MULTIPLE - Multi-level User Preference Learning for List Recommendation.
WISE(2021)
摘要
On many streaming platforms, several individual items with certain common correlations are organized into lists, making list recommendation a distinctive and significant task. Multiple data including user-list interactions, user-item interactions and the list-item hierarchical structure are available for list recommendation. However, existing list recommendation models fail to conduct in-depth analyses of user preferences implied in the interactions and also fail to make use of the sequential information among interactions. In this paper, we propose a model named MULTIPLE, which recognizes the structural and compositional complexity of user preferences and explicitly learns user preferences from different perspectives, i.e., list-level, item-level and dual-level. In particular, MULTIPLE designs a gated and attentive sequence learning module to identify the drift of user preferences. By taking the list-item hierarchical structure as a bridge, MULTIPLE designs an attention network to distill essential items from the constituent items of lists and help learn the dual-level user preferences. We conduct extensive experiments on real-world datasets. The performance enhancement on recallAK and NDCGAK verifies the effectiveness of the model.
更多查看译文
关键词
Recommender system,List recommendation,Multi-level user preferences
AI 理解论文
溯源树
样例
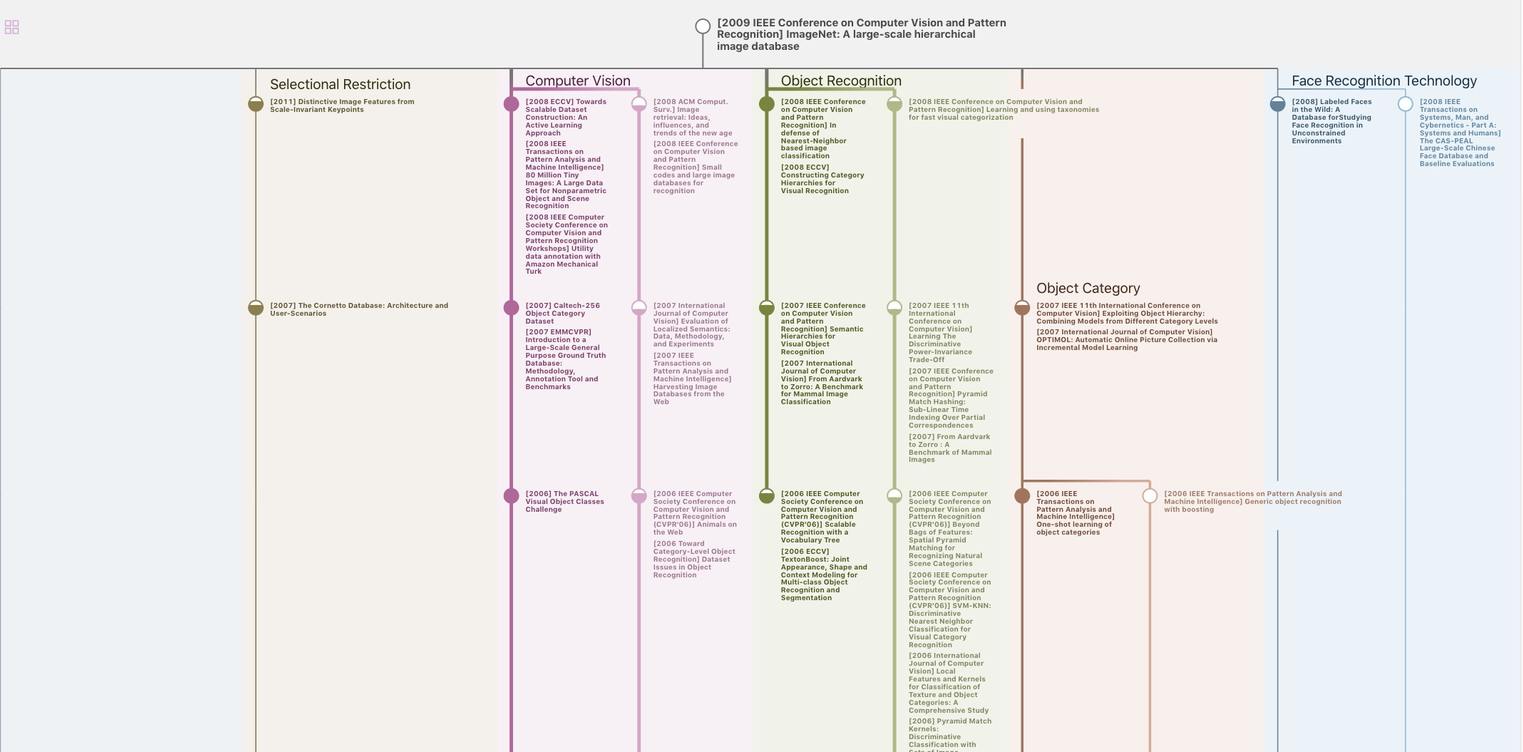
生成溯源树,研究论文发展脉络
Chat Paper
正在生成论文摘要