Anchoring-and-Adjustment to Improve the Quality of Significant Features.
WISE(2021)
摘要
There is an enormous demand for Explainable Artificial Intelligence to obtain human-understandable models. For example, advertisers are keen to understand what makes video ads successful. In our investigation, we have analysed heterogeneous visual, auditory, and textual content features from YouTube video ads. This paper proposes a two-stage anchoring-and-adjustment approach. In the first stage, we search for the optimum penalized value in the regularization path of Lasso that maximizes the number of Significant Features (SFs). After that, we improve the quality of SFs by dropping features with high Variance-Inflation-Factor (VIF) because high VIF often makes a spurious set of SFs. Experiments show that, compared to the one-stage approach without the adjustment stage, our proposed two-stage approach results in a smaller number of SFs but a higher ability to identify true features that appeal to ad viewers from human evaluation. Furthermore, our approach can identify much more SFs while maintaining similar prediction accuracy as what Lasso and Elastic-net can obtain.
更多查看译文
关键词
Explainable models,Content features,Anchoring-and-adjustment,Variance-inflation-factor,Significance test
AI 理解论文
溯源树
样例
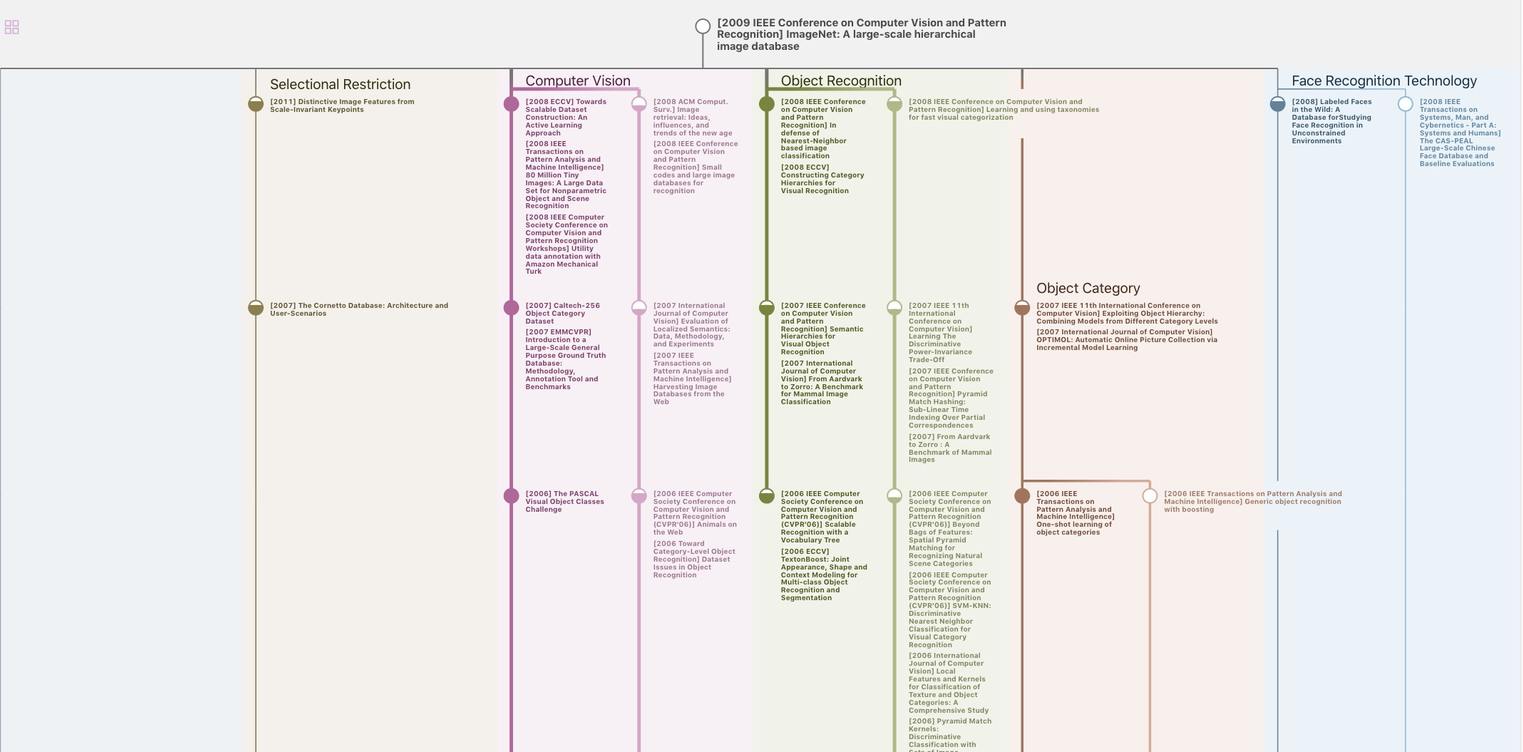
生成溯源树,研究论文发展脉络
Chat Paper
正在生成论文摘要