Reinforcement Learning with Model-Based Approaches for Dynamic Resource Allocation in a Tandem Queue
PERFORMANCE ENGINEERING AND STOCHASTIC MODELING(2021)
摘要
We consider three-tier network architecture modeled with two physical nodes in tandem where an autonomous agent controls the number of active resources on each node. We analyse the learning of auto-scaling strategies in order to optimise both performance and energy consumption of the whole system. We compare several model-based reinforcement learning with model-free Q-learning algorithm. The relevance of these algorithms is to faster update Q-value function with an additional planning phase allowed by approximated model of the dynamics of the environment. Secondly, we consider the same tandem queue scenario with MMPP (Markov modulated Poisson process) for arrivals. In this context, the arrival rate is varying over time and this information is hidden to the agent. Our goal is to assess the robustness of such model-based reinforcement learning algorithms in this particular scenario.
更多查看译文
关键词
Model-based reinforcement learning, Tandem queues, Energy saving, Cloud, QoS guarantee
AI 理解论文
溯源树
样例
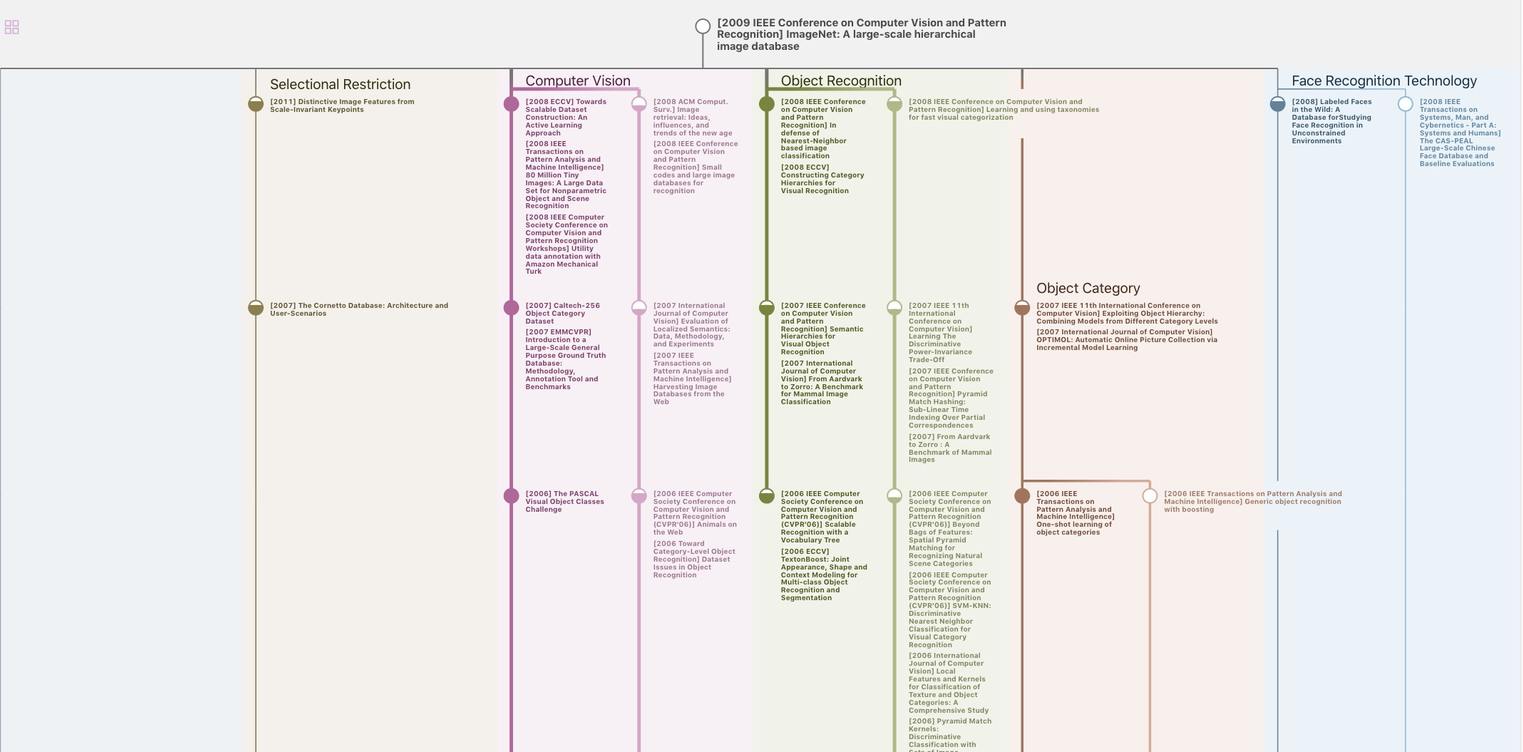
生成溯源树,研究论文发展脉络
Chat Paper
正在生成论文摘要