Bridging the Reality Gap for Pose Estimation Networks using Sensor-Based Domain Randomization
2021 IEEE/CVF INTERNATIONAL CONFERENCE ON COMPUTER VISION WORKSHOPS (ICCVW 2021)(2021)
摘要
Since the introduction of modern deep learning methods for object pose estimation, test accuracy and efficiency has increased significantly. For training, however, large amounts of annotated training data are required for good performance. While the use of synthetic training data prevents the need for manual annotation, there is currently a large performance gap between methods trained on real and synthetic data. This paper introduces a new method, which bridges this gap. Most methods trained on synthetic data use 2D images, as domain randomization in 2D is more developed. To obtain precise poses, many of these methods perform a final refinement using 3D data. Our method integrates the 3D data into the network to increase the accuracy of the pose estimation. To allow for domain randomization in 3D, a sensor-based data augmentation has been developed. Additionally, we introduce the SparseEdge feature, which uses a wider search space during point cloud propagation to avoid relying on specific features without increasing run-time. Experiments on three large pose estimation benchmarks show that the presented method outperforms previous methods trained on synthetic data and achieves comparable results to existing methods trained on real data.
更多查看译文
关键词
test accuracy,modern deep learning methods,sensor-based domain randomization,pose estimation networks,reality gap,estimation benchmarks,sensor-based data augmentation,precise poses,synthetic data,performance gap,manual annotation,synthetic training data,annotated training data
AI 理解论文
溯源树
样例
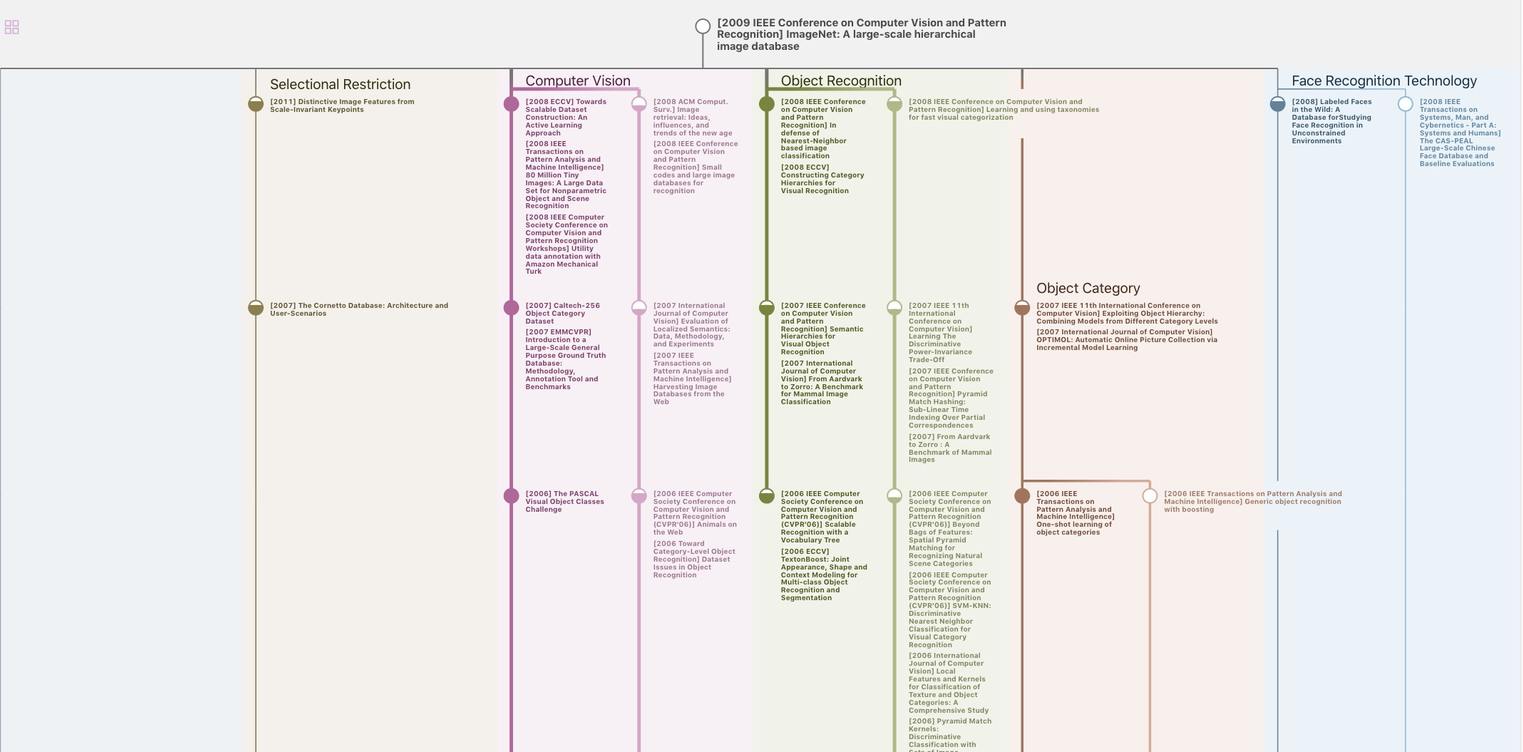
生成溯源树,研究论文发展脉络
Chat Paper
正在生成论文摘要