Language-guided Multi-Modal Fusion for Video Action Recognition.
IEEE International Conference on Computer Vision(2021)
摘要
A recent study [30] has found that training a multi-modal network often produces a network that has not learned the proper parameters for video action recognition. These multi-modal network models perform normally during training but fall short to its single modality counterpart when testing. The main cause for this performance drop could be two-fold. First, conventional methods use a poor fusion mechanism, where each modality is trained separately and then simply combine together (e.g., late feature fusion). Second, collecting videos is much more expensive than images. The insufficient video data can hardly provide support for training a multi-modal network that has a larger and more complex weight space. In this paper, we proposed the Language-guided Multi-Modal Fusion to address the above poor fusion problem. A sophisticatedly designed bi-modal video encoder is used to fuse audio and visual signal to generate a finer video representation. To ensure the over-fitting can be avoid, we use a language-guided contrastive learning to largely augment the video data to support the learning of multi-modal network. On a large-scale benchmark video dataset, the proposed method successfully elevates the accuracy of video action recognition.
更多查看译文
关键词
Language-guided multimodal fusion,video action recognition,multimodal network models,single modality counter-part,poor fusion mechanism,late feature fusion,insufficient video data,Language-guided MultiModal Fusion,poor fusion problem,sophisticatedly designed bi-modal video encoder,finer video representation,language-guided contrastive learning,large-scale benchmark video dataset
AI 理解论文
溯源树
样例
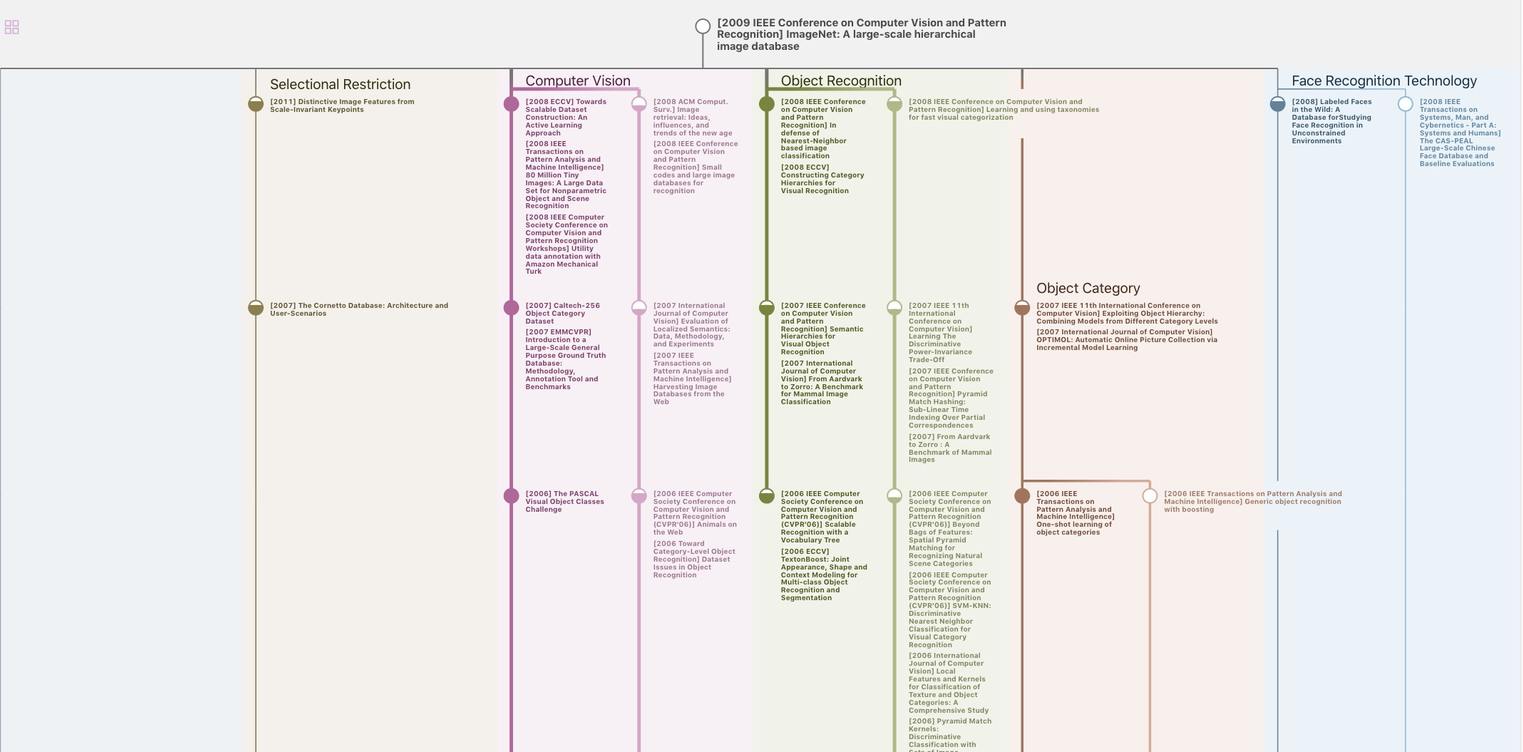
生成溯源树,研究论文发展脉络
Chat Paper
正在生成论文摘要