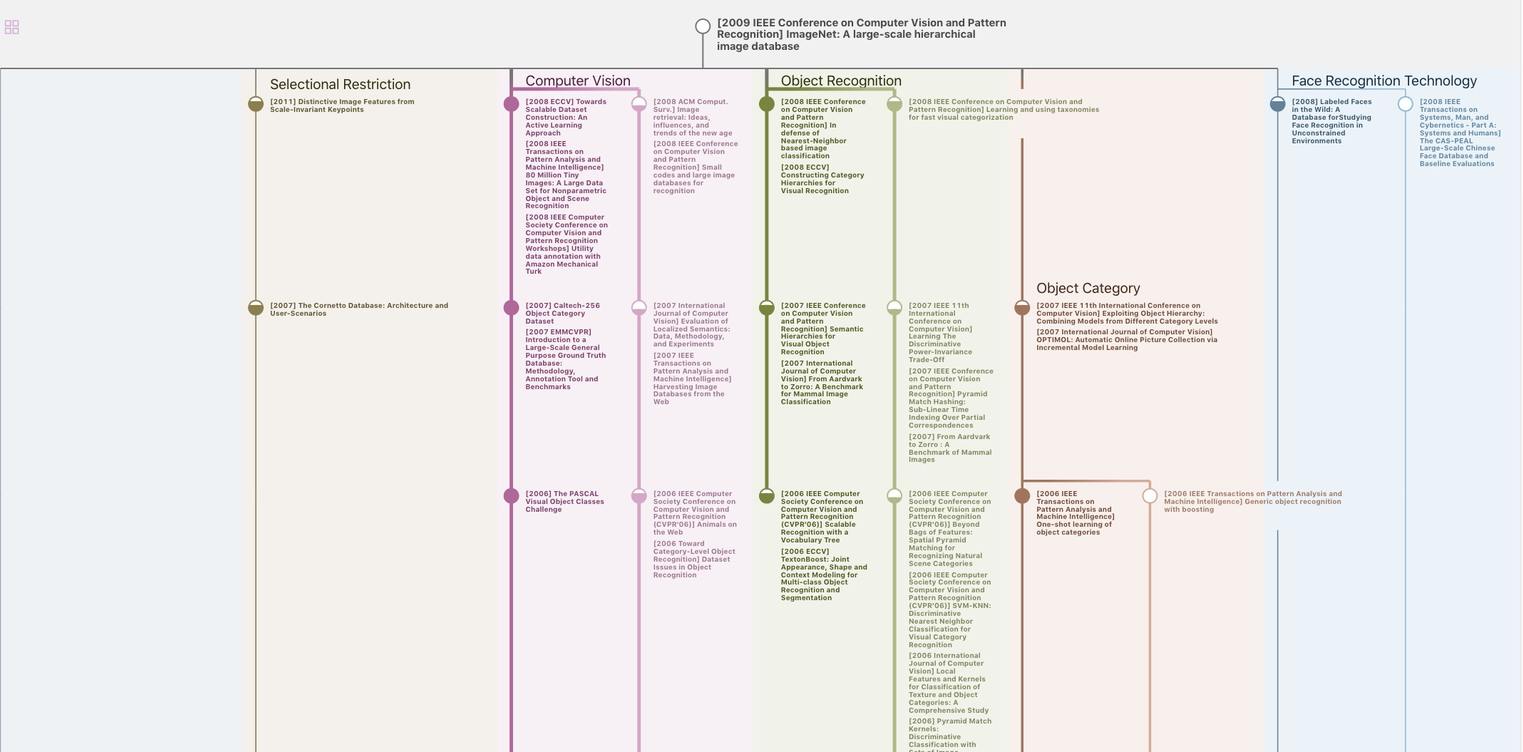
生成溯源树,研究论文发展脉络
Chat Paper
正在生成论文摘要