Sequential Recommender System of Educational Contents with End-to-End Title Feature Extraction for Reducing Utility Gap.
GCCE(2021)
摘要
Conventional sequential recommender system uses content features extracted by a hand-craft method, and a pre-trained deep learning method. This causes the utility gap between content features and the purpose of sequential recommendations. To reduce the utility gap, we propose a sequential recommender system with end-to-end title feature extraction. Specifically, we develop a convolutional text feature extraction method for title features. Because we can optimize text features for the sequential recommendation in the end-to-end manner, we can reduce the utility gap. Experimental results for real educational contents and teachers’ operating histories on Forestanet show the effectiveness of our proposed method.
更多查看译文
关键词
educational contents,extraction,title,end-to-end
AI 理解论文
溯源树
样例
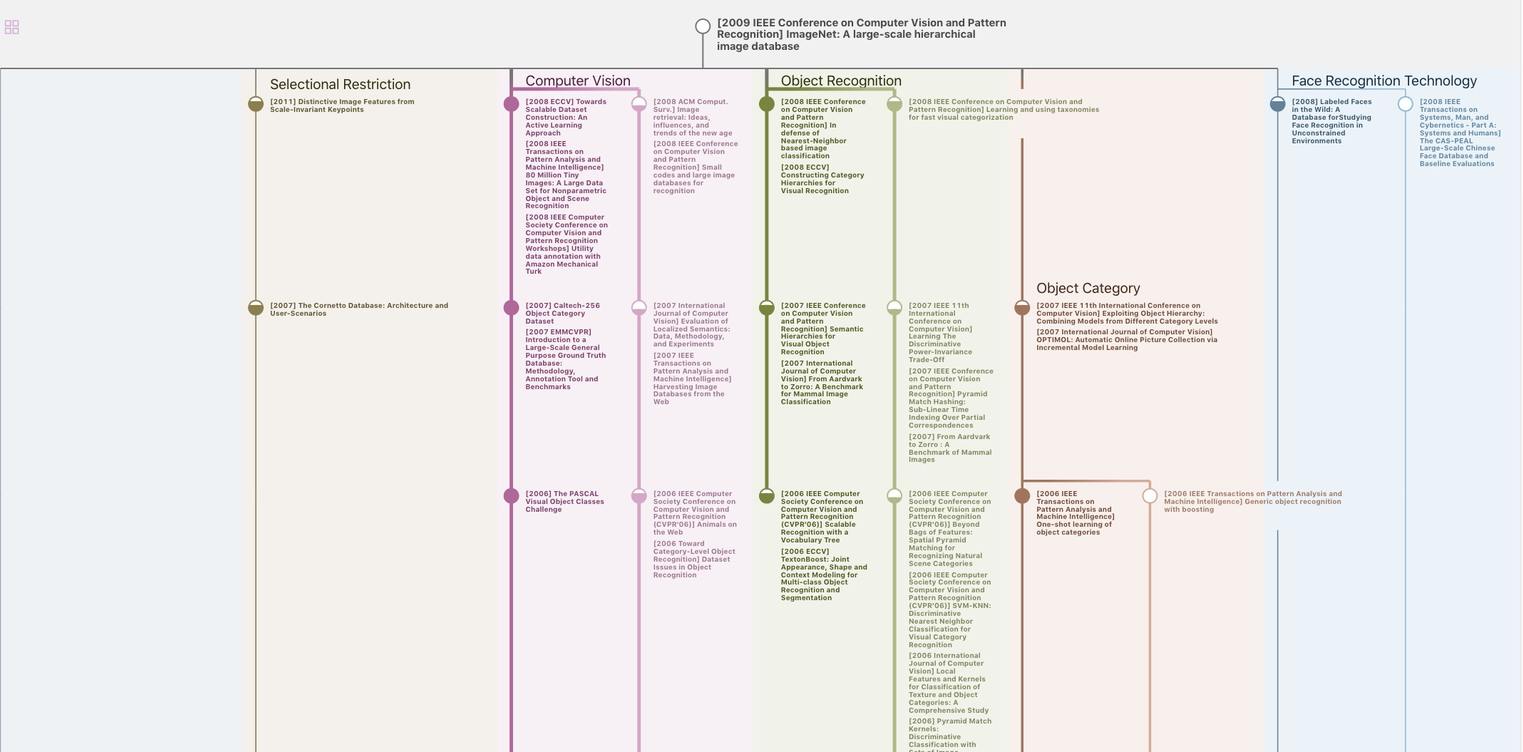
生成溯源树,研究论文发展脉络
Chat Paper
正在生成论文摘要